Artificial intelligence in the diagnosis and management of COVID-19: a narrative review
Introduction
Corona virus Disease 2019, or COVID-19, has over 90.9 million confirmed cases as per statistics from 12th January 2021 (1). It is caused by severe acute respiratory syndrome coronavirus-2 (SARS-COV-2), which is a single stranded RNA virus causing respiratory infection in humans (2,3). Compared to the previous strains causing severe acute respiratory syndrome (SARS) and middle eastern respiratory syndrome (MERS) infections, SARS-COV-2 has a much higher rate of infectivity, thereby succeeding to achieve a worldwide spread (1,3). However, it has a significantly lower death rate of 3.63% (as of March 2020)/7% (as of end of April 2020) while that of SARS was 11% and 37% for MERS 37% (3,4).
Rationale for the review
There are several issues faced in the management of COVID-19, which can be met through the involvement of artificial intelligence (AI). COVID-19 presents with pneumonia and flu-like symptoms, which makes it difficult to differentiate from other types of respiratory infections at an early stage, especially with the lack of large-scale screening programs (5). There is a high risk of community spreads owing to the lack of early diagnosis (refer to Figure 1 for its pathophysiology) (4,6). With the current levels of spread of COVID-19, it has been recognised that the efforts of health care professionals, alone, are not sufficient in controlling the outbreak, especially when most of the treatments and vaccines are still under the phase of clinical trials therapies (7). The inclusion of AI technologies helps physicians in reducing the challenges faced during the management of COVID-19, including the risk of self-contamination through positive cases, overburdening and limitations with quick decision making in stressful situations (8).
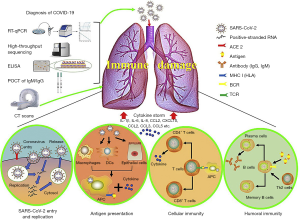
AI refers to the simulation of human intelligence by machines without being actively encoded with additional commands (9). During the pandemic crisis of COVID-19, it is being used for the screening of positive cases, description of predictive and analytical models in decision making, treatment planning as well as prediction of future cases and vaccine development (10). It is noteworthy that the global spread of COVID-19 was predicted by AI platforms such as Bluedot Global before the cases started from China (11). However, while AI is widely used for prediction, its use is limited with respect to diagnosis and treatment planning for COVID-19 in the present scenario. Further, its utilisations with respect to high-risk populations remain unclear.
Objectives
The purpose of this review is to explore the practical applications of AI in COVID-19 including its use in diagnosis, treatment planning, identification of high-risk cases and prevention. Its main objective is to identify artificial diagnostic tools that can be used in the present pandemic situations in order to streamline hospital procedures and reduce physician load. It also aims to answer the key question about the utilisations of AI for high-risk populations including the elderly, diabetic patients, hypertensive cases, asthmatic patients, pregnant women, cancer patient and post-transplant cases.
We present the following article in accordance with the Narrative Review reporting checklist (available at http://dx.doi.org/10.21037/jmai-20-48).
Methodology
The sources used for the review have been listed under Table 1.
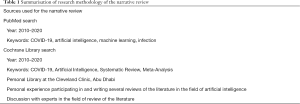
Full table
No prospective or retrospective data from human subjects has been collected for the purpose of this manuscript. Hence, no informed consent and ethical permissions were needed. The authors are accountable for all aspects of the work in ensuring that questions related to the accuracy or integrity of any part of the work are appropriately investigated and resolved.
Results
AI-based diagnosis facilitates early detection of COVID-19, which is one of its most significant advantages (12). In a clinical trial of 1,014 patients in Wuhan, it was found that the using chest CT for diagnosis is more sensitive for the diagnosis of COVID-19 when compared with RT-PCR tests (13). The use of machine learning (ML), trained on the basis of patient data from Wuhan, has been found to have a predictive accuracy of 80% for determining the patient prognosis (13).
Deep learning for the detection of COVID-19
Deep Learning-based predictive method is the most suitable method for diagnosis COVID-19 as well as complications such as pneumonia on the basis of data augmentation of radiographic images (DARI) algorithm and convolutional neural network (14). The DARI algorithm combines a generative adversarial network (GAN) model with generic augmentation techniques for the generation of synthetic radiographic data (14). This synthesised data assists in the scanning of CT images for the detection and monitoring of COVID-19 features on the radiograph (14). DL-based algorithms are efficient in uncovering hidden patterns within patient radiographs that serve as key to diagnosis (15). Anterior-posterior radiographs of the patient are perceived with high accuracy by DL models (96.3%) reducing the possibility of false positive and false negative results (15). In some studies, the specificity and sensitivity of AI technologies has been predicted to be as high as 100% indicating its possibility to minimise the manual interactions required by the radiologist for diagnostic procedures (15,16).
Deep neural network algorithm is of major diagnostic value in positive cases. It is based on real-time object detection system through training from X-ray images (17). Deep neural network model has a sensitivity of 85.35%, a specificity of 92.18% and an F1 score value of 87.37%; DarkCovidNet (a model of deep neural network) achieved this result (14,17). This makes it to be superior in comparison with the reverse transcription polymerase chain reaction (RT-PCR) test, which is conventionally used in the diagnosis of COVID-19 cases (sensitivity of 60% to 70%) (17,18). Some evidence has highlighted that AI-based models making the use of deep neural networks have comparable efficiency to radiologists in the process of diagnosis (19). The elaborate analysis of X-ray and CT parameters by AI has the potential to identify minute changes which could possibly be ignored by the physician (18). However, their use without the supervision of radiologists is currently not recommended because of the absence of clinical trials on COVID-positive patients.
Deep learning platforms also help in differentiating COVID-19 from the symptoms of flu, in cases where it is non-distinguishable by physicians (20). This is particularly helpful in low-income countries where a large-scale RT-PCR may not be economically feasible (20). Its sensitivity for differentiating COVID-19 from community—acquired pneumonia is 87% and its specificity is 95% (18). Thus, AI-based diagnosis of COVID-19 under the supervision of the physician is said to be time-saving, economical and is ascertained to reduce the burden faced by health care professionals as well as overcome the drawback of lowering number of RT-PCR test kits (21).
Discussion
AI is an efficient technique, which enables faster diagnosis of infectious diseases, their early prediction, large scale screening and efficient decision making (10). It also assists in public reporting by tracking high-risk individuals from the contact history of the identified positive cases (8,10). The applications of different AI tools in COVID-19 have been elaborated below.
Definitions and overview of AI tools used during COVID-19
Machine learning for predicting high-risk cases
ML is utilised for the integration of statistical models for the discovery of new knowledge from the data (22). It is used for developing prognostic algorithms for the prediction of the risk of mortality in COVID-19 positive cases. The use of ML helps to forecast the patients who will be most severely affected by COVID-19 enabling efficient treatment planning, early intensive care support and resource allocation for high-risk cases (23,24).
Deep learning and neural network for automated diagnosis
Deep learning involves the resolution of complex algorithms from unstructured, varied, and interconnected data (22). It assists in the automated diagnosis of COVID-19 for favouring early patient management (17). ResNet50 model with Support Vector Model classifier makes the use of X-rays to provide the best diagnostic results (17).
Neural networks refer to the development of tailored solutions from the study of patient’s data (22). They are used in the diagnosis of COVID-19 from the analysis of chest radiography images of patients (17). Neural networks also have a use in efficient patient monitoring and in providing regular updates of the case to the multi-disciplinary teams (19).
AI in the prediction of COVID-19 positive cases
AI and the use of infrared thermography are useful tools in the early diagnosis of COVID-19 (19,25). Fever is the most common early symptom reported in almost 83% of the patients as depicted by a recent meta-analysis (25). With the advancement of AI technologies, thermal scanners can be installed as mobile phone applications making it easier to screen large populations (26). This neural network-based contactless technology is used for self-screening as well as population-based screening before large gatherings, community-based activities and even at the point of entry to hospitals, restaurants or treatment centres in times of COVID-19 (26). Some facial-recognition companies such as Sense Time have integrated thermal imaging-based facial recognition of fever, which can be installed at entry points of public places for automated screening (25,26). This advances the social distancing protocol and minimises the need for human resource involvement. Other than thermal scanners, acoustic AI technologies are used for differentiating healthy patients from positive cases through the sound of their cough; however, the data on this is limited at the current stage (26).
Large-scale screening with AI technologies helps in building of the government database for ensuring timely tracking and notification of patient’s information to the relevant authorities (20). Through this sharing of data, AI has been efficiently trained for taking up bigger roles in the management of the pandemic including public health assistance through Chatbot applications, which are already being used in a large number of countries (20).
AI in the diagnosis of COVID-19
The most notable application of AI in the COVID-19 crisis is its role in diagnosis. AI tools quickly identify irregular patient symptoms or ‘red flags’ among hospitalized cases thereby instituting faster decision making in positive cases (10). Approximately, 15% of positive patients progress to have severe pneumonia, of which, 5% develop serious complications such as acute respiratory distress syndrome, sepsis, and multiple organ failure (5,18). The utilisation of AI technologies in this context helps to foresee patient deteriorations and advance decision-making processes (18).
AI in the treatment of COVID-19
Overview of treatment for COVID-19
Currently, the treatment offered against COVID-19 is only supportive aimed at preventing the worsening of patient symptoms due to the advancement of infection (5). The mainstay of clinical treatment involves symptomatic management; oxygen therapy and mechanical ventilation in case of respiratory failure (5) (refer to Table 2 for information on the current WHO prescribed treatment protocol). For patients with septic shock, hemodynamic therapy may be required (27,28).
Role of AI in the treatment of COVID-19
The current applications of AI in treatment include its role in telemedicine and decision making through the analysis of EHR and trial data (18). AI tools such as logistic regression models can be used for determining patient prognosis in cases of sepsis through the identification of biomarkers such as C-reactive protein and procalcitonin (28). This can help physicians in planning timely actions to prevent severe patient deterioration (28). By analyzing trial data, AI technologies can help in selecting the most suitable treatment options for the patient thereby enhancing patient outcomes (29). EHR analysis helps in the prediction of signs of clinical deterioration, thus, alarming physicians for planning of emergency management in positive cases (29).
Further, decision tree analysers and artificial neural networks have made it possible to design a real-time alarming system, which can be used during COVID-19 crisis (30). Medical decision support systems also allow the planning of treatment priorities (30).
Since most of these technologies have not been tested on COVID-19 cases, large-scale clinical trials need to be conducted before validating its use in treatment. Until then, AI can be used under close supervision of the physician for treatment planning.
Another role of AI in the management of COVID-19 is in the process of drug repositioning, wherein AI algorithms are used for predicting the protein structure of the virus, based on which, treatment protocols are designed with the help of biomedical graphs (31). Generated network complex, deep neural networks, conditional latent space sampling and fingerprint-based deep neural networks are used for drug discovery (32). They are also used for predicting the properties of the formulated drugs enabling their practical utilisation (32). Using similar techniques, AI technologies are also witnessing their role in the formulation of vaccine against COVID-19, which may conclude its spread (26).
Telemedicine during COVID-19
Telemedicine helps in providing treatment support to patients with mild discomforting symptoms due to conditions other than COVID-19 for minimizing their exposure to the SARS-COV-2 virus (21). It has been ascertained that patients with comorbid conditions are more likely to make the use of telemedicine practices rather than turning to a new practitioner in times of COVID-19 crisis (26). So, with the help of proper training of both physicians and patients, telemedicine can be used for the remote management of comorbid conditions (33). For patients living in remote areas, it can also be used for identifying patients at risk of COVID-19 through symptom-based analysis, following which, an RT-PCR can be prescribed (33). Through the involvement of interdisciplinary team in telemedicine practices, diagnosed positive cases can then be safely escorted to hospital settings reducing their interactions with health care staff members and other patients (33). The use of AI in this process ensures that possible COVID-positive patients are directly admitted to specialized wards, which minimizes the risk of exposure among non-COVID hospitalized patients (10).
Assessments to be made via telemedicine include regular checking of body temperature, calculation of respiratory rates and patterns, presence of cold/cough/fatigue/flu-like symptoms, generalized signs of illness and lymphadenopathy, which can be observed through patient-directed palpation of cervical lymph nodes while making the use of video conferencing tools (33).
Digital technologies are also used in the surveillance, control and management of patients living in remote areas during COVID-19 crisis. Innovative measures such as the use of drones are presently being employed for the transportation of medical samples for testing from remote areas (26). They are also used for commuting medical essentials for symptom-based management in these areas. Further, the use of surveillance drones helps in pointing out individuals who are not following lockdown/social isolation measures who are then followed up (26). Telemedicine is also helpful for remote management of high-risk population/individuals with comorbid conditions (refer to Table 3) (34-58).
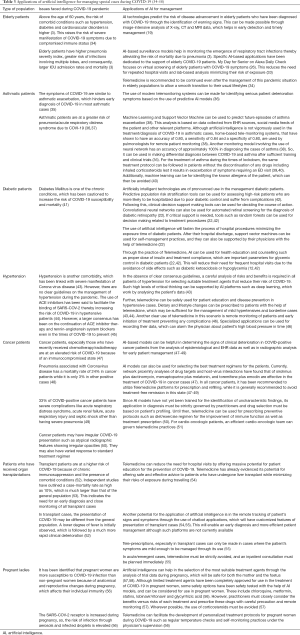
Full table
Rewards and limitations of the use of AI in the COVID-19 situation
AI helps in providing an early response to the pandemic by enabling efficient patient monitoring, screening and decision making for the prevention of large-scale community spread of COVID-19 (10). One of the major rewards of the use of AI in the management of the COVID-19 is that it helps in reducing the burden of health care professionals by managing the patient flow (9). Second advantage is that its practicable algorithms such as modified Susceptible-Exposed-Infectious-Removed (SIER) models help in providing informations about the epidemic peaks and sizes thereby helping individuals to take necessary precautions in time (59).
Well-trained AI models are also useful in providing insights into the disease patterns, which are not entirely understood by practitioners so far (32). One useful discovery made with the help of deep learning algorithms is that patients may continue to spread the disease even after their efficient recovery because diagnostic tests were found to be positive 5 to 13 days after the treatment (17). This discerns that suitable isolation and quarantine protocols must be followed even after successful treatment and recovery of the patient. Overall, the use of AI helps in enhancing patient safety due to early identification of complications and their logical management (24).
Despite these benefits, AI has some limitations, which is why its applications during the COVID-19 crisis have been limited. One of the major limitations is the lack of clinical trials to demonstrate the safety of use of AI in COVID-positive cases. This confines its use in treatment-related roles; however, it can be safely implemented in the form of telemedicine practices following appropriate national and international guidelines on their pragmatic use (refer to Table 3). There is also lack of training and input models, which are essential for the efficient functioning of superior AI systems such as big data analytics (45).
Summary
In the absence of supportive clinical trial data, AI has a limited role during the COVID-19 crisis. It can be used for patient education, thermal screening and early detection of symptoms through chatbot applications. AI can also be used for the remote management of mild symptoms in patients with comorbid conditions through the implementation of telemedicine in practice. For a bigger role such as in treatment planning/diagnosis, physician’s supervision is strictly recommended.
Limitations of the review
This narrative review is an early attempt at evaluating the applications of AI during the virulent spread of COVID-19. The quality of research evidence collected for our review is not sufficient to make a strong recommendation since majority of the findings have been gathered from grade III or grade V resources in the absence of randomized clinical trials encompassing the use of AI technologies on COVID-19 patients. With the current lack of clinical trial data and deficiency of clear national and international guidelines regarding the utilization of AI during COVID-19, we do not intend to recommend its applications at our discretion. However, our review provides solid directions for future research.
Directions for future research
Future clinical trials must focus on the comparison of AI-based automated diagnosis of pneumonia in COVID-19 patients with that of radiologists to expand its role in inpatient monitoring. Safety of telemonitoring in critical cases such as patients on active chemotherapy regimen must also be examined. Along with this, future research must elaborate how AI can be used as a prominent tool for managing patient flow in the times of the pandemic.
Acknowledgments
We acknowledge the efforts of Dr. Punit Srivastava and Dr. Garvita Arora of Mediception Science Pvt Ltd. for providing writing assistance.
Funding: None.
Footnote
Reporting Checklist: The author has completed the Narrative Review reporting checklist. Available at http://dx.doi.org/10.21037/jmai-20-48
Conflicts of Interest: The author has completed the ICMJE uniform disclosure form (available at http://dx.doi.org/10.21037/jmai-20-48). The author has no conflicts of interest to declare.
Ethical Statement: The author is accountable for all aspects of the work in ensuring that questions related to the accuracy or integrity of any part of the work are appropriately investigated and resolved.
Open Access Statement: This is an Open Access article distributed in accordance with the Creative Commons Attribution-NonCommercial-NoDerivs 4.0 International License (CC BY-NC-ND 4.0), which permits the non-commercial replication and distribution of the article with the strict proviso that no changes or edits are made and the original work is properly cited (including links to both the formal publication through the relevant DOI and the license). See: https://creativecommons.org/licenses/by-nc-nd/4.0/.
References
- WorldoMeters. Countries where COVID-19 has spread; 2020. Available online: https://www.worldometers.info/coronavirus/countries-where-coronavirus-has-spread/
- Li H, Liu SM, Yu XH, et al. Coronavirus disease 2019 (COVID-19): current status and future perspectives. Int J Antimicrob Agents 2020;55:105951 [Crossref] [PubMed]
- Liu K, Chen Y, Lin R, et al. Clinical features of COVID-19 in elderly patients: A comparison with young and middle-aged patients. J Infect 2020;80:e14-e18. [Crossref] [PubMed]
- Li X, Geng M, Peng Y, Meng L, et al. Molecular immune pathogenesis and diagnosis of COVID-19. J Pharm Anal 2020;10:102-8. [Crossref] [PubMed]
- Cascella M, Rajnik M, Cuomo A, et al. Features, Evaluation, and Treatment of Coronavirus (COVID-19). In: StatPearls [Internet]. Treasure Island (FL): StatPearls Publishing; 2021.
- Zhai P, Ding Y, Wu X, et al. The epidemiology, diagnosis and treatment of COVID-19. Int J Antimicrob Agents 2020;55:105955 [Crossref] [PubMed]
- Dong L, Hu S, Gao J. Discovering drugs to treat coronavirus disease 2019 (COVID-19). Drug Discov Ther 2020;14:58-60. [Crossref] [PubMed]
- McCall B. COVID-19 and artificial intelligence: protecting health-care workers and curbing the spread. Lancet Digit Health 2020;2:e166-e167. [Crossref] [PubMed]
- Ellahham S, Ellahham N. Use of Artificial Intelligence for Improving Patient Flow and Healthcare Delivery. J Comput Sci Syst Biol 2019;12:80-5.
- Vaishya R, Javaid M, Khan IH, et al. Artificial Intelligence (AI) applications for COVID-19 pandemic. Diabetes Metab Syndr 2020;14:337-9. [Crossref] [PubMed]
- Bogoch II, Watts A, Thomas-Bachli A, et al. Potential for global spread of a novel coronavirus from China. J Travel Med 2020;27:taaa011.
- Lalmuanawma S, Hussain J, Chhakchhuak L. Applications of machine learning and artificial intelligence for Covid-19 (SARS-CoV-2) pandemic: A review. Chaos Solitons Fractals 2020;139:110059 [Crossref] [PubMed]
- Ai T, Yang Z, Hou H, et al. Correlation of chest CT and RT-PCR testing in coronavirus disease 2019 (COVID-19) in China: a report of 1014 cases. Radiology 2020;296:E32-E40. [Crossref] [PubMed]
- Sakib S, Tazrin T, Fouda MM, et al. DL-CRC: Deep Learning-Based Chest Radiograph Classification for COVID-19 Detection: A Novel Approach. IEEE Access 2020;8:171575-89.
- Vaid S, Kalantar R, Bhandari M. Deep learning COVID-19 detection bias: accuracy through artificial intelligence. Int Orthop 2020;44:1539-42. [Crossref] [PubMed]
- Ozsahin I, Sekeroglu B, Musa MS, et al. Review on Diagnosis of COVID-19 from Chest CT Images Using Artificial Intelligence. Comput Math Methods Med 2020;2020:9756518 [Crossref] [PubMed]
- Ozturk T, Talo M, Yildirim EA. Automated detection of COVID-19 cases using deep neural networks with X-ray images. Comput Biol Med 2020;121:103792 [Crossref] [PubMed]
- Li L, Qin L, Xu Z, et al. Using Artificial Intelligence to Detect COVID-19 and Community-acquired Pneumonia Based on Pulmonary CT: Evaluation of the Diagnostic Accuracy. Radiology 2020;296:E65-E71. [Crossref] [PubMed]
- Neri E, Miele V, Coppola F, et al. Use of CT and artificial intelligence in suspected or COVID-19 positive patients: statement of the Italian Society of Medical and Interventional Radiology. La Radiologia Medica 2020;125:505-8. [Crossref] [PubMed]
- Ting DS, Carin L, Dzau V, et al. Digital technology and COVID-19. Nat Med 2020;26:459-61. [Crossref] [PubMed]
- Kapoor A, Guha S, Kanti Das M, et al. Digital healthcare: The only solution for better healthcare during COVID-19 pandemic? Indian Heart J 2020;72:61-4. [Crossref] [PubMed]
- Ellahham S. Artificial Intelligence: The Future for Diabetes Care. Am J Med 2020;133:895-900. [Crossref] [PubMed]
- Naudé W. Artificial intelligence vs COVID-19: limitations, constraints and pitfalls. AI Soc 2020; [Crossref] [PubMed]
- Ellahham S, Ellahham N, Simsekler MCE. Application of Artificial Intelligence in the Health Care Safety Context: Opportunities and Challenges. Am J Med Qual 2020;35:341-8. [Crossref] [PubMed]
- Fu L, Wang B, Yuan T, et al. Clinical characteristics of coronavirus disease 2019 (COVID-19) in China: A systematic review and meta-analysis. J Infect 2020;80:656-65. [Crossref] [PubMed]
- Kumar A, Gupta PK, Srivastava A. A review of modern technologies for tackling COVID-19 pandemic. Diabetes Metab Syndr 2020;14:569-73. [Crossref] [PubMed]
- Vellido A, Ribas V, Morales C, et al. Machine learning in critical care: state-of-the-art and a sepsis case study. Biomed Eng Online 2018;17:135. [Crossref] [PubMed]
- Garnacho-Montero J, Martín-Loeches I. Clinical management of sepsis can be improved by artificial intelligence: no. Intensive Care Med 2020;46:378-80. [Crossref] [PubMed]
- Rahmatizadeh S, Valizadeh-Haghi S, Dabbagh A. The role of Artificial Intelligence in Management of Critical COVID-19 patients. J Cell Mol Anesth 2020;5:16-22.
- Schinkel M, Paranjape K, Panday RN, et al. Clinical applications of artificial intelligence in sepsis: A narrative review. Comput Biol Med 2019;115:103488 [Crossref] [PubMed]
- Alimadadi A, Aryal S, Manandhar I, et al. Artificial intelligence and machine learning to fight COVID-19. Physiol Genomics 2020;52:200-2. [Crossref] [PubMed]
- Bullock J, Luccioni A, Pham KH, et al. Mapping the landscape of artificial intelligence applications against COVID-19. J Artif Intell Res 2020;69:807-45. [Crossref]
- Portnoy J, Waller M, Elliott T. Telemedicine in the Era of COVID-19. J All Clin Immunol. In practice 2020;8:1489-91. [PubMed]
- Schrack JA, Wanigatunga AA, Juraschek SP. After the COVID-19 pandemic: the next wave of health challenges for older adults. J Gerontol A Biol Sci Med Sci 2020;75:e121-2. [Crossref] [PubMed]
- Abrams EM, Szefler SJ. Managing Asthma during Coronavirus Disease-2019: An Example for Other Chronic Conditions in Children and Adolescents. J Pediatr 2020;222:221-6. [Crossref] [PubMed]
- Abrams EM, 't Jong GW, Yang CL. Asthma and COVID-19. CMAJ 2020;192:E551 [Crossref] [PubMed]
- Johnston SL. Asthma and COVID-19: is asthma a risk factor for severe outcomes? Allergy 2020;75:1543-5. [Crossref] [PubMed]
- Finkelstein J, Wood J. Predicting asthma exacerbations using artificial intelligence. Stud Health Technol Inform 2013;190:56-8. [PubMed]
- Alizadeh B, Safdari R, Zolnoori M, et al. Developing an Intelligent System for Diagnosis of Asthma Based on Artificial Neural Network. Acta Inform Med 2015;23:220-3. [Crossref] [PubMed]
- Messinger AI, Luo G, Deterding RR. The doctor will see you now: How machine learning and artificial intelligence can extend our understanding and treatment of asthma. J Allergy Clin Immunol 2020;145:476-8. [Crossref] [PubMed]
- Hussain A, Bhowmik B, do Vale Moreira NC. COVID-19 and diabetes: Knowledge in progress. Diabetes Res Clin Pract 2020;162:108142 [Crossref] [PubMed]
- Dankwa-Mullan I, Rivo M, Sepulveda M, et al. Transforming Diabetes Care Through Artificial Intelligence: The Future Is Here. Popul Health Manag 2019;22:229-42. [Crossref] [PubMed]
- Krittanawong C, Bomback AS, Baber U, et al. Future Direction for Using Artificial Intelligence to Predict and Manage Hypertension. Curr Hypertens Rep 2018;20:75. [Crossref] [PubMed]
- Schiffrin EL, Flack JM, Ito S, et al. Hypertension and COVID-19. Am J Hypertens 2020;33:373-4. [Crossref] [PubMed]
- Singh AK, Gupta R, Misra A. Comorbidities in COVID-19: Outcomes in hypertensive cohort and controversies with renin angiotensin system blockers. Diabetes Metab Syndr 2020;14:283-7. [Crossref] [PubMed]
- Peng F, Tu L, Yang Y. Management and Treatment of COVID-19: The Chinese Experience. Can J Cardiol 2020;36:915-30. [Crossref] [PubMed]
- Moujaess E, Kourie HR, Ghosn M. Cancer patients and research during COVID-19 pandemic: A systematic review of current evidence. Crit Rev Oncol Hematol 2020;150:102972 [Crossref] [PubMed]
- Yeoh CB, Lee KJ, Rieth EF, et al. COVID-19 in the Cancer Patient. Anesth Analg 2020;131:16-23. [Crossref] [PubMed]
- Al-Quteimat OM, Amer AM. The Impact of the COVID-19 Pandemic on Cancer Patients. Am J Clin Oncol 2020;43:452-5. [Crossref] [PubMed]
- Exercise Before, During, and After Cancer Therapy - American College of Cardiology. Available online: https://www.acc.org/latest-in-cardiology/articles/2019/12/04/08/22/exercise-before-during-and-after-cancer-therapy
- The Nuts and Bolts of an Inpatient Cardio-Oncology Consult Team - American College of Cardiology. Available online: https://www.acc.org/latest-in-cardiology/articles/2019/10/28/08/26/the-nuts-and-bolts-of-an-inpatient-cardio-oncology-consult-team
- Akalin E, Azzi Y, Bartash R, et al. Covid-19 and Kidney Transplantation. N Engl J Med 2020;382:2475-7. [Crossref] [PubMed]
- Bhoori S, Rossi RE, Citterio D, et al. COVID-19 in long-term liver transplant patients: preliminary experience from an Italian transplant centre in Lombardy. The lancet. Gastroenterol Hepatol 2020;5:532-3. [Crossref] [PubMed]
- Le LB, Rahal HK, Viramontes MR, et al. Patient Satisfaction and Healthcare Utilization Using Telemedicine in Liver Transplant Recipients. Dig Dis Sci 2019;64:1150-7. [Crossref] [PubMed]
- Concepcion BP, Forbes RC. The Role of Telemedicine in Kidney Transplantation: Opportunities and Challenges. Kidney 2020;360:34067.
- Zhao X, Jiang Y, Zhao Y, et al. Analysis of the susceptibility to COVID-19 in pregnancy and recommendations on potential drug screening. Eur J Clin Microbiol Infect Dis 2020;39:1209-20. [Crossref] [PubMed]
- Greiner AL. Telemedicine Applications in Obstetrics and Gynecology. Clin Obstet Gynecol 2017;60:853-66. [Crossref] [PubMed]
- Omer S, Ali S, Babar Z. Preventive measures and management of COVID-19 in pregnancy. Drug Ther Perspect 2020. doi:
10.1007/s40267-020-00725-x . - Yang Z, Zeng Z, Wang K, et al. Modified SEIR and AI prediction of the epidemics trend of COVID-19 in China under public health interventions. J Thorac Dis 2020;12:165-74. [Crossref] [PubMed]
Cite this article as: Ellahham S. Artificial intelligence in the diagnosis and management of COVID-19: a narrative review. J Med Artif Intell 2021;4:4.