Prediction of low back pain using artificial intelligence modeling
Introduction
Back pain is one of the most common chronic diseases worldwide, with nearly a quarter of the world’s population suffering from chronic low back pain (1). In a study on the US veteran population, the annualized prevalence rate of patients with a diagnosis of lower back pain increased by 4.8% per year, compared to 3.8% for depression and 4.1% for hypertension (2). Like other chronic illnesses, back pain has profound effect on daily life with impairment in activities and decrease in quality of life. This leads to further hospital and primary care physician visits with an estimated $200 billion being spent annually on the management of back pain (3). In terms of diagnosing back pain, the first step is distinguishing mechanical pain from neuropathic pain. Most cases of back pain are mechanical in nature and respond to rest, ice, physical therapy and activity modification (4). Other conservative management modalities include acetaminophen, nonsteroidal anti-inflammatory drugs (NSAIDs), gabapentinoids, opioids and muscle relaxants. A further subgroup of mechanical back pain includes degenerative back pain, which includes osteoarthritis of the spine such as facet joint, sacroiliac joint, spinal stenosis and degenerative disc disease. In the older adult population, most of the low back pain has no definitive pathology and thus is classified as non-specific back pain. Panta et al. mention that in older population, disk degeneration is more prevalent; however, it is less likely to be the pain source as compared to in younger adults (5).
This paper explores the risk factors for low back pain in terms of radiologic data and applies artificial intelligence modeling to decipher the associations.
Methods
Datasets and analysis software
The dataset used for the analysis is available in public domain at the website (6) and is identified as PONE-D-14-50818. The dataset is provided under the Creative Commons by license and has been properly deidentified in compliance with the Health Insurance Portability and Accountability Act (HIPAA) laws. This data file contains the data used in the manuscript “Ligamentum Flavum Hypertrophy in Asymptomatic and Chronic Low Back Pain Subjects” by Munns et al. (7). As per the authors, the data set contains data for 63 individuals that were part of this IRB-approved study: 27 with chronic low back pain, and 36 as asymptomatic. All patients underwent magnetic resonance (MR) imaging and computed tomography (CT) of the lumbar spine. The MR images at the mid-disc level were captured and enlarged 800% using a bilinear interpolation size conversion algorithm that allowed for enhanced image quality. Ligamentum flavum thickness was assessed using bilateral medial and lateral measurements. Disc height at each level was measured by the least-distance measurement method in three-dimensional models created by CT images taken of the same subject (7). The data set consisted of 63 patients. Patient #35 was excluded from the analysis as it had missing disc height.
Statistical analysis
The statistical analysis was carried out in JMP 15 (8) and the artificial intelligence modeling was undertaken in Orange (version 3.26.0) (9). The modeling was carried out on all the 62 patients.
Ten different artificial intelligence machine learning and deep learning classification systems were implemented in order to predict the presence or absence of back pain. The classification systems consisted of logistic regression, Naïve Bayes, Neural network, decision tree, random forest (10), support vector machine (SVM) (11), Adaptive Boosting (AdaBoost) (12), Stochastic Gradient Descent (SGM), Constant (baseline model that always selects the most frequent class) and k-nearest neighbors (kNN). Models that employ regularization (e.g., logistic regression, SVM, all ordinal regression models), models based on model averaging (e.g., random forest), and models endowed with parameters for explicitly tuning model complexity (e.g., decision tree, random forest, kNN) were specifically added to the analysis as these characteristics are known to mitigate both variance and overfitting in small size datasets (13-15). In addition, a leave one cross validation was performed in order to reduce overfitting on the model, to avoid over estimation of the model performance and to allow for as much data as possible for training of the model (13). Hyper-parameter values were selected from a combination of literature and Orange modeling algorithms. The neural network analysis uses sklearn’s Multi-layer Perceptron algorithm and as such sklearn’s 100 neurons per hidden layer was set. The number of trees for the random forest model was set to 100 based upon Breiman’s random forest model analysis (16). A ten-fold cross validation was performed to assess the performance of each model. In this process, the data was split into ten groups and one group was chosen as the testing data while the remaining nine were training data. Each model was trained on the training data and then tested on the testing data. Next, a different group was chosen as the testing data and the remaining groups were designated as training data. The models were again trained and tested. This process was repeated until all ten groups had been designated as a testing data. The performance of each model on each of the ten testing data was averaged to obtain cumulative results. The receiver operating characteristic (ROC) curve was plotted for each model and then the area under the curve (AUC) for each ROC curve was calculated. The AUC for each model can be compared with each other to assess which model has a superior performance. AUC ranges from 0 to 1 with a higher value indicating a better ability to decipher whether the patient will have back pain. Measures, including classification accuracy, F1 score, precision and sensitivity are also calculated in order to compare the classification models. The study is in accordance with the Helsinki Declaration (as revised in 2013).
Results
There were a total of 62 patients of the age range 20–60 years. Thirteen (about 1/3rd) males out of 35 total males reported pain symptoms while 14 females (almost half) females out of 27 total females exhibited pain symptoms. The descriptive statistics for the data set are not being reported here as they have been reported earlier by Munns et al. (7).
Correlation
The correlation heat map for the disc heights for all the subjects (n=62) is given in Figure 1. The highest correlations were found for L1 disc height and L2 disc height followed by L2 disc height and L3 disc height. The correlation in the disc height is positive and of greater magnitude for the adjoining level discs. The correlation decreases as the distance of the discs increases. For example, the L5 disc height is highly positively correlated (correlation coefficient =0.47) with L4 disc height while negatively correlated with the farthest disc—L1 disc height. As such, if L4 disc height is low, disc heights of L3 and L5 are also low.
Classification tree
Classification tree (partition) (Figure 2) was generated in JMP 15. It is used to predict a categorical response as a function of predictor variables using recursive partitioning. The first branch of the diagram assesses the L4 disc height, indicating that this independent variable was the most important in separating patients with and without back pain. If the L4 disc height is greater than 8.43 mm, most of the respondents had no back pain whereas if the L4 disc height was below 6.24 mm, the respondents had the low back pain.
Logistic regression
The logistic regression of the study data with “symptomatic/asymptomatic lower back pain” (yes/no) was modeled as a binary output variable. The effect summary of the variables is provided in Figure 3. Based on the effect summary, the top three measurements with the greatest effect on back pain are L4 disc height, L3 ligamentum flavum mean medial and L4 ligamentum flavum mean medial. The logistic regression model results of the analysis are provided in Table 1. As per the logistic regression model L4 disc height, L2 disc height, and L3 mean medial thickness are significant risk factors or predictors for the lower back pain.
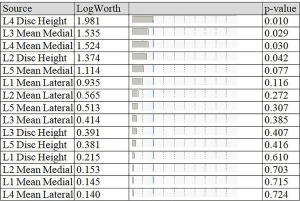
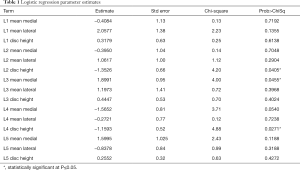
Full table
Artificial intelligence predictive modeling
The data was also modeled by various Artificial Intelligence methods to predict if the L1–L5 disc height and L1–L5 ligamentum flavum medial and lateral measurements can be used to predict presence or absence of lower back pain. The evaluation results of the various models are provided in Table 2. The top five machine learning and deep learning models as determined by all five measurements (AUC, classification accuracy, F1 score, precision and sensitivity) were logistic regression, Naïve Bayes, SVM, neural network and random forest. All five models had AUC greater than 0.7 and logistic regression had AUC greater than 0.8, meaning that the models can effectively predict low back pain based on quantitative measurements obtained from Lumbar imaging.
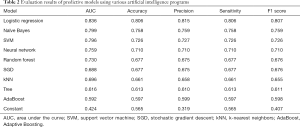
Full table
Nomogram
A nomogram (Figure 4) was created using SVM modeling. The nomogram depicts various probabilities at different L1–L5 disc heights and L1–L5 mean medial and lateral ligamentum flavum thickness. By providing an easy-to-interpret visualization the nomogram can provide insight into the effects of predictive factors.
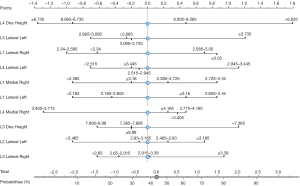
Discussion
Back pain is widespread in adults and is a focus of many providers due to its effects on quality of life. Less than 50% of the primary care physicians have strong confidence in diagnosing the causes of chronic low back pain (17). There are many medical risk factors for low back pain, some of which are modifiable. Physicians urge patients to maintain healthy body weight with ideal body mass index less than 25 (18). Smoking is also associated with increased rate of back pain (19). There are several papers describing risk factors for low back pain in the elderly population. They are prone to develop chronic low back pain based on physical and psychosocial changes (20). They are also more likely to develop osteoporotic vertebral fractures and lumbar spinal stenosis. Interestingly, Williams et al. concluded that individuals age 80 or older were three times more likely to experience severe low back pain than individuals in their 50s (21). Our analysis only contained patients with ages from 20s to 50s and thus this association could not be examined. Under-treating such pain can have profound effect on the patient including sleep disturbances and deterioration of functional ability.
We examined the risk factors for low back pain in terms of radiologic data. In our logistic regression analysis, the L2 and L4 disk height was statistically significant in terms of predicting symptoms of low back pain and had appreciable effect on the lower back pain. This is clinically relevant as more the disk degeneration, the less the disk height and thus more the risk of increased back pain. There is evidence that women are more susceptible to osteoporotic fractures and subsequent low back pain (22). Our analysis did show a significant association between some lumbar disk heights and gender, but no significant association between gender and low back pain. The disk height association based on gender can have a confounding variable where a woman’s lumbar disk heights are more likely to be decreased compared to that of man’s based on the fact that women are likely to be shorter than men. Other risk factors for decreased disk height can be from vertebral fractures such as with corticosteroid use (23). This vertebral compression fracture can occur either in the center of the vertebral body or in the anterior portion of the vertebra resulting in a kyphotic deformity since the anterior wall heals without regaining height (24). These result in asymmetrical biomechanical load on the vertebral endplate causing an inflammatory response in the endplate and on adjacent vertebral body, resulting in lower back pain. In our analysis, there was a positive correlation between reduced lumbar disk height at one level and reduced disk height at the lumbar level below. The aforementioned inflammatory response can induce bone marrow edema. Another interesting study found that bone marrow edema was more prevalent in adults with lower back pain than in patients without lower back pain (25). Furthermore, the location of bone marrow edema on MR image was closely associated with local lumbar tenderness. There needs to be further studies to evaluate association between disk degeneration and low back pain.
Usually the management of low back pain includes conservative modalities before interventional approach is employed. Some of the minimally invasive interventional techniques for painful vertebral compression fractures include balloon kyphoplasty and percutaneous transpedicular vertebroplasty (26). A recent meta-analysis showed that vertebral augmentation procedures attenuate pain and correct the deformity without increased risk of new vertebral fractures, which the patient is at risk for, as mentioned earlier (26). In a retrospective analysis on kyphoplasty, Civelek et al. state that to avoid subsequent fracture in the same or adjacent level, vertebral body should be filled adequately (27).
In our analysis, the other broad variable we analyzed from radiographic data was thickness of the ligamentum flavum. The presence of degenerative spondylolisthesis with thickening of ligamentum flavum can result is severe lower back pain (28). Munns et al. reported that thickness of ligamentum flavum was significantly increased with older age, lower lumbar level (caudally) and chronic low back pain (7). This supports the compensation to increased mechanical forces exhibited at lower lumbar levels. In addition, as the lumbar disk undergoes degenerative process and disk height decreases, the thickness of ligamentum flavum at that lumbar level increases. Interestingly, our analysis also found an inverse correlation between disk height at one lumbar level and ligamentum flavum thickness at the lumbar level above. There are several implications for ligamentum flavum hypertrophy. It can cause a decrease in spinal canal area causing lumbar spinal stenosis. Hansson et al. performed magnetic resonance imaging (MRI) before and after an external axial load and showed ligamentum flavum to be the primary cause of canal encroachment with up to 85% reduction in canal area (29). Since axial loading causes disk height loss, ligamentum flavum hypertrophy can thus produce low back pain symptoms. In our analysis, the L3 mean medial thickness and L4 mean medial thickness were statistically significantly associated with low back pain. This could be due to decreased L4 disk height, which could be placing mechanical stress on the ligamentum flavum at and above the degenerative disk. The pathomechanism is poorly understood, but Sairyo et al. theorize that mechanical stress induces tissue damage, leading to release of inflammation, scarring and growth factors which finally induce fibrosis (30). Patient with lumbar spinal stenosis caused by ligamentum flavum hypertrophy are usually inappropriately treated. The debulking of ligamentum flavum is a possible treatment for patients who have poor quality of life due to pain and weakness (31).
The use of artificial intelligence and machine learning techniques have been employed to enhance understanding and management of chronic diseases (32). However, the utilization of such techniques in low back pain is limited. There have been efforts to use imaging software techniques to identify parameters such as pelvic tilt and sacral slope (33). We employed artificial intelligence and machine learning techniques to enhance the ability to detect patterns of clinical importance in low back pain patients. The limitations of our study may stem from the relatively small sample size and availability of MRI image data. A study by Oude et al. reported a modest accuracy of 72% for low back pain based on a model of nearly 1,300 fictional cases applied to real cases (34).
Conclusions
Data from MRI and CT of the lumbar spine showed a positive association of low back pain with decreased disc heights (statistically significant for L2 and L4) and a positive association with increased ligamentum flavum hypertrophy. Based upon the data, the logistic regression classification model was effectively able to use quantitative measures from lumbar imaging in order to predict the presence or absence of lower back pain. Such a model could be instrumental in clinical practice in deciphering lower back pain due to skeletal abnormalities. The artificial intelligence models can not only be used to predict back pain in new patients, but also each new patient’s data can be inputted into the model, leading to even higher accuracy of the model for future patients. The model output can help the pain specialist in quantitatively assessing the predominant risk areas and guide intervention thereof. We believe the future of data classification to decipher the association and assess low back pain prognosis will be based on artificial intelligence and machine learning techniques.
Acknowledgments
Funding: None.
Footnote
Conflicts of Interest: The author has completed the ICMJE uniform disclosure form (available at http://dx.doi.org/10.21037/jmai-20-55). The author has no conflicts of interest to declare.
Ethical Statement: The author is accountable for all aspects of the work in ensuring that questions related to the accuracy or integrity of any part of the work are appropriately investigated and resolved. The study is in accordance with the Helsinki Declaration (as revised in 2013).
Open Access Statement: This is an Open Access article distributed in accordance with the Creative Commons Attribution-NonCommercial-NoDerivs 4.0 International License (CC BY-NC-ND 4.0), which permits the non-commercial replication and distribution of the article with the strict proviso that no changes or edits are made and the original work is properly cited (including links to both the formal publication through the relevant DOI and the license). See: https://creativecommons.org/licenses/by-nc-nd/4.0/.
References
- Balagué F, Mannion AF, Pellisé F, et al. Non-specific low back pain. Lancet 2012;379:482-91. [Crossref] [PubMed]
- Sinnott P, Wagner TH. Low back pain in VA users. Arch Intern Med 2009;169:1338-9; author reply 1339. [Crossref] [PubMed]
- Freburger JK, Holmes GM, Agans RP, et al. The rising prevalence of chronic low back pain. Arch Intern Med 2009;169:251-8. [Crossref] [PubMed]
- Casiano VE, Dydyk, AM, Varacallo M. Back Pain. Treasure Island (FL): StatPearls Publishing, 2020.
- Panta OB, Songmen S, Maharjan S, et al. Morphological Changes in Degenerative Disc Disease on Magnetic Resonance Imaging: Comparison Between Young and Elderly. J Nepal Health Res Counc 2015;13:209-13. [PubMed]
. Available online: https://spinebiomechanics.figshare.com/articles/Ligamentum_Flavum_thickness_measurements/6820175Ligamentum Flavum - Munns JJ, Lee JYB, Espinoza Orías AA, et al. Ligamentum flavum hypertrophy in asymptomatic and chronic low back pain subjects. PloS One 2015;10:e0128321 [Crossref] [PubMed]
- JMP®, Version <15>. Cary, NC: SAS Institute Inc., 1989-2020.
- Demšar J, Curk T, Erjavec A, et al. Orange: Data Mining Toolbox in Python. J Mach Learn Res 2013;14:2349-53.
- Ho TK. Random decision forests. Proceedings of 3rd international conference on document analysis and recognition; Montreal, 14-16 August, 1995:278-82.
- Cortes C, Vapnik V. Support-vector networks. Machine Learning 1995;20:273-97. [Crossref]
- Freund Y, Schapire RE. A Decision-Theoretic Generalization of On-Line Learning and an Application to Boosting. J Comput Syst Sci 1997;55:119-39. [Crossref]
- Campagner A, Berjano P, Lamartina C, et al. Assessment and prediction of spine surgery invasiveness with machine learning techniques. Comput Biol Med 2020;121:103796 [Crossref] [PubMed]
- Anguita D, Ghio A, Greco N, et al. Model selection for support vector machines: Advantages and disadvantages of the Machine Learning Theory. The 2010 International Joint Conference on Neural Networks (IJCNN); 18-23 July, 2010.
- Skurichina M, Duin RPW. editors. Stabilizing classifiers for very small sample sizes. Proceedings of 13th International Conference on Pattern Recognition; 25-29 Aug, 1996.
- Breiman L. Random Forests: Statistics Department, University of California. Berkeley, CA 94720; January 2001. Available online: https://www.stat.berkeley.edu/~breiman/randomforest2001.pdf
- Cayea D, Perera S, Weiner DK. Chronic low back pain in older adults: What physicians know, what they think they know, and what they should be taught. J Am Geriatr Soc 2006;54:1772-7. [Crossref] [PubMed]
- Chou R, Shekelle P. Will this patient develop persistent disabling low back pain? JAMA 2010;303:1295-302. [Crossref] [PubMed]
- Hestbaek L, Leboeuf-Yde C, Kyvik KO. Are lifestyle-factors in adolescence predictors for adult low back pain? A cross-sectional and prospective study of young twins. BMC Musculoskelet Disord 2006;7:27. [Crossref] [PubMed]
- Wong AY, Karppinen J, Samartzis D. Low back pain in older adults: risk factors, management options and future directions. Scoliosis Spinal Disord 2017;12:14. [Crossref] [PubMed]
- Stewart Williams J, Ng N, Peltzer K, et al. Risk Factors and Disability Associated with Low Back Pain in Older Adults in Low- and Middle-Income Countries. Results from the WHO Study on Global AGEing and Adult Health (SAGE). PLoS One 2015;10:e0127880 [Crossref] [PubMed]
- Old JL, Calvert M. Vertebral compression fractures in the elderly. Am Fam Physician 2004;69:111-6. [PubMed]
- Downie A, Williams CM, Henschke N, et al. Red flags to screen for malignancy and fracture in patients with low back pain: systematic review. BMJ 2013;347:f7095. [Crossref] [PubMed]
- Dewar C. Diagnosis and treatment of vertebral compression fractures. Radiol Technol 2015;86:301-20; quiz 321-3. [PubMed]
- Nakamae T, Yamada K, Shimbo T, et al. Bone Marrow Edema and Low Back Pain in Elderly Degenerative Lumbar Scoliosis: A Cross-Sectional Study. Spine (Phila Pa 1976) 2016;41:885-92. [Crossref] [PubMed]
- Zhang H, Xu C, Zhang T, et al. Does Percutaneous Vertebroplasty or Balloon Kyphoplasty for Osteoporotic Vertebral Compression Fractures Increase the Incidence of New Vertebral Fractures? A Meta-Analysis. Pain Physician 2017;20:E13-28. [Crossref] [PubMed]
- Civelek E, Cansever T, Yilmaz C, et al. The retrospective analysis of the effect of balloon kyphoplasty to the adjacent-segment fracture in 171 patients. J Spinal Disord Tech 2014;27:98-104. [Crossref] [PubMed]
- Kalichman L, Hunter DJ. Diagnosis and conservative management of degenerative lumbar spondylolisthesis. Eur Spine J 2008;17:327-35. [Crossref] [PubMed]
- Hansson T, Suzuki N, Hebelka H, et al. The narrowing of the lumbar spinal canal during loaded MRI: the effects of the disc and ligamentum flavum. Eur Spine J 2009;18:679-86. [Crossref] [PubMed]
- Sairyo K, Biyani A, Goel VK, et al. Lumbar ligamentum flavum hypertrophy is due to accumulation of inflammation-related scar tissue. Spine (Phila Pa 1976) 2007;32:E340-7. [Crossref] [PubMed]
- Mekhail N, Vallejo R, Coleman MH, et al. Long-term results of percutaneous lumbar decompression mild(®) for spinal stenosis. Pain Pract 2012;12:184-93. [Crossref] [PubMed]
- Diller GP, Kempny A, Babu-Narayan SV, et al. Machine learning algorithms estimating prognosis and guiding therapy in adult congenital heart disease: data from a single tertiary centre including 10 019 patients. Eur Heart J 2019;40:1069-77. [Crossref] [PubMed]
- Champain S, Benchikh K, Nogier A, et al. Validation of new clinical quantitative analysis software applicable in spine orthopaedic studies. Eur Spine J 2006;15:982-91. [Crossref] [PubMed]
- Oude Nijeweme-d'Hollosy W, van Velsen L, Poel M, et al. Evaluation of three machine learning models for self-referral decision support on low back pain in primary care. Int J Med Inform 2018;110:31-41. [Crossref] [PubMed]
Cite this article as: Aggarwal N. Prediction of low back pain using artificial intelligence modeling. J Med Artif Intell 2021;4:2.