Data annotators are the unsung heroes of medicine’s artificial intelligence revolution
The artificial intelligence (A.I.) era is booming. More than twice as many papers mentioning A.I. were published in medical journals in 2018 than in 2017. The way machine and deep learning algorithms can contribute to diagnosing medical conditions or tailoring therapies have been explored in major studies (1). However, the medical community is concerned and has expressed doubts about the challenges A.I. poses to healthcare (2,3). While the potential of artificial narrow intelligence in assisting medical professionals in their daily work is exceptionally high, there is one aspect of the journey towards A.I. that often gets forgotten: the importance of data annotators.
Data annotators are medical professionals who undertake this time-consuming, rather monotonous task without the flare that usually encircles A.I. As algorithms improve through vast amounts of relevant data, without the annotators’ dedicated work, it is simply impossible to develop algorithms, and thus A.I. will not arise and will not be used in the healthcare setting (4).
The issue of data annotation is reflected well through a simple example: the challenge of recognizing cats on images. General rules humans create do not work in this context as having four legs, fur and two eyes are not features that are easy to explain to an algorithm that only detects pixels on the image with their individual colour and intensity, all expressed in numbers. Therefore, the way developers have been tackling this is by feeding the algorithm with a lot of images of cats and letting the algorithm find out its own rules for recognizing a cat.
This requires not only images of cats but annotated ones where a person has previously marked the area on the image that represents a cat. The same rule applies to data obtained in medicine. Medical images, medical records, photos and other scans all require annotation by a professional to make sure the algorithm learns the right rules and draws useful conclusions (5).
These medical professionals sit in a room and go through X-ray and CT scans; electrocardiogram (ECG) recording; medical records; pathology slides, or other sources of data, and count, label and draw lines to make sure the algorithm will understand the diagnosis, the details of a case, the exact cell types on a pathological slide, or the precise location of a medical issue on a scan. They spend countless hours doing this repetitive type of work while the real consequence of their efforts might only appear years later, if they have a chance to enjoy it themselves.
As medical data archives were obviously not created with future A.I. algorithms in mind, standardizing existing sampling processes is also a challenge. In pathology, for instance, the staining method, the age of the sample, the department where the sample was produced all matter when it comes to making a decision about a sample whether it can be annotated for algorithms.
As the community of physicians annotating data is small, there are not enough of them to provide data sets big enough to help build the algorithms healthcare desperately needs. To tackle this issue, in the case of medical images, developers often rearrange the images, doubling the database by mirroring the images or inverting their colour (6). This way, the annotation is still helpful, but the size of the database becomes bigger, thus leading to more accurate algorithms. However, this is not ideal as the more originally annotated scans the algorithm sees, the more accurate it becomes. Simply put, more data annotator physicians, nurses and medical students are needed.
Therefore, it’s time to acknowledge their crucial role and more importantly, find ways of persuading more and more medical professionals to help annotate data. There have been examples of supporting their work, although the room for improvement here is huge.
For example, a smartphone app called DiagnosUs creates a community of medical professionals to help them analyze and annotate medical images and videos (7). By using a free tool that allows them to improve their clinical skills, compete with their peers, and winning prizes in competitions, they also contribute to improving datasets for A.I. Such platforms have a place in this space.
The Google-backed company DeepMind has built a working prototype of a device that can diagnose complex eye diseases in real-time (8). It performs a retinal scan, is analysed by algorithms to provide an urgency score and a detailed diagnosis in roughly 30 seconds. The system that was developed in conjunction with London’s Moorfields Eye Hospital can detect conditions such as glaucoma, diabetic retinopathy and age-related macular degeneration with the same level of accuracy as ophthalmologists. DeepMind claimed that if the product passes clinical trials and regulatory approvals, physicians at Moorfields will be able to use the product for free for an initial period of 5 years. This is also method to make data annotation attractive to medical professionals.
The American Medical Association released a guidance about how to use and implement A.I.-based algorithms into the practice of medicine in an evidence-based way (9). Such clear guidelines can help not only adjust such advanced technologies to the daily work of physicians but also to find the right incentives that make them motivated. Practical regulations and policies can also support the safe and fast adoption of A.I. Good examples include the regulatory frameworks of the FDA in the US (10), as well as a similar guide published by the NHS in the UK (11).
If data annotation becomes an appreciated, rewarded and respected part of practice, its immediate consequence will be making machine and deep learning algorithms much more precise in radiology, pathology, cardiology, oncology, among others in recognizing patterns, supporting diagnoses, and designing treatment pathways.
It is becoming a common notion that the role of A.I. is not to replace physicians, however, physicians using A.I. might replace those who do not. While it will take plenty of time to find out whether this is the case, we will only find out if enough data annotators participate in the process. Even now, they might be sitting in dark hospital rooms with bright screens in front of them annotating radiology or ophthalmology images so that in the near future, someone might be able to create a useful medical application from them.
Without the unsung heroes of data annotation, healthcare will never benefit from A.I. (Figure 1).
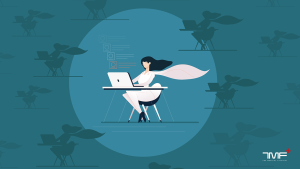
Acknowledgments
I'm grateful to Nóra Radó for her help with this manuscript.
Funding: None.
Footnote
Provenance and Peer Review: This article was a standard submission to the journal. The article did not undergo external peer review.
Conflicts of Interest: The author has completed the ICMJE uniform disclosure form (available at http://dx.doi.org/10.21037/jmai.2019.11.02). BM serves as an unpaid editorial board member of Journal of Medical Artificial Intelligence from Sep 2019 to Aug 2021.
Ethical Statement: The author is accountable for all aspects of the work in ensuring that questions related to the accuracy or integrity of any part of the work are appropriately investigated and resolved.
Open Access Statement: This is an Open Access article distributed in accordance with the Creative Commons Attribution-NonCommercial-NoDerivs 4.0 International License (CC BY-NC-ND 4.0), which permits the non-commercial replication and distribution of the article with the strict proviso that no changes or edits are made and the original work is properly cited (including links to both the formal publication through the relevant DOI and the license). See: https://creativecommons.org/licenses/by-nc-nd/4.0/.
References
- Topol EJ. High-performance medicine: the convergence of human and artificial intelligence. Nat Med 2019;25:44-56. [Crossref] [PubMed]
- Char DS, Shah NH, Magnus D. Implementing machine learning in health care - addressing ethical challenges. N Engl J Med 2018;378:981-3. [Crossref] [PubMed]
- Beam AL, Kohane IS. Translating artificial intelligence into clinical care. JAMA 2016;316:2368-9. [Crossref] [PubMed]
- Data annotators: the unsung heroes of artificial intelligence development. The Medical Futurist. 2019. Available online: https://medicalfuturist.com/data-annotation/
- Erickson BJ, Korfiatis P, Akkus Z, et al. Machine Learning for Medical Imaging. Radiographics 2017;37:505-15. [Crossref] [PubMed]
- Seif G. 3 ways to improve your Machine Learning results without more data. Towards Data Science. 2018. Available online: https://towardsdatascience.com/3-ways-to-improve-your-machine-learning-results-without-more-data-f2f0fe78976e
-
. Available online: https://apps.apple.com/ca/app/diagnosus/id1369759559DiagnosUs - Artificial intelligence group DeepMind readies first commercial product. Financial Times. 2019. Available online: https://www.ft.com/content/0e099914-514a-11e9-9c76-bf4a0ce37d49
- American Medical Association. Augmented intelligence in health care*. 2018. Available online: https://www.ama-assn.org/system/files/2019-01/augmented-intelligence-policy-report.pdf
- FDA. Proposed regulatory framework for modifications to artificial intelligence/machine learning (AI/ML)-based software as a medical device (SaMD) - discussion paper and request for feedback. 2019. Available online: https://www.regulations.gov/document?D=FDA-2019-N-1185-0001
- The NHS Constitution. Preparing the healthcare workforce to deliver the digital future. 2018. Available online: https://www.hee.nhs.uk/sites/default/files/documents/Topol Review interim report_0.pdf
Cite this article as: Meskó B. Data annotators are the unsung heroes of medicine’s artificial intelligence revolution. J Med Artif Intell 2020;3:1.