Weibo users perception of the COVID-19 pandemic on Chinese social networking service (Weibo): sentiment analysis and fuzzy-c-means model
Introduction
The coronavirus disease 2019 (COVID-19) pandemic has spread in more than 200 countries and has caused many deaths (1). As of October 15, 2021, the death toll reached 4,876,778 (2). Research articles have indicated that many outbreaks and pandemics could have been promptly controlled if experts considered social media data (3). Over the last decade, social media analysis tools have been used to monitor public sentiment and communication methods for public health emergencies such as the Ebola (4) and Zika epidemics (5). With the World Health Organization (WHO) pandemic statement and various governments government action on the disease, various sentiments regarding COVID-19 have spread across the world. Therefore, sentiment analyses in studying pandemics, such as COVID-19, are important based on recent events.
Many studies have analyzed sentiment strength detection and detection of multiple emotions on social media, such as tweets (6-9). However, most of China’s social media (Weibo) analyses perform sentiment strength detection (6,10) and the emotions fall into only three categories: positive, neutral, and negative. Such analysis does not reflect its importance or the user’s emotions, e.g., happiness and sadness (11); anger (12); concern, surprise, disgust, or confusion (13). However, large-scale extraction of human emotions and entertainment from social media networks is critical for international public influence, business decision-making, and policymaking (14). Sentiment analysis is a powerful tool for understanding the most important events and trends. With this feature, large-scale communities can be observed at low cost (15).
In this study, seven emotions were set, and emotion classification was studied according to these seven emotions.
The fuzzy c-means (FCM) method used in this study is an unsupervised soft computing technology. It was developed by Dunn (16) in 1973 and improved by Bezdek (17) in 1981. The soft clustering method, as compared to the hard clustering method uses a fuzzy set (18), which can better solve the problem of text ambiguity. Membership in fuzzy sets, indicates the degree of matching between the element and the set, with membership values ranging from 0 to 1. The concept of membership was extended using FCM. In this method, the membership matrix represents the membership value of the elements in multiple clusters. FCM is one of the most used methods for solving fuzzy problems. Compared with other clustering methods, it is flexible and can accurately represent the degree of data affiliation (19).
The advantages of this method are as follows: (I) the number of words that constitute an sentiment lexicon can be reduced; (II) it can analyze words that are not in an sentiment lexicon; (III) it is suitable for accurately judging the ambiguity of people’s emotions.
This study aims to better understand the impact of public health emergencies on citizens and provide reference material for future public health emergency prevention. FCM was used to analyze seven different emotions related to Weibo’s content and track changes in these emotions over time.
Methods
The overall structure of the used method is shown in Figure 1. It contains eight parts. Preprocessing, keyword extraction, topic analysis, feature extraction, sentiment lexicon, clustering, and emotion classification. All calculation methods used in this experiment were implemented in Python.
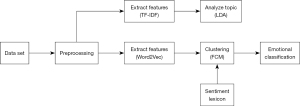
First, the raw data needs to be preprocessed. The TF-IDF method is then used to extract the keywords of the data to analyze people’s attentions. Then use the LDA method to analyze the topic of the data, which is used to analyze the aspects that people are interested in during the epidemic. And a skip-gram is used to extract word features and convert them into computer-processable data. Next, the processed data is clustered by the FCM method. At the same time, the lexicon containing seven emotions is clustered by the FCM method to obtain seven centers of emotions. Finally, the data is divided into seven emotions using the word vector of the words in each sentence and seven centers.
Data set
As shown in Figure 2, the data source used in this study was Weibo. The collection time was from January 24, 2020, to March 31, 2020. The keywords searched were “COVID-19 outbreak status” and “COVID-19 pneumonia” and the data collected totaled 1,367,842 user contents.
Preprocessing
The input dataset is preprocessed using normalization and python code. The preprocessing tasks are as follows:
- Excluding Weibo contents that have no meaning: URLs, images, etc.
- Removing special characters: remove all special characters (punctuation marks, question marks, exclamation marks, etc.) and replace them with spaces.
- Morphological analysis: extract only nouns, adjectives, verbs, and adverbs (NAVA).
Finally, there were 850,083 contents (only NAVA) that were used as data.
Sentiment lexicon
In this study, seven emotions were set based on Ekman, P, and Xu. The first six sentiments were set based on Ekman’s basic emotions (20), whereas the last was set based on Chinese local emotions (21). Table 1 lists the seven emotions and some of the corresponding words that represent them.
Table 1
Emotions | Representative words |
---|---|
Fear (惧) | 惶惶然 (Panic), 惊悚 (horror), 后怕 (fear), 恐惧 (fear), 担忧 (worry), 毛骨悚然 (horror), 吓人 (scary), 不寒而栗 (shuddering), 提心吊胆 (frightened), 心惊胆战 (frightened), 惧色 (fearful)… |
Happiness (乐) | 喜人 (Pleasant), 怡悦 (joyous), 欢快 (cheerful), 如意 (wishful), 得志 (happy), 欢娱 (entertaining), 庆幸 (fortunate), 喜气 (happy), 兴高采烈 (happy), 神采飞扬 (cheerful), 安适 (comfortable), 喜盈盈 (happy), 哈哈 (haha)… |
Disgust (恶) | 厌恶 (Disgust), 可憎 (abomination), 叛徒 (traitor), 欺凌 (bullying), 敲诈 (extortion), 屈辱 (humiliation), 轻蔑 (contempt), 卖友求荣 (betrayal), 奸险 (treacherous), 猥劣 (wicked), 奸诈 (treacherous), 诡诈 (deceitful)… |
Surprise (惊) | 错愕 (Wrong), 讶然失色 (shocked), 怔神儿 (stunned), 叹为观止 (stunned), 奇妙 (amazed), 惊诧 (shocking), 怪讶 (surprised), 惊人 (strangely surprised), 瞠目结舌 (amazing), 哄动一时 (stunned), 哗然 (shocked), 惊爆 (uproar)… |
Sadness (哀) | 悲恸 (Grief), 悲哀 (sorrow), 悲伤 (sadness), 啼泣 (weeping), 悲泣 (weeping), 号哭 (crying), 涕泗滂沱 (crying), 辛酸 (bitterness), 哀戚 (sorrow), 揪心 (worry), 哀怜 (pity), 哀哀 (sorrow), 苦处 (suffering)… |
Good (好) | 甜头 (Sweetness), 佳句 (good sentences), 佳趣 (good fun), 兼爱 (universal love), 坚守 (perseverance), 简雅 (simple and elegant), 味道好 (good taste), 立国安邦 (building a country and a nation), 崇敬 (reverence), 称颂 (praise), 传颂 (praise), 颂扬 (praise)… |
Anger (怒) | 忿忿不平 (Rage),怒火冲天 (anger), 气愤愤 (anger), 悲愤 (grief), 勃然大怒 (anger), 含怒 (anger), 恼羞成怒 (anger), 忿怒 (anger), 气急败坏 (frustration), 愤怒 (anger), 恼火 (anger)… |
Feature extraction
Feature extraction must be used to convert words in natural languages into computer-processable word vectors. In this study, the word2vec skip-gram (22) was used to extract features from the collected data.
The dimension of the word vector was set to 100 (23). Each word was represented as a 1×100 vector.
Statistical analysis
Term frequency-inverse document frequency (TF-IDF)
TF-IDF is a statistical method and used to evaluate the importance of a word to a document or a corpus. It is a commonly used weighting technique. As the number of a word occurrences in the file increases, the importance of that word increases.
In documents, the term frequency (TF) refers to the number of times a word appears in a document. Inverse document frequency (IDF) is a measure of the general importance of a word. A word’s IF-IDF is the value obtained by multiplying TF and IDF. The larger the IF-IDF of a word, the more important the word is in the document. TF-IDF formula are as follows.
Let D be a set of documents, denoted as:
where di represents the ith document in D.
Let d be a set of terms in D, denoted as:
where ti represents the ith term in d.
Term frequency
TFij is frequency of ti appear in dj, the formula is as follows:
where is the number of times term ti appears in a document dj. is total number of times that all terms appear in the document dj.
Inverse document frequency
The IDF is the total number of documents divided by the number of documents contain the ti. And then take the logarithm of the quotient. The formula is as follows:
Where, |M| is the total number of documents. is the number of documents containing the ti.
Term frequency-inverse document frequency
The TF-IDF is obtained by multiplying TFij and IDFi. It is defined as follows:
Latent Dirichlet Allocation (LDA)
The LDA model is a hierarchical Bayesian model consisting of three layers: document, topic, and word. These three-layer vector structures using the Bayesian algorithm, ignoring the order of word appearances in the document, and using the Bag-of-words model to transform the document set into a vector matrix. The LDA model is generated as shown in Figure 3.
Where K is the number of topics, |D| is the total number of documents, N is the number of words in the document, α is the Dirichlet proportions parameter of the topics for each document, β is the Dirichlet proportions parameter of the words of each topic. z is the topic number of words in the document, w is the word in the document, θd is the topic probability distribution in the document d, and φk is the word probability distribution of the kth topic.
The process of the LDA topic model is as follows.
- For the document d∈[1, |D|],
- Extract the topic probability distribution θd~Dir(α)
- Extract the document length Nd~Poiss(ξ)
- For topic k∈[1, K],
- Extract the mixed variable φk~Dir(β)
- For each word n∈[1, Nd],
- Extract the topic index Zd,n~Mult(θd)
- Generate words Wd,n~Mult(φzd,n)
In this study, we tested 3, 4, 5, 6, 7, 8, 9, 10 for topic count calculations and finally set the topic count to 5.
Fuzzy-c-means
The FCM method was used to cluster representative words of seven types of emotion dictionaries. The coordinates of seven centers (100 dimensions) were obtained.
FCM aims to minimize the following objective function:
where B is the objective function, N is the number of vectors, C is the number of clusters, xi is the degree of membership of xi in cluster j, xi is the ith value of d-dimensional measured data, cj is the d-dimension center of the jth cluster. The Euclidean distance between xi and cj.
The FCM clustering algorithm has five steps:
- Set the number c of clusters and the stopping condition.
- Calculate the cluster centroid.
- For each sensor point, compute the membership value for each cluster.
- Compute the objective function, shown in
Eq. [2]. If the value of A between consecutive iterations < ε, then stop. Otherwise, go to step II. - Assign each positioning index to a cluster after defuzzification.
Emotion classification
The membership value for each word of the seven emotions was calculated using the word vector for each word and the center coordinates of the seven emotions. The word membership value was then used to calculate the average membership value for each emotion in the sentence. The emotion with the highest degree of membership was the final emotion.
Results
TF-IDF values of COVID-19 related terms
Figure 4 shows the trend of COVID-19-related words in the top six TF-IDF values. From January to March, almost all the TOP keywords are related to the COVID-19 epidemic. People’s focus has changed from the outbreak of the COVID-9 in Wuhan to the number of confirmed cases and resumption of work. Due to limited space, we put the detailed results in the Tables S1-S5.
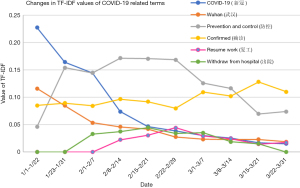
The topic extracted by LDA
Results for January 2020
Table 2 shows the topics extracted by LDA for January. It contains five topics “Infection control and measures”, “Epidemic situation in China”, “Supply of medical resources and social support”, “Information transmission and rumors”, “Support and medical personnel”.
Table 2
Topics | Words included in topic |
---|---|
Topic 0 Infection control and measures | Prevention and control (防控), epidemic (疫情), job (工作), infection (感染), COVID-19 (新冠肺炎), COVID-19 (新型冠状病毒), pneumonia (肺炎), respond to emergencies (应急), personnel (人员), do it well (做好), requirement (要求), pause (暂停), the masses (群众), fight (抗击), notice (通知), beginning (开展), united control (联防), notice (通告), back to school (开学), united control (联控), time (时间), command (指挥部), effort (全力), action (行动), about (关于), publish (发布), strengthen (加强), cancel (取消), period (期间), activity (活动) |
Topic 1 Epidemic situation in China | Medical case (病例), confirmed (确诊), add (新增), pneumonia (肺炎), COVID-19 (新型冠状病毒), infection (感染), patient (患者), report (报告), grand total (累计), epidemic (疫情), newest (最新), COVID-19 (新冠肺炎), discharged from hospital (出院), novel (新型), press conference (发布会), announce (通报), death (死亡), among (其中), cure (治愈), date (日时), as of (截至), nationwide (全国), suspected case (疑似病例), first case (首例), condition (情况), live broadcasting (直播), news (新闻), health commission (卫健委), dynamic (动态), prevention and control (防控) |
Topic 2 Supply of medical resources and social support | Prevention and control (防控), epidemic (疫情), fight (抗击), donate (捐赠), COVID-19 (新冠肺炎), pneumonia (肺炎), job (工作), supplies (物资), COVID-19 (新型冠状病毒), public health (公共卫生), event (事件), outbreak (突发), action (行动), Wuhan (武汉), infection (感染), start (启动), internationality (国际), response (响应), donate (捐款), Wuhan (武汉市), fight with epidemic (阻击战), attention (关注), win (打赢), organization (组织), Hubei (湖北), urgent (紧急), medical treatment (医疗), millions of people united as one man (万众一心) |
Topic 3 Information transmission and rumors | Anhui (安徽), propaganda (宣传), Henan (河南), COVID-19 (新冠肺炎), epidemic (疫情), be on guard (防范), prevention and control (防控), what (什么), government (政府), confirmed (确诊), knowledge (知识), information (信息), implore (恳请), forward (转发), which (哪些), rumor (谣言), manual (手册), close contact (密切接触), Zhoukou (周口), countryside (农村), science (科学), mask (口罩), rumors (传谣), importance (重视), about (关于), message (短信), source (来源), spread (传播), refute rumors (辟谣), why (为什么) |
Topic 4 Support and medical personnel | Wuhan (武汉), epidemic (疫情), we (我们), COVID-19 (新冠肺炎), virus (病毒), fight (抗击), effort (加油), COVID-19 (新冠), pneumonia (肺炎), hope (希望), everyone (大家), mask (口罩), self (自己), hospital (医院), nationwide (全国), millions of people united as one man (万众一心), novel (新型), China (中国), can (可以), no (没有), spring festival (春节), doctor (医生), this (这个), one (一个), medical personnel (医护人员), them (他们), how (如何), forefront of the fight (一线), pay tribute (致敬), must (一定) |
Results for February 2020
Table 3 shows the topics extracted by LDA for February. It contains five topics “Support and medical”, “Situation of overseas epidemics”, “Resume production and operation”, “Infection prevention measures (government)”, “Epidemic situation in China”.
Table 3
Topics | Words included in topic |
---|---|
Topic 0 Support and medical | Wuhan (武汉), epidemic (疫情), fight (抗击), forefront of the fight (一线), hospital (医院), effort (加油), we (我们), COVID-19 (新冠肺炎), them (他们), fight with epidemic (战疫), fight with epidemic (抗疫), Hubei (湖北), self (自己), action (行动), medical personnel (医护人员), medical team (医疗队), one (一个), support (支援), doctor (医生), prevention and control (防控), people (人民), pay tribute (致敬), front (前线), Jiangxi (江西), China (中国), medical personnel (医务人员), fight hard (奋战), together (一起), pneumonia (肺炎) |
Topic 1 Situation of overseas epidemics | Epidemic (疫情), COVID-19 (新冠肺炎), China (中国), Zhong Nanshan (钟南山), Japan (日本), Virus (病毒), Korea (韩国), end (结束), mask (口罩), Wuhan (武汉), COVID-19 (新冠), peak (峰值), we (我们), influence (影响), hope (希望), no (没有), Italy (意大利), express (表示), America (美国), beginning (开始), what (什么), worldwide (全球), appear (出现), nation (国家), everyone (大家), possible (可能), can (可以), already (已经), confirmed (确诊), nationwide (全国) |
Topic 2 Resume production and operation | Prevention and control (防控), epidemic (疫情), enterprise (企业), job (工作), resume work (复工), COVID-19 (新冠肺炎), mask (口罩), personnel (人员), period (期间), fight (抗击), epidemic prevention (防疫), supplies (物资), assure (保障), action (行动), community (社区), service (服务), since (以来), production (生产), donate (捐赠), boost (助力), do it well (做好), community (小区), resume production (复产), the masses (群众), beginning (开展), resume (恢复), fight with epidemic (阻击战), fight with epidemic (战疫), pneumonia (肺炎) |
Topic 3 Infection prevention measures (government) | Prevention and control (防控), epidemic (疫情), job (工作), response (应对), COVID-19 (新冠肺炎), press conference (发布会), convene (召开), about (关于), news (新闻), meeting (会议), command (指挥部), spread (传播), response (响应), COVID-19 (新型冠状病毒), pneumonia (肺炎), deploy (部署), respond to emergencies (应急), publish (发布), leader group (领导小组), notice (通知), developing (发展), health (健康), do it well (做好), back to school (开学), united control (联防), united control (联控), notice (通告), Xi Jinping (习近平), introduce (介绍), measure (措施) |
Topic 4 Epidemic situation in China | Medical case (病例), confirmed (确诊), add (新增), patient (患者), cure (治愈), discharged from hospital (出院), grand total (累计), COVID-19 (新冠肺炎), pneumonia (肺炎), report (报告), newest (最新), as of (截至), date (日时), epidemic (疫情), COVID-19 (新型冠状病毒), infection (感染), death (死亡), suspected case (疑似病例), map (地图), among (其中), hospital (医院), isolation (隔离), Hubei (湖北), treatment (治疗), date (年月日时), nationwide (全国), announce (通报), condition (情况), severe illness (重症), first case (首例) |
Results for March 2020
Table 4 shows the topics extracted by LDA for March. It contains five topics “Epidemic situation in China”, “medical correlation”, “Resume production and operation”, “Situation of overseas epidemics”, “Tokyo Olympics and economy”.
Table 4
Topics | Words included in topic |
---|---|
Topic 0 Epidemic situation in China | Medical case (病例), add (新增), confirmed (确诊), abroad (境外), entry (入境), report (报告), the epidemic was introduced from abroad (输入), COVID-19 (新冠肺炎), discharged from hospital (出院), cure (治愈), patient (患者), grand total (累计), date (日时), hospital (医院), risk (风险), as of (截至), epidemic (疫情), suspected case (疑似病例), isolation (隔离), pneumonia (肺炎), clear (清零), among (其中), personnel (人员), Beijing (北京), covid-19 (新型冠状病毒), date (年月日时), condition (情况), newest (最新), Hubei (湖北), announce (通报) |
Topic 1 medical correlation | Epidemic (疫情), COVID-19 (新冠肺炎), we (我们), Wuhan (武汉), China (中国), fight (抗击), them (他们), mask (口罩), hospital (医院), no (没有), hope (希望), fight with epidemic (抗疫), one (一个), self (自己), virus (病毒), that is (就是), Zhong Nanshan (钟南山), fight with epidemic (战疫), everyone (大家), forefront of the fight (一线), patient (患者), medical team (医疗队), doctor (医生), this (这个), people (人民), what (什么), COVID-19 (新冠), medical personnel (医护人员), effort (加油), medical treatment (医疗) |
Topic 2 Resume production and operation | Prevention and control (防控), epidemic (疫情), job (工作), about (关于), COVID-19 (新冠肺炎), resume work (复工), enterprise (企业), fight (抗击), resume production (复产), command (指挥部), personnel (人员), do it well (做好), community (社区), service (服务), notice (通知), period (期间), response (应对), beginning (开展), action (行动), leader group (领导小组), convene (召开), assure (保障), notice (通告), donate (捐款), back to school (开学), press conference (发布会), health (健康), orderly (有序), developing (发展), requirement (要求) |
Topic 3 Situation of overseas epidemics | Confirmed (确诊), America (美国), Italy (意大利), medical case (病例), COVID-19 (新冠肺炎), death (死亡), epidemic (疫情), grand total (累计), worldwide (全球), Trump (特朗普), China (中国), Korea (韩国), time (时间), add (新增), infection (感染), Britain (英国), COVID-19 (新冠), local (当地), nation (国家), virus (病毒), Europe (欧洲), Announce (宣布), France (法国), Iran (伊朗), Spain (西班牙), president (总统), number of people (人数), as of (截至), report (报道), WHO (世卫) |
Topic 4 Tokyo Olympics and economy | Epidemic (疫情), influence (影响), worldwide (全球), COVID-19 (新冠肺炎), economy (经济), China (中国), America (美国), Covid-19 (新冠疫情), virus (病毒), Japan (日本), COVID-19 (新冠), possible (可能), mask (口罩), express (表示), world (世界), vaccine (疫苗), market (市场), delay (推迟), plan (方案), Tokyo (东京), cancel (取消), response (应对), Olympic (奥运会), internationality (国际), announce (宣布), company (公司), nation (国家), plan (计划), pause (暂停), spread (蔓延) |
Overall result of Emotion classification
Judging from the overall results, “surprise” was the most common (475,102 cases, 55.89%). The second was “fear” (156,526 cases, 18.41%), and the third was “good” (117,799 cases, 13.86%). This is shown in Figure 5 and Figure 6.
Time series analysis of Emotion classification
As shown in Figure 7, the time series of emotions can be divided into three stages: the start stage (January 24–February 6, 2020), occurrence stage (February 7–March 10, 2020), and end stage in this study (March 11–March 30, 2020).
As shown in Table 5, “surprise” was higher in the start stage (average: 59.95%) and the end stage in this study (average: 66.17%) compared with the occurrence stage (average: 46.58%). But “surprise” remains higher than all the other sentiments throughout the period of the study. “Fear” was lower in the start stage (average: 15.42%) and the end stage in this study (average: 15.79%) compared to the occurrence stage (average: 20.95%). But “Fear” remains second in the period of the study. “Good” was lower in the start stage (average: 10.31%) and the end stage in this study (average: 8.46%) compared with the occurrence stage (average: 18.89%). But “good” was lower than “happiness” in start stage. “Happiness” was higher in the start stage (average: 12.73%) and the occurrence stage (average: 11.55%) compared with the end stage in this study (average: 7.41%).
Table 5
Emotions | Start stage (%) | Occurrence stage (%) | End stage (%) |
---|---|---|---|
Surprise (惊) | 59.95 | 46.58 | 66.17 |
Fear (惧) | 15.42 | 20.95 | 15.79 |
Good (好) | 10.31 | 18.89 | 8.46 |
Happiness (乐) | 12.73 | 11.55 | 7.41 |
Sadness (哀) | 1.35 | 1.62 | 1.38 |
Disgust (恶) | 0.09 | 0.32 | 0.53 |
Anger (怒) | 0.14 | 0.09 | 0.26 |
Discussion
In this study, keywords were extracted from the Weibo data related to COVID-19 from January 24 to March 30, 2020, and the topics that interest Weibo users were analyzed from keywords. And the sentiments of Weibo users were analyzed in chronological order.
Keyword extraction results (TF-IDF)
In this study, Weibo contents related to “New Coronavirus (COVID-19 outbreak situation)” were analyzed from January 1, 2020, to March 31, 2020 using the TF-IDF method. We analyzed the content of Weibo and clarified the content and changes that Weibo users were interested in in January, February, and March 2020.
The content posted by Weibo users was extracted by keyword using the TF-IDF method, and the top 25 words of TF-IDF values were calculated. Among these keywords, the content related to the COVID-19 epidemic has attracted a great deal of attention, and in particular, “COVID-19 epidemic situation”, “number of confirmed patients”, and “infection control effect” are of great interest. From January to March, all the TOP keywords are almost related to the COVID-19 epidemic.
Weibo users were very interested in the progress of treatment for COVID-19 and the development of vaccines. Since February, words such as “cure” and “Withdraw from the hospital” have appeared in the keywords of Weibo users’ discussions. At the same time, some Weibo users focused on and discussed whether the work environment of health care workers and medical resources could meet the supply and demand of patients. This is reflected in the appearance of keywords such as “hospital”, “health care workers”, and “Zhong Nanshan” in the discussions of Weibo users from January to March. Weibo users were also paying close attention to the real-time situation that is prevalent in China and abroad. Many Weibos contained real-time data on the outbreak of the new coronavirus infection.
Weibo users were also paying attention to the epidemics of mainland China, as well as much discussion about the epidemics and evolution of neighboring countries and other parts of the world. From February, interest shifted to Japan and South Korea, which are close to China, and to Italy and the United States, where the epidemic of the new coronavirus infection was serious. At the same time, a declaration (24) issued by Trump who was US President at that moment has made Mr. Trump’s name appeared frequently as a keyword in user discussions.
Changes in Weibo posts at different times and with different keywords reflect the process of the outbreak, development, and decay of the COVID-19 epidemic in China (25). The results show that January 2020 was an important time to prevent infection against the COVID-19 epidemic, during which time the epidemic and statistical data in China received widespread attention. In addition, since the new coronavirus infection was unknown, there has been a great deal of interest in the transmission model, symptoms, and preventive measures for the new coronavirus infection. People who experienced the SARS outbreak in 2003 also began to discuss the SARS. February 2020 and March 2020 are important times to curb the spread of the new coronavirus infection in mainland China (25). During this period, epidemic statistics and epidemics in China remain of great interest. But COVID-19 has already begun to spread to other countries and regions, so some Weibo users’ attention changes to neighboring countries and global pandemics. In Hubei, on March 25, 2020, lockdowns were gradually lifted. The resumption of work and production became one of the most concerned topics on Weibo.
Topic extraction results
In this study, Weibo contents related to “New Coronavirus (COVID-19 outbreak situation)” were analyzed from January 1, 2020, to March 31, 2020, using the LDA topic model method. A textual content analysis of Weibo content revealed potential topics and changes in content that Weibo users were interested in January, February, and March.
Topic content that Weibo users are interested in
We extracted 5 topics and manually summarized the topics from the extracted keywords. In these topics, the content on the epidemic of COVID-19 has received a great deal of attention, and the results from January to March include the content on the epidemic. Such as real-time data, the progress of treatment for COVID-19, and the development of vaccines. Also, as shown in the results of January and February, many Weibo users are focusing on and discussing measures to prevent and control the new coronavirus infection. Including but not limited to measures such as canceling all events, extending the Chinese New Year holidays, lockdowns in Wuhan, and even quarantining homes in provinces and cities across China. In addition, whether the supply of medical resources is sufficient, whether the patient’s treatment needs and the support of society to Wuhan City, Hubei Province can be met are also the focus of the user’s interest in January. And, since January is the early stage of the outbreak of the new coronavirus infection in China, the transmission method and case fatality rate of the new coronavirus are not yet clearly recognized, and it is easy to cause breeding and transmission of rumors (25). Therefore, in the result of January 2020, there are keywords related to rumors (26). Since late January, China’s national medical team has been supporting Wuhan City one after another. Weibo users’ discussions reflect this fact.
Weibo users were also very much aware of the real-time epidemic of new coronavirus infections outside mainland China. Many Weibo posts have provided real-time data on overseas epidemics, especially since it was reported in February that the number of confirmed cases was increasing overseas. Weibo users are not only keeping an eye on the epidemic situation in mainland China but also much discussing the development of epidemics in neighboring countries and other parts of the world. In February, the keyword of the Philippines appeared in the topic under discussion, because of the Philippines was announced as the first country to be confirmed dead overseas. From mid-February to late February 2020, the new coronavirus infection was first reported in China, followed by neighboring countries such as Japan, South Korea, and Russia. After that, the names of countries such as Thailand, Japan, South Korea, the United States, Italy, and Iran also appeared in the discussion, causing a heated debate among Weibo users. Since then, user interest has gradually shifted to economic recovery and resumption of operations and production in China. At the same time, With the declaration of a pandemic of the new coronavirus infection worldwide in March, users are paying attention to the outbreak of overseas epidemics. It should be noted that the decision to postpone the Japanese Olympics in the latter half of March became a hot topic on Weibo.
Comparative analysis with Twitter-related research
Recent studies have reported topics that Twitter users are paying attention to in relation to COVID-19 (27-30). The author analyzed four themes that Twitter users focused on from February 2nd to March 15th, 2020, and 12 themes related to COVID-19. They found that Twitter users were primarily focused on the impact of COVID-19 on people and countries. For example, many tweets mention the number of deaths associated with COVID-19 and its impact on the emotions and psychology of citizens. The economic impact of COVID-19 was also widely discussed. Compared to these findings, Weibo’s posts have some similarities. Topics such as economic and psychological impacts are of great interest on Weibo and Twitter (27-30). But at the same time, Weibo users’ attention is unique. First, Twitter users are looking at the causes and consequences of the new coronavirus infection, while Weibo users are looking more at prevention, control, and treatment. Prevention and control measures and epidemic conditions of various epidemics such as quarantine, detection, and co-prevention have caused the widespread interest of Weibo users (Tables 2-4). It is probable that this is because the Chinese government has taken a series of countermeasures to make the citizens aware of the seriousness and harm of this epidemic. As a result, Weibo users are more interested in health-related themes such as virus prevention, control, and treatment (Tables 2-4).
Overall result of Emotion classification
From the results of the overall analysis, it was judged that the citizens’ feelings toward COVID-19 were mainly “surprised” (55.88%). This is consistent with the results of Wang et al. (31) (surprise: 53.3%). Fan et al. (32) showed that Weibo’s emotions were primarily angry. Comparing the results of this study with those of Fan et al.’s study (31-33), it was found that the emotions of Weibo people during the outbreak of COVID-19 was mainly “surprise”.
Time series analysis of Emotion classification
Citizens’ emotions regarding COVID-19 can be classified into three parts: the start stage of COVID-19, the occurrence stage of COVID-19, and the end stage of COVID-19 in this study. The stages and emotions toward it were the same as that found by previous studies on Weibo (32-34). Compared to a previous study on Tweet (35), the emotions of the three stages and each stage are primarily the same, but the time partition of each stage is different because the user range is different. The details are as follows.
At the start of COVID-19 (January 24–February 6, 2020), people’s main feelings were “surprise”. The reason is that the citizens did not understand COVID-19 (contents about COVID-19 knowledge: 21.16% on the January 24, 2020) (36,37). At the same time, the mood of “happiness” fluctuated during this period, which was because of China’s “Chinese New Year (37)”.
During the COVID-19 outbreak (February 7–March 10, 2020), citizens’ feeling of “surprise” decreased (from 59.95% to 46.58%). This is because of the large-scale dissemination of knowledge regarding COVID-19 organized by scholars, such as Zhong Nanshan (38). A decrease in content related to COVID-19 knowledge (number of contents for COVID-19 knowledge: 4.19% on the February 6, 2020) indicates a decrease in public interest in COVID-19 knowledge. In addition, with the increase in the number of deaths (39), the feeling of “fear” increased from 15.42% to 20.95%. The feeling of “good” increased from 10.31% to 18.89%. Because people hope the situation of the COVID-19 epidemic will get better (26).
At the end of phase I of the COVID-19 pandemic (March 11–March 31, 2020), the feeling of “fear” decreased from 20.95% to 15.79% because the infectious disease was suppressed. The feeling of “good” decreased from 18.89% to 8.46%. However, the increase in “surprise” was inconsistent with the survey (31). This is because the public’s focus was on the epidemic situation in foreign countries such as Italy, India, Brazil, and France (40).
Limitations
This study has some limitations. First, it uses only Sina Weibo as a social media platform, and the data source for it was narrow. Because China is advancing into an aging society and most Internet users are young people, Sina Weibo users are mainly in the 18–41 age group (41). Representing the sentiment of the population of all age groups in China is impossible.
Second, only texts were used for this study to analyze emotions. Pictograms or symbols contained in sentences were not analyzed. Pictograms and symbols contain considerable emotional information (42), and emotions are lost if not processed.
Conclusions
The findings of this study indicate that January 2020 has been an important time to respond to the COVID-19 epidemic, during which time statistical data on epidemics and epidemics in China has received widespread attention. The sudden outbreak of the new coronavirus infection caused a lot of discussion about the spread of rumors, how it spreads, and infection control measures. February 2020 and March 2020 were critical times to curb the spread of this infection in mainland China (37). During this time, pandemic statistics and China’s pandemic situation remained of great interest. On the other hand, the fight against coronavirus infection and the growing interest in COVID-19 treatment and medical resources due to the rapid increased in the number of patients have been the topics of discussion among Weibo users during this period.
In addition, people’s feelings were analyzed regarding the COVID-19 pandemic in three stages over time. Throughout the period, the public’s attitude toward emergencies was a “surprise”. In the beginning, people’s emotions were primarily “surprised”; however after the outbreak, people’s “surprise” decreased with increasing knowledge. In addition, as the number of deaths increased, people felt “fear” and “good”. At the end of the phase, I of the COVID-19 pandemic, people’s “fear” and “good” feelings were diminished as the epidemic was suppressed. People’s interest shifted from China to other countries and their concern about the situation in other countries.
From the results, it is possible to understand whether a public health emergency is a public sentiment or an idea. Our findings facilitate an understanding of public discussions and emotions about the COVID-19 pandemic among Weibo users between January 24 and March 31, 2020. By analyzing these topics and emotions, we can provide reference materials and enable better preparation for a future public health emergency.
Acknowledgments
Funding: None.
Footnote
Conflicts of Interest: All authors have completed the ICMJE uniform disclosure form (available at https://jmai.amegroups.com/article/view/10.21037/jmai-21-36/coif). The authors have no conflicts of interest to declare.
Ethical Statement: The authors are accountable for all aspects of the work in ensuring that questions related to the accuracy or integrity of any part of the work are appropriately investigated and resolved.
Open Access Statement: This is an Open Access article distributed in accordance with the Creative Commons Attribution-NonCommercial-NoDerivs 4.0 International License (CC BY-NC-ND 4.0), which permits the non-commercial replication and distribution of the article with the strict proviso that no changes or edits are made and the original work is properly cited (including links to both the formal publication through the relevant DOI and the license). See: https://creativecommons.org/licenses/by-nc-nd/4.0/.
References
- World Health Organization. 2020.05.18. Coronavirus disease 2019 (COVID-19) Situation. Report 83. Available online: www.who.int/docs/default-source/coronaviruse/situation-reports/20200412-sitrep-83-covid-19.pdf?sfvrsn=697ce98d_4 (April 30, 2021)
- The report of Coronavirus disease 2019[in Chinese]. Available online: https://wp.m.163.com/163/page/news/virus_world/index.html?spss=epidemic
- Singh R, Singh R, Bhatia A. Sentiment analysis using Machine Learning technique to predict outbreaks and epidemics. International Journal of Advanced Science and Research 2018;3:19-24.
- Kim EHJ, Jeong YK, Kim Y, et al. Topic-based content and sentiment analysis of Ebola virus on Twitter and in the news. Journal of Information Science 2016;42:763-81. [Crossref]
- Lwin MO, Lu J, Sheldenkar A, et al. Strategic Uses of Facebook in Zika Outbreak Communication: Implications for the Crisis and Emergency Risk Communication Model. International Journal of Environmental Research and Public Health 2018;15:1974. [Crossref] [PubMed]
- Available online: http://wp.m.163.com/163/page/news/virus_report/index.html?_nw_=1&_anw_=1 (October 15, 2021)
- Hung M, Lauren E, Hon ES, et al. Social Network Analysis of COVID-19 Sentiments: Application of Artificial Intelligence. J Med Internet Res 2020;22:e22590. [Crossref] [PubMed]
- Dyer J, Kolic B. Public risk perception and emotion on Twitter during the Covid-19 pandemic. Appl Netw Sci 2020;5:99. [Crossref] [PubMed]
- Lwin MO, Lu J, Sheldenkar A, et al. Global Sentiments Surrounding the COVID-19 Pandemic on Twitter: Analysis of Twitter Trends. JMIR Public Health Surveill 2020;6:e19447. [Crossref] [PubMed]
- Xue J, Chen J, Hu R, et al. Twitter Discussions and Emotions About the COVID-19 Pandemic: Machine Learning Approach. J Med Internet Res 2020;22:e20550. [Crossref] [PubMed]
- Ali K, Dong H, Bouguettaya A, et al. Sentiment Analysis as a Service: A Social Media Based Sentiment Analysis Framework. 2017 IEEE International Conference on Web Services (ICWS) 2017:660-7.
- Ji X, Chun SA, Wei Z, et al. Twitter sentiment classification for measuring public health concerns. Soc Netw Anal Min 2015;5:13. [Crossref] [PubMed]
- Ji X, Chun SA, Geller J. Knowledge-Based Tweet Classification for Disease Sentiment Monitoring. Sentiment Analysis and Ontology Engineering 2016;639:425-54. [Crossref]
- Chung W, He S, Zeng D. emood: Modeling emotion for social media analytics on Ebola disease outbreak. International Conference on Information Systems, Texas, USA 2015.
- Choi S, Lee J, Kang MG, et al. Large-scale machine learning of media outlets for understanding public reactions to nation-wide viral infection outbreaks. Methods 2017;129:50-9. [Crossref] [PubMed]
- Li S, Wang Y, Xue J, et al. The Impact of COVID-19 Epidemic Declaration on Psychological Consequences: A Study on Active Weibo Users. Int J Environ Res Public Health 2020;17:2032. [Crossref] [PubMed]
- Dunn JC. A fuzzy relative of the ISODATA process and its use in detecting compact well-separated clusters. Journal of Cybernetics 1973;3:32-57. [Crossref]
- Bezdek JC, Ehrlich R, Full W. FCM: The fuzzy c-means clustering algorithm. Computers & Geosciences 1984;10:191-203. [Crossref]
- Zadeh LA. Fuzzy sets. In: Fuzzy sets, fuzzy logic, and fuzzy systems: selected papers by Lotfi A Zadeh. 1996:394-432.
- Ekman P. Basic emotions. Handbook of Cognition and Emotion 1999;98:16.
- Xu L, Lin H, Pan Y, et al. Construcing the Affective Lexicon Ontology. Journal of the China Society for Scientific and Technical Information 2008;27:180-5.
- Mikolov T, Chen K, Corrado G, et al. Efficient estimation of word representations in vector space. arXiv preprint arXiv:1301.3781 2013.
- Wang Y, Liu S, Afzal N, et al. A comparison of word embeddings for the biomedical natural language processing. J Biomed Inform 2018;87:12-20. [Crossref] [PubMed]
- Bohn K. Trump concedes US coronavirus death toll could be 100,000 or more. CNN, 2020. [2021-11-15]. Available online: https://www.cnn.com/2020/03/29/politics/trump-deaths-coronavirus/index.html
- White paper - Fighting Covid-19: China in Action. China Daily. [2021-11-15] Available online: https://covid-19.chinadaily.com.cn/a/202006/08/WS5edd8bd6a3108348172515ec.html
- Wang K, Wang S, Wang J, et al. Shaping and Publicization of Personal Information Spacec of COVID-19 Information Dissemination. J Phys: Conf Ser 2020;1574:012169. [Crossref]
- Rosenberg H, Syed S, Rezaie S. The Twitter pandemic: The critical role of Twitter in the dissemination of medical information and misinformation during the COVID-19 pandemic. CJEM 2020;22:418-21. [Crossref] [PubMed]
- Manguri KH, Ramadhan RN, Mohammed Amin PR. Twitter Sentiment Analysis on Worldwide COVID-19 Outbreaks. Kurdistan Journal of Applied Research 2020;5:54-65. [Crossref]
- Mutanga MB, Abayomi A. Tweeting on COVID-19 pandemic in South Africa: LDA-based topic modelling approach. African Journal of Science 2022;14:163-72.
- Abd-Alrazaq A, Alhuwail D, Househ M, et al. Top Concerns of Tweeters During the COVID-19 Pandemic: Infoveillance Study. J Med Internet Res 2020;22:e19016. [Crossref] [PubMed]
- Wang Y, Gao J, Chen H, et al. The relationship between media exposure and mental health problems during COVID-19 outbreak. Fudan University Journal of Medical Science 2020;3:47.
- Fan R, Zhao J, Chen Y, et al. Anger is more influential than joy: sentiment correlation in weibo. PLoS One 2014;9:e110184. [Crossref] [PubMed]
- Chen X, Chang T, Wang H, et al. Spatial and temporal analysis on public opinion evolution of epidemic situation about novel coronavirus pneumonia based on micro-blog data. Journal of Sichuan University 2020;3:2. (Natural Science Edition).
- Yu S, Eisenman D, Han Z. Temporal Dynamics of Public Emotions During the COVID-19 Pandemic at the Epicenter of the Outbreak: Sentiment Analysis of Weibo Posts From Wuhan. J Med Internet Res 2021;23:e27078. [Crossref] [PubMed]
- Zhao Y, Cheng S, Yu X, et al. Chinese public’s attention to the COVID-19 epidemic on social media: observational descriptive study. Journal of Medical Internet Research 2020;22:e18825. [Crossref] [PubMed]
- Boon-Itt S, Skunkan Y. Public Perception of the COVID-19 Pandemic on Twitter: Sentiment Analysis and Topic Modeling Study. JMIR Public Health Surveill 2020;6:e21978. [Crossref] [PubMed]
- Chen H, Zhang K. Insight into the psychological problems on the epidemic of COVID-19 in China by online searching behaviors. J Affect Disord 2020;276:1093-4. [Crossref] [PubMed]
- Liu W, Guan WJ, Zhong NS. Strategies and Advances in Combating COVID-19 in China. Engineering (Beijing) 2020;6:1076-84. [Crossref] [PubMed]
- Zhu B, Zheng X, Liu H, et al. Analysis of spatiotemporal characteristics of big data on social media sentiment with COVID-19 epidemic topics. Chaos Solitons Fractals 2020;140:110123. [Crossref] [PubMed]
- China CDC. (CCDC) Available online: http://weekly.chinacdc.cn/news/TrackingtheEpidemic.htm
- Wang J, Zhou Y, Zhang W, et al. Concerns Expressed by Chinese Social Media Users During the COVID-19 Pandemic: Content Analysis of Sina Weibo Microblogging Data. J Med Internet Res 2020;22:e22152. [Crossref] [PubMed]
- Sina microblog data center. 2020.02.21. 2018. Sina microblog user development report [in Chinese]. Available online: https://data.weibo.com/report/reportDetail?id=433 (March 25, 2021).
Cite this article as: Han F, Cao YD, Zhang ZH, Zhang HJ, Aoki T, Ogasawara K. Weibo users perception of the COVID-19 pandemic on Chinese social networking service (Weibo): sentiment analysis and fuzzy-c-means model. J Med Artif Intell 2022;5:8.