A narrative review on radiotherapy practice in the era of artificial intelligence: how relevant is the medical physicist?
Introduction
Medical physicists play a critical role in radiation therapy by contributing to the processes of simulation, treatment planning, dose delivery, and post-treatment follow-up (1). It however appears that advances in technologies employed for radiation therapy procedures are gradually limiting the direct involvement of medical physicists in these clinical radiotherapy procedures. Technologies now incorporate artificial intelligence (AI) in the radiotherapy process; AI tools implement algorithms in radiation machines and imaging equipment to accurately plan and deliver radiation treatment (2). The need for AI in radiotherapy practice has become necessary because the workflow of radiation therapy is time-consuming due to the multiple manual inputs involving the medical physicist, radiation oncologist, dosimetrist, and radiation therapist, coupled with the increasing incidence of cancer (3,4). As a result, AI has the benefit of reducing human intervention, workload, and treatment technique bias, potentially improving plan quality and accuracy of treatment planning procedures (5). AI was first introduced in the United States in 1956 during the Summer Research Project at Dartmouth College (3,4). It uses machine learning to apply data-driven algorithms to copy human habits and deep learning to develop models (6,7). The computer algorithms execute tasks that need human intelligence to increase performance (8). For example, through AI, data obtained from computed tomography (CT) scans can instantly be uploaded to a treatment planning system without manual dose calculations. In addition, for complex body tissues involving many cross-sections, computerized planning systems via dose distribution algorithms are more accurate and feasible than manual dose calculations (2). For full clinical adoption of AI, a specialized multidisciplinary team (e.g., radiation oncologist, medical physicist, IT professional, and radiation therapist) who have a fundamental understanding of relevant AI models and patient cohorts are required to assess the strength and limitations of AI in radiation therapy to guarantee patient safety (5). However, the people who plan the treatments are frequently less familiar with these algorithms due to their limited knowledge underlying the theoretical principles of the algorithms. This means that the person who executes treatment planning operations other than the medical physicist will eventually become an expert. Hence, the role of a medical physicist may be shifted from treatment planning procedures (2). This review presents the applications of AI in radiotherapy practice, with the evolving role of the medical physicist in radiation therapy in the era of AI. We present the following article in accordance with the Narrative Review reporting checklist (available at https://jmai.amegroups.com/article/view/10.21037/jmai-22-27/rc).
Methods
This review provides an overview of the application of AI in the delivery of radiotherapy and examines the evolving role of the medical physicist in the radiotherapy workflow. A preliminary search of the literature on AI in radiation practice was conducted to identify relevant studies previously published. Table 1 below shows a summary of the search strategy.
Table 1
Items | Specification |
---|---|
Date of search | March 22, 2022 to May 2, 2022. |
Databases and other sources searched | PubMed, Scopus, and Science Direct |
Search terms used | Artificial intelligence, medical physicist, radiation therapy, radiation oncology, and treatment planning |
Time frame | 2002 to 2022 |
Inclusion and exclusion criteria | Articles published in the English Language were included in the review. Articles published in any other language were excluded |
Selection process | The search was conducted independently by all authors |
Content and findings
Applications of AI in radiotherapy practice
Monte Carlo (MC) simulation has been the acknowledged target standard for treatment planning methods in radiotherapy because of its ability to give a real physical interaction process in biological tissues. These simulations, on the other hand, are complex, time-consuming, and necessitate a significant amount of processing and data storage power. Using patient data, AI can provide more efficient, convenient, and tailored therapeutic practice in a shorter amount of time (9). Radiation therapy uses high-energy beams to kill cancer cells. The process is not straightforward but involves a multitude of steps in sequential order. There are five steps (Figure 1) in the general radiation therapy procedure.
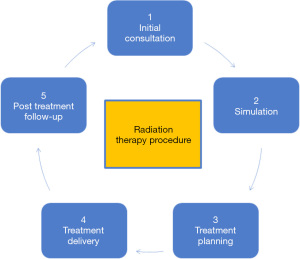
Initial consultation
The first step in the radiotherapy procedure is consultation, which can be frustrating especially when the waiting time for an appointment or consultation is too long (10). During consultation, the radiation oncologist decides on the most appropriate radiation treatment method using the clinical information obtained from the patient. Based on the medical history, pathology information, physical examinations, patient symptoms, and diagnostic report, the radiation oncologist can recommend treatment plans (8). To assist radiation oncology staff, AI can allow an integrated and thorough assessment of the patient’s status using all accessible information in oncology information systems. Because machines are generally dedicated to a specific duty, a radiation oncologist’s judgment can be more accurate than those made by AI machines (e.g., patient identification, prioritization, monitoring, or patient workflow checks). Radiation oncologists contact patients on a regular basis and are capable of understanding their unstated requirements and values. In this respect, AI technologies should be viewed as computer assistants to the staff, who would still be in charge of patient care (11). Before seeing a doctor, the AI module can assist in evaluating outpatients, depending on their main complaints by automatically scheduling imaging or laboratory tests. This would drastically reduce patient waiting times and, as a result, improve outpatient service processes (10). AI has proven to be promising in predicting pathological responses with treatment methods (e.g., chemotherapy, surgery, or radiation therapy) and treatment outcomes using patient data to conduct simulation routines (8).
Simulation
This phase of radiation therapy uses data obtained from the initial consultation to simulate the exact tumor location and configuration of the treatment beam via X-ray or CT scans. During this phase, the patient is immobilized to avoid gross body motion and target areas to be irradiated are accurately marked manually with a tiny tattoo dot. The procedure can be complex depending on the site of the tumor especially when it involves organ motion (8,12). The simulation phase includes image registration, synthetic CT (sCT)/MRI, image segmentation, motion management, etc.
Image registration
The process of determining a spatial transformation that maps two or more images to a common coordinate frame so that related anatomical structures are properly aligned is known as image registration (13). Many clinical applications use medical image registration, including image guidance, motion tracking, segmentation, dose accumulation, and image reconstruction (14). Image registration methods can be divided into intensity-based and feature-based. The (dis)similarity of the two images is described in terms of the correlation between pixel/voxel intensities in intensity-based registration (15). The intensity-based image registration approach immediately establishes the similarity measure function based on intensity information and then registers the images by performing the associated transformation. Examples of traditional algorithms used in this approach are cross-correlation, mutual information, and the sequence similarity detection technique (16). Image features (such as edges, lines, contours, and point-based features) are extracted prior to the registration process in feature-based registration approaches, and the correspondence between these features is utilized to establish the (dis)similarity. Both types of approaches rely on establishing the transform that minimizes a cost function that describes the dissimilarity between the fixed image and the transformed moving image, assuming one of the images is fixed and the other is moving (15). Examples of transformation models used to bring the images into the same coordinate system based on the region of interest (i.e., brain, thorax, lung, abdomen, etc.) include rigid, affine, parametric splines, and dense motion fields. The aligned images could then be used to supplement decision-making, allow for longitudinal change analysis, or guide minimally invasive therapy (17). Conventional image registration is an iterative optimization procedure that involves extracting proper features, choosing a similarity measure (to assess registration quality), selecting a transformation model, and finally, investigating the search space with a mechanism. Multi-modal image registration with the conventional method is not efficient and could cause stagnation or convergence. Besides, conventional image registration can be extremely slow due to the iterative manner of its implementation (18).
Deep learning AI algorithms can learn the optimum features for registration directly from the input data. The affine image registration deep learning model was effectively utilized to predict transformation parameters and register two 3D images using a twelve-element output vector modified from 2D DenseNet. To extract features from the image pair, an encoder was initially utilized. The characteristics were then concatenated as input to many fully connected layers, which used regression to fulfill the registration process (15).
Other types of deep learning AI models used in medical image registration include CNN, Staked Auto-Encoders (SAEs), Generative Adversarial Network (GAN), Recurrent Neural Network (RNN), and Deep Reinforcement Learning (DRL). Among these models, the CNNs are considered the most successful and powerful deep learning approaches since the entire image (or certain extracted patches) is fed directly to the network. In terms of accuracy and computing efficiency, the CNNs have surpassed similar existing state-of-the-art approaches. With CNN, Kernels are trained to extract the most important features by convolving with the input. The output of each layer, known as a feature map, is then fed into the next layer, and when the number of layers is large enough, a hierarchical feature set can be obtained, allowing the network to be classified as deep CNN. For the final classification, the feature maps of the last layer are concatenated and vectorized to feed a fully linked two or three-layer network (18). For instance, to forecast parameters for rigid-body transformations and conduct multi-modal medical image registration, synthetic images could be created from a manual transformation and trained with the CNN regression model. The model could be first trained on a large number of synthetic images and then fine-tuned with a smaller number of image sets (18). Researchers have obtained some results using deep learning algorithms in the registration of chest CT images, brain CT and MR images, 2D X-ray and 3D CT images since the first registered spinal ultrasonography and CT images using CNN (16).
Synthetic CT/MRI
Both CT and MRI images are vital to radiotherapy treatment planning workflow. Whiles CT images rely on electron density maps to calculate organ doses, MRI images are used to segment and delineate organs at risk (OARs) because of their exceptional soft-tissue contrast. sCT generation refers to the methods for generating electron density and CT images from MR images (19). There are three types of sCT generation methods: bulk density, atlas-based, and machine learning (ML) methods (including deep learning methods). The bulk density methods divide MRI images into various categories (usually air, soft tissue, and bone). The dose can then be estimated by assigning a homogenous electron density to each of these delimited volumes. The atlas-based approach on the other hand follows a fusion step to generate the sCT by registering one or more co-registered MRI-CT atlases with a target MRI (20). Guerreiro et al. (21) demonstrated the viability of treating children with abdominal malignancies using automatic atlas-based segmentation of tissue classes followed by a voxel-based MRI intensity to Hounsfield unit (HU) conversion algorithm.
Deep learning generates sCT by modeling the associations between HU CT readings and MRI intensities. Once the optimal DL parameters have been determined, the model can be used to create the associated sCT from a test MRI. Deep learning models have the benefit of being quick to generate sCTs, and some do not even require deformable inter-patient registration (20). Deep learning-based methods are gaining popularity for image synthesis because they can give a more complicated nonlinear mapping from input to output image through a multilayer and fully trainable model (19). CNNs are a common type of deep neural network (DNN) that detect image features using a series of convolution kernels/filters. It consists of three layers (i.e., an input layer, multiple hidden layers, and an output layer). Convolutions with trainable kernels are performed in the hidden layers (20). Han used a CNN model consisting of 27 convolutional layers interspersed with pooling and un-pooling layers and 37 million free parameters to create sCT using CT and T1-weighted MR brain tumor images of eighteen patients. When trained via principal transfer, the model was capable of learning a direct end-to-end mapping from MR images to their corresponding CTs. The average mean absolute error (84.8±17.3 HU) for all individuals was found to be much lower than the atlas-based method’s average mean absolute error (94.5±17.8 HU). When the mean squared error and the Pearson correlation coefficient were examined, the CNN approach showed much superior accuracy (22).
Image segmentation
The technique of extracting the desired object (organ) from a medical image (2D or 3D) is known as medical image segmentation. There are two types of segmentation: intensity-based segmentation and shape-based segmentation. The idea of the former is that voxels within an organ of interest have similar intensity (gray value), whereas the latter group assumes that the shape of the target to be segmented is roughly known (23). Auto segmentation algorithms based on machine learning AI can successfully contour structures with typical shapes or identify them from among other surrounding organs (24). For example, during segmentation users initially define precise imaging parameters and features, based on professional knowledge to extract the shapes, areas, and histogram of image pixels of regions of interest (i.e., tumor regions). To understand the features, a specific AI machine learning algorithm is chosen for training using part of the specified number of available data items, while the rest is used for testing. Principal component analysis (PCA), support vector machines (SVMs), and convolutional neural networks (CNNs) are examples of algorithms. The trained algorithm is then meant to recognize the features and classify the image for a particular testing image (25). The CNN, a key block in the building of deep networks, is the most efficient model for image analysis. CNN entails the creation of a number of optimization techniques, including LeNet, AlexNet, ZFNet, GoogLeNet, VGGNet, and ResNet. CNN is a robust feature extractor that can extract features from images and has a deep layer. Deep learning techniques, particularly convolutional networks, are quickly gaining popularity as a way of evaluating medical images (26). AI in three dimensions CNN was utilized to delineate nasopharyngeal cancer with a 79% accuracy, well-matched with delineation performed by a radiation specialist (8,24,27). With AI advancements, segmentation applications may be able to outline increasingly difficult structures including the prostate, spinal canal, and planning target volume (PTV) (28).
Motion management
Treatment of lesions in the thorax and upper abdomen is complicated by respiratory motion. Organs and tumors in the thoracic and abdominal cavities move in a dynamic and complex manner due to daily physiological changes, which can vary minute by minute or breath by breath. Breath-holding has become more common in breast irradiation to decrease pulmonary and cardiac damage. To accommodate for respiratory motion, respiratory motion management (RMM) has traditionally relied on the inclusion of considerable planning margins. The images obtained during simulation can be a useful source of respiratory motion data (29). AI algorithms can be applied to the motion data to optimize the simulation phase and correct for errors resulting from organ motion (8). AI can create strong-motion management models that account for motion variations, such as magnitude, amplitude, and frequency. These models can predict respiratory motion using data from external surrogate makers as inputs (30).
Using CNN and the adaptive neuro-fuzzy inference system, Zhou et al. (31) created an AI model to predict 3D tumor movements (ANFIS). Patients whose respiration-induced motion of the tumor fiducial markers exceeded 8 mm were given 1,079 logfiles of infrared reflective (IR) marker-based hybrid real-time tumor tracking. The CNN model’s historical dataset included 1,003 logfiles, with the remaining 76 logfiles supplementing the evaluation dataset. The logfiles captured the positions of external IR markers at 60 Hz and fiducial markers as surrogates for detected target positions every 80–640 ms for 20–40 seconds. The prediction models for each log file in the evaluation dataset were trained using data from the first three-quarters of the recording period. The percentage of projected target positions within 2 mm of the detected target position was used to rank the overall performance of the AI-driven prediction models. For the CNN and ANFIS, the projected target location was 95.1% and 92.6% within 2 mm of the detected target position, respectively. When compared to a regression model, AI-driven prediction models beat the regression model, with the CNN model doing marginally better than the ANFIS model overall (31).
Similarly, Lin et al. (32) also trained a super learner model that integrates four base machine models (i.e., Random Forest, Multi-Layer Perceptron, LightGBM, and XGBoost) to assess lung tumor movements in 3D space. As inputs, they used sixteen non-4D diagnostic CT imaging features and eleven clinical features derived from the Electronic Health Record (EHR) database. Tumor motion predictions in the superior-inferior (SI), anterior-posterior (AP), and left-right (LR) directions were generated by the super-learner model and compared to tumor motions detected in free-breathing 4D CT images. Mean Absolute Error (MAE) and Root Mean Square Error (RMSE) were used to assess prediction accuracy. In the SI direction, the MAE and RMSE of forecasts were 1.23 and 1.70 mm; in the AP direction, the MAE and RMSE of predictions were 0.81 and 1.19 mm; and in the LR direction, the MAE and RMSE of predictions were 0.70 and 0.95 mm. According to the findings, the super-learner model can reliably predict tumor motion ranges as assessed by 4D CT and could give a machine learning framework to help radiation oncologists decide on an active motion management strategy for patients with substantial tumor motion (32). Therefore, by accounting for changes in organ motion during the course of treatment, AI can enhance precision during adaptive radiation therapy to ensure that the proper dose is delivered at the right time to the right spot with little damage to healthy tissue.
Treatment planning
The primary goal of treatment planning is to deliver an appropriate dose of radiation to the tumor or cancer cells while minimizing the dose to surrounding healthy tissues as low as reasonably achievable (3). AI technology can extract and analyze a significant quantity of clinical data from medical records and generate cancer treatment options, allowing treatment plans to be developed automatically from the learning of clinical big data of cancer patients (27). Because of automated treatment planning (ATP), plan generating time and repetitive human interactions have both been reduced (33). Previously, machine learning techniques were the most common application in ATP based on the fact that many cases exhibit patient-geometrical and clinical-goal characteristics (34). More recently, deep learning algorithms are largely used to automatically generate treatment plans and optimal radiation doses for cancer patients (8). Xia et al. (35) created a deep learning-based automatic treatment plan for rectal cancer patients. A CNN was used in the segmentation of targets, OARs, as well as dose distribution. Manual segmentation was compared to PTV and OAR automatic segmentation. Between the auto and manual plans, there were no significant differences (35). There are different methods for generating automatic treatment plans, most of which are centered on a knowledge base DVH set derived from voxel-based dose distribution and open-source fluence map optimization (36).
Knowledge-based dose-volume histogram (DVH)
The knowledge-based DVH is one of the most reliable methods for achieving desired target dose conformity and sufficient sparing of critical structures in both IMRT and VMAT planning (37,38). The goal of knowledge-based planning (KBP) is to lessen the impact of user variability on final plan quality. This is accomplished by referring to a “model” that was created with the best treatment options in mind. Given the relative geometry and departmental planning technique, KBP determines the link between the dose to at-risk organs and treatment goals. Based on previous plans with similar characteristics, this information is utilized to estimate the DVH for each new patient (39). Information acquired from KBP can be used to create automatic treatment plans. Certain automated procedures can use this data as input to a treatment planning system. The development of automatic treatment plans entails analyzing similar prior “good” cases, which necessitates directly introducing certain planning characteristics (e.g., beam configurations and DVH objectives in inverse planning) or using them as decision references for a current case. Using best clinical judgment and knowledge, researchers have used statistical models to extract key aspects from earlier “good” cases (36).
Knowledge-based DVH prediction has demonstrated promising outcomes, particularly in the treatment planning of head and neck, pancreatic, and prostate cancers (37). Kostovski developed automated plan models for head and neck and prostate IMRT. The geometry of an organ at risk (OAR) relative to the PTV was represented by the distance-to-target histogram (DTH), and characteristic geometry and dosimetric features were derived from DTH and DVH by PCA. The method provided a precise dose prediction in both modeled sites. However, due to a lack of specific knowledge, the DVH-based technique was not perfect, as planners may need more time to address some instances with sophisticated OAR/target geometry (33).
Researchers have looked into various deep learning network designs for knowledge-based predictions during the last few years. For instance, U-Net, which was originally developed for image segmentation, has recently been utilized to forecast the radiation dosage distribution without the requirement for the sophisticated dose computations that are commonly employed in treatment planning (40). Zhong et al. (41) showed that by utilizing voxel-based dosage prediction, the clinical application of ATP for renal cancer patients is feasible. In their study, PTVs and contour delineation of the OARs were used as input and 3D dose distribution as output to create a 3D-UNet deep learning model. Intensity-modulated radiation treatment (IMRT) plans were developed automatically using post-optimization procedures based on the voxel-wise predicted dose distribution, including a complicated clinical dose goal metrics homogeneity index (HI) and conformation index (CI). The 3D dose distributions of the plan, as well as the DVH parameters of OARs and PTV, were compared to those of manual plans. The ATP system has the ability to generate IMRT plans that are clinically acceptable and equivalent to dosimetrist-generated plans (41). The U-Net design has also been employed in radiopharmaceutical dosimetry, where the network was trained to predict 3D dose rate maps from mass density and radioactivity maps (40).
RapidPlan (Varian Medical Systems, Palo Alto, CA, USA), a new commercial KBP optimization engine, was created and released for clinical usage. RapidPlan predicts feasible DVHs and produces optimization objectives automatically to actualize the prediction. Although the benefits of RapidPlan are still being researched, many reports of improvements in sparing OARs using KBP have been published. KBP’s mechanical and dosimetric accuracy have also been validated, indicating that it might be utilized safely in clinical practice (42).
The usage of KBP could aid workers who are not familiar with inverse planning, allowing them to produce automatic plans that are comparable to those produced by expert planners. Experienced planners may also benefit from a preliminary set of goals that will result in a high-quality plan, decreasing the time and effort required for plan optimization. KBP could also be used as a teaching tool for novice planners, as well as a tool to quantitatively verify the quality of an RT plan. This could result in higher average plan quality, lower inter-patient plan variability, faster patient throughput, and possibly better patient outcomes (39).
Auto fluence map optimization
A fluence map is a two-dimensional (2D) photon intensity image of a single beam that forecasts the dosage distribution of that beam in the patient. The fluence map optimization problem can be thought of as an inverse problem in which one specifies the desired dose distribution and then seeks to find the optimal beamlet intensity vector to actualize it. This prediction paradigm necessitates a second inverse optimization phase to convert the desired dosage to deliverable fluence maps that match machine specification parameters such as MLC leaf control points (43,44).
Wang et al. (43) utilized direct prediction of fluence maps and anticipated field dose distribution from CT images of patient anatomy to generate automatic treatment plans for pancreas stereotactic body radiation therapy. The method employed a deep learning model with two CNNs, one of which predicts the field-dose distributions in the region of interest and the other the final fluence map per beam. The anticipated fluence maps were input into a treatment planning system for leaf sequencing and dose computation. The gold standard for training the model was a nine-beam benchmark plan with standardized goal prescription and organ-at-risk constraints data. The quality of the automatic treatment plan was found to be clinically feasible when compared to manual planning (43). Ma et al. (45) also suggested a DL-based inverse mapping method for predicting fluence maps for desired VMAT dose distributions, which was backed up by a theoretical foundation. Clinical Head and Neck full-arc VMAT designs were used to train and evaluate this fluence map prediction approach (45).
In a different study, a DNN was effectively employed to automatically forecast clinically acceptable dose distributions from organ outlines in IMRT. Organ outlines and dose distributions from 240 prostate plans were used in training the DNN. With just a few errors, the DDN was able to generate fluence maps. Following training, 45 synthetic plans (SPs) were created using the generated fluence maps. The SPs were then compared with clinical plans (CPs) using a variety of plan quality metrics such as homogeneity and conformity indices for the target and dose constraints for OAR such as the rectum, bladder, and bowel. The SPs’ attributes were comparable to those of the corresponding CPs. The time it took to generate fluence maps and the quality of SPs showed that the suggested method has the potential to increase treatment planning efficiency and assist preserve plan quality (46).
A software system and method for the automatic prediction of fluence maps and subsequent generation of radiation treatment plans have been created by Duke inventors and colleagues. This software employs deep learning neural networks to estimate field dosage distribution based on patient anatomy data and treatment goals. Deep learning-based projections of corresponding beam’s eye view projections are used to forecast a final fluence map per beam. These fluence maps have been validated in dozens of retrospective pancreatic, prostate, and head-and-neck cancer cases, resulting in treatment plans that are clinically comparable to those created by human planners (47).
Treatment delivery
The patient on the first day of treatment is placed in position for treatment with the aid of immobilized devices for delivery of the prescribed radiation dose (8). Due to respiratory movements, complete immobilization of a patient is not possible, thereby compromising the precision of irradiation. Artificial Neural Networks have been proven to be effective in predicting tumor location from respiratory motion measures, allowing adaptive beam realignment to take place in real-time (48). Also, significant changes in a patient’s anatomy between the planning appointment and therapy delivery, or even during treatment, may necessitate re-planning. These fluctuations are frequently due to tumor-shrinking or growth, as well as physical variables such as bowel movement, which could result in different dosages to the tumor and organs. Machine learning AI has been developed to identify potential candidates for re-planning intervention as well as the best timing to do so (48). In addition, accurate placement of the patient in a reproducible position during the simulation phase is critical and can be achieved with high-quality images. With AI tools, the quality of cone-beam computed tomography (CBCT) images commonly used for positioning the patient can be enhanced through multimodal imaging techniques. During radiation delivery, patient-specific dynamic motion management models are used to correct for changes in patient or organ motion (8).
Modern radiotherapy necessitates high accuracy standards and methodologies for predicting dose distribution variations that occur during treatment administration. AI can be utilized to extrapolate a dose that is really administered to the patient. To predict these disparities from plan files (i.e., leaf position and velocity, movement toward or away from the isocenter), a machine learning approach was devised. When comparing the predicted leaf position to the delivered leaf position, the results showed that the predicted leaf position was significantly closer than planned, resulting in a more realistic representation of plan delivery (33).
Patients who miss radiation therapy sessions during cancer treatment have a higher risk of their disease returning, even if they finish their course of radiation treatment. In a study published by Chaix et al. (49) in 2019, a “chatbot” was designed to assess the efficacy of text message interactions utilizing AI technologies among breast cancer patients. Patients were quite responsive to medication reminders, and many of them suggested the “chatbot” to their friends (49) due to its effectiveness.
Post-treatment follow-up
This is the final phase of radiation therapy where the response to treatment and overall health of the patient is monitored at specific periods. AI can offer information on tumor response throughout treatment and predict cancer-specific outcomes such as disease progression, metastasis, and overall survival of patients (8). AI technology was integrated with radiomics to create a predictive model that could evaluate the response to bladder cancer treatment (28). To characterize an image in radiomics, quantitative parameters such as size and shape, image intensity, texture, voxel relationships, and fractal properties are retrieved. The image-based attributes can then be correlated with biological observations or clinical outcomes using machine or deep learning methods (50). During follow-up, data was collected to build a model and this model can be then used during the consultations to guide clinicians to select the best treatment options (8).
Quality assurance (QA) in radiation therapy
The medical physicist is mainly in charge of QA in radiotherapy. Machine QA, patient-specific QA, and delivery errors are the three basic types of QA in radiotherapy. Machine QA involves evaluating the performance of radiation medical devices like linear accelerators, electronic portal imaging devices, onboard imaging, and CT scanners. During machine QA, image quality mechanical and dosimetric qualities are constantly evaluated. On the other hand, patient-specific QA tasks involve in vivo dosimetry, monitor units, and dosimetric measurements of patient treatment plans. Dosimetric measurements are performed in phantoms with one of the following detectors: ion chamber matrix, films, or electronic portal imaging devices. The third type of radiation QA looks for delivery errors in log files generated during treatment (51). Errors detected during delivery are used to minimize or prevent problems associated with the linac systems. Several aspects of a radiation QA program have been documented in the areas of error detection and prevention, treatment machine QA, and time-series analysis. Examples of these areas include predicting linear accelerator performance over time, detection of problems with the Linac imaging system, prediction of multi-leaf collimator positional errors, detection anomalies in QA data, quantifying the value of quality control checks in radiation oncology, predicting planning deviations from the initial intentions and predicting the need for re-planning, and predicting when the head and neck of patients treated with photons will require re-planning, etc. (50).
AI can help medical physicists with machine QA to develop automated analysis tools to identify image artifacts for QA of onboard imaging, QA of gantry sag and MLC offset, prediction of dosimetric symmetry of beams using CNN time series modeling, and prediction of dose profiles for different file sizes during the commissioning of linear accelerator systems (51). QA of linac commissioning can be labor-intensive and time-consuming. Machine learning can be used to lessen the work burden of linac commissioning by creating an autonomous prediction model based on the data collected during commissioning. Specifically, one can train a machine-learning algorithm to simulate the intrinsic correlation of beam data under various configurations using previously obtained beam data, and the trained model can then create accurate and trustworthy beam data for linac commissioning for everyday radiation (31). For instance, using patient-specific planar dose maps from 186 IMRT beams from 23 IMRT plans, Nyflot et al. (52) studied a deep learning approach to categorize the presence or absence of radiation treatment delivery errors. For planning, each plan was supplied to the electronic portal imaging device. The doses measured from the planning were then used to create gamma images. A CNN with triplet learning was used to extract image features from the gamma images. A handcrafted technique based on texture features was also used for the same purpose. In order to assess if images had the added errors, the resulting metrics from both procedures were fed into four machine learning classifiers. 303 and 255 gamma images were used for training and testing the model respectively. The deep learning strategy yielded the highest categorization accuracy (52). El Naqa et al. (53) also studied machine learning algorithms for gantry sag, radiation field shift, and multileaf collimator (MLC) offset data gathered using electronic portal imaging devices for prospective visualization, automation, and targeting of QA (EPID). Nonlinear kernel mapping with support vector data description-driven (SVDD) techniques was employed for automated QA data analysis. The data was collected from eight LINACS and seven institutions. At four cardinal gantry angles, a standardized EPID image of a phantom with fiducials provided deviation estimates between the radiation field and phantom center. The gantry sag was determined using horizontal deviation measurements, while the field shift was determined using vertical deviation measurements. With changing hypersphere radii, these measurements were entered into the SVDD clustering algorithm. MLC analysis revealed one outlier cluster that matched with TG-142 limits along the Leaf offset Constancy axis. Machine learning algorithms based on SVDD clustering show promise for establishing automated QA tools (53).
QA of dose distribution can also be more difficult with some radiation therapy techniques such as volumetric modulated arc therapy (VMAT) and intensity-modulated radiation therapy (IMRT) which use both point dose and 2D plane dose measurements (54). During treatment planning, gamma index QA is one of the analysis methods used to identify and quantify dose distribution techniques to minimize errors that may result from patient setup, beam modeling, beam profile, and output that may affect the gamma index (5,55). Evaluation of planar dose distribution with gamma index in the IMRT technique is based on preselected dose difference (DD) and distance to agreement (DTA) criteria (54). The DTA is a measure of alignment between the dose distribution (in the high and low dose gradient region) and the dose difference histogram. A good alignment indicates that the difference between the two distributions is zero. On the other hand, DD is the percentage difference, implying that the two distributions are perfectly aligned. A predefined acceptance criterion can be used to compare the acceptability of a DD and DTA (56). Although the selection of DD, DTA, and the passing rate criteria may vary with one another, the passing percentage of gamma values ≤1 has been the standard method for determining if the two-dose distributions agree (54).
To develop virtual IMRT QA using machine learning models, patient QA data must first be gathered and accessed, in order to extract all parameters from plan files, including features for calculating all complexity metrics that affect passing rates. The fluence map for each plan is fed into the CNN, which is a form of neural network designed to evaluate images without the use of expert-made features. The machine models are then trained to forecast IMRT QA gamma passing rates with the aid of TensorFlow and Keras (57). Valdes et al. mention in their 2016 work that they want to build a virtual IMRT QA where planners can forecast the gamma indices of a plan before executing the QA, potentially preventing overmodulated plans and saving time. This is especially true for head and neck treatment programs, which are more complicated than other anatomical regions. The authors started by using roughly 500 Eclipse-based treatment plans (Varian) to train a generalized linear model with Poisson regression and LASSO regularization. Subsequently, the model was supplemented with portal dosimetry values from a separate institution and updated their 500-plan model with a CNN termed VGG-16 in their most recent article. The CNN and the Poisson-regression model produced similar results, but the CNN had numerous advantages over the Poisson model, including calculation speed (after model training) and independence from user-selected parameters (58). In addition, QA based on deep learning models predicts gamma passing rate using input training data such as PTV, rectum, and overlapping region, as well as the monitor unit for each field (57).
The MC approach is another valuable QA analysis tool for calculating radiation therapy doses by utilizing phase-space information (i.e., particle type, energy, charge, rotational, and spatial distributions) of beam particles. There have been reports of significant discrepancies in dosage distributions between MC estimates and treatment plans created using traditional dose calculation algorithms. However, the MC technique has the potential to replace conventional-dose calculation because of its ability to properly forecast dose distributions for complex beam delivery systems and heterogeneous patient anatomy. Currently, several commercial radiotherapy treatment planning systems are utilizing the MC approach because of its ability to achieve the maximum accuracy in radiotherapy dose computation (59,60). Three steps are often used in MC Treatment Planning; i.e., (I) calculating phase-space data after the primary set of Linac collimators, which is a machine calculation but not patient-specific; (II) calculating the phase-space data after the secondary or MLCs, which define the radiation field for a given treatment; and (III) simulation of the patient-specific CT geometry, where the dose-planning distribution is computed (61). The phase space data is calculated by simulating the path of each individual ionizing particle (photons or electrons) across the volume of interest. The particle may interact with the substance it passes through along the route, such as through Compton scattering (for photons) or Coulomb scattering (for electrons) (62). The particles are transported until they reach a user-defined energy cut-off (e.g., 0.01 MeV for photons and 0.6 MeV for electrons). The quantity of interest, such as the deposited energy in each voxel, can be determined using a large number of simulations (13). The program samples the distance l to the ‘next’ interaction for a particle at a particular place and with velocity vector v in a specific direction using a random number generator and probability distributions for the various types of interactions. The particle is then transmitted over the distance l to the interaction point with velocity v. The program then selects the type of interaction that will occur. The dose is the quantity of energy deposited per unit of mass (in SI units, J/kg = Gy). As a result, the MC code determines the energy balance for each simulated interaction by calculating the energy of the ‘incoming’ particle(s) minus the energy of the ‘outgoing’ one(s). The dose in a certain volume is determined as the ratio of the contributions from all interactions occurring inside the volume and the mass in the volume (62). However, AI trained on MC-derived data has the ability to replicate dose distributions that are almost identical to the MC approach while being much faster to deploy (17).
Challenges with AI in radiotherapy
Although AI has a lot of potential in radiation therapy, a significant number of challenges may prevent its full adoption in the clinical setting. Firstly, AI research, especially deep learning techniques requires a large number of quality data sets. Deep learning algorithms are tasked with creating classifiers and designing the best features from raw data. This is especially critical when human professionals, such as computer vision experts, are unable to develop adequate characteristics or quantify a given process. The small amount of the datasets available in radiation oncology is a significant restriction on using deep learning for segmentation. Deep learning models have millions of parameters and hence require more data than typical machine learning algorithms because the system is tasked with finding both the features and the classifier (50). Ideally, each data collected should be divided into three subsets (i.e., training, validation, and test data). The model should be trained using both training and validation data, with the validation data being utilized to tweak the model during training. Cross-validation can be used to do model training. After model validation, the test data should be utilized to evaluate the model’s performance. The test data set is sometimes not used and the validation findings are given as the research endpoint when using restricted size of data for automatic treatment planning (ATP), which can lead to overestimation of model performance or modeling error (36). The data augmentation approach, which has the potential to increase the usable number of data by adding affine image transformations such as translations, rotations, and scaling to the original image sets during the data training for auto segmentation, could overcome the challenges with limited data. Alternatively, model learning could also use high-level handmade features that reflect statistics from small data sets to correct the modeling error. This strategy, however, necessitates a deep understanding of AI algorithms at a low hierarchical level, which might be difficult for those without a strong computer science background (36). In situations where deep learning has been successful, tens of thousands of observations were used to train the models (50).
Secondly, AI ATP procedure can be somewhat complicated, especially when there are a lot of subsequent tasks. This can make the computation of ATP simulation process expensive (36) and deep learning models difficult to interpret. It is critical to have a thorough grasp of the reason for the plan generation process before deploying a model to automate the treatment planning process for patients. A model’s interpretability is required to ensure its generality and resilience over a wide range of patients. Lack of interpretability could result in unanticipated model failure during clinical deployment, putting patients at risk (63). By imposing simple principles during ATP, researchers can limit the complexity. Manual treatment planning is a common practice, and it follows rules based on a variety of factors, including machine hardware restrictions, radiological-based clinical preferences, and institutional practice guidelines. The ATP workflow complexity can be reduced by integrating these rules by correcting related variables. More crucially, developing a realistic ATP process for AI training necessitates collaboration among medical physicists, radiation oncologists, radiation dosimetrists, radiation therapists, and other personnel engaged in the creation and verification of radiotherapy plans (36).
The third challenge that may limit the advancement of AI technology in radiation therapy has to do with ethical and legal concerns. The majority of AI research necessitates the utilization of huge healthcare datasets, including imaging and clinical data. This raises legal and ethical concerns about who “owns” the data and who has the right to use it, especially when it has a commercial value. Although patient consent for data use would be desirable, given the vast number of patients in large datasets, especially in retrospective settings, this may not be feasible (46). AI systems are therefore created based on existing data in order to learn and draw conclusions. However, data sources may contain some bias (e.g., gender, sexual orientation, environmental, or economic characteristics), which can affect AI models trained on these data. Physicians in a certain clinical investigation ignored AI’s positive outcomes because the model’s positive predictive value was poor (3). This could be one of the reasons why some physicians, radiologists, and other health professionals regard AI models as a black box because they cannot fully verify and trust the algorithm (64). Besides, there are currently no globally consistent laws or regulations governing the use of AI in medicine to standardize practitioners’ behavior. Clinicians are totally responsible for the outcome of the patient’s diagnostic strategy, regardless of whether the AI system aided the strategy partially or completely. As a result, those who are unfamiliar with the inner workings of AI algorithms prefer to entrust complex clinical choices to a trustworthy ethical person (3). In a study by Wang et al., it was discovered that in complex scenarios, experienced planners outperform AP systems. In this sense, AP will be beneficial in reducing the workload from easy situations and allowing human planners to spend more time on hard issues, thus enhancing plan quality even more (36). One good step in dealing with AI-related ethical issues is when researchers cultivate the habit of sharing AI algorithms and data from a research study with other academics or practitioners so that they may independently validate and compare them to similar algorithms. This could ensure reproducibility, transparency, and generalizability of AI technology in radiotherapy (65). It is also necessary to establish clear legal and ethical frameworks for the use of AI in radiation oncology, including input from all stakeholders (i.e., patients, hospitals, research institutes, government, legislation, and so on) (46).
The evolving role of the medical physicist in radiation therapy
A qualified medical physicist must have a role to play in the evaluation of current technologies and the incorporation of new inventions into clinical practice (66). Considering the benefits of AI technologies in segmentation, quantification, diagnosis, and most importantly QA in radiation therapy, who should be responsible when an AI system fails? (24). Medical physicists have often been at the helm of integrating AI with radiotherapy practice. With little input from the medical physicist in the radiotherapy process, AI can aid in knowledge-based treatment planning in its implementation. Machine learning algorithms are trained on a dataset that includes patient images, contours, clinical information, and treatment plans performed by an experienced medical physicist to automatically develop high-quality plans, allowing for faster radiotherapy plan design (67).
Medical physicists can use both machine and deep learning-based approaches to better identify IMRT QA measurement problems and establish proactive QA strategies (68). During the implementation of QA procedures using AI technologies, challenges that emerge are appropriately addressed by medical physicists (28). For instance, when AI tools predict a failure in a LINAC machine, a medical physicist can assist in identifying the reason for a machine failure and take corrective steps, such as calibrations or quality control tests. In addition, the dose predicted by deep learning algorithms is validated by the medical physicist who performs QA using correctly designed in-phantom film/ion chamber measurements and comparing against previously published dose calculation techniques (67) or verifies the planned dose against the delivered dose (8).
AI image registration methods are susceptible to artifacts, which can reduce the accuracy of segmentation and dose distribution during treatment planning and delivery. When such problems are discovered, the medical physicist works with other members of the team, such as physicians, therapists, and dosimetrists, to come up with a clinically acceptable remedy. In such circumstances, further manual modifications are usually required in order to achieve an acceptable clinical registration outcome. In a radiotherapy center without a dosimetrist, these modifications are performed by the medical physicist who designs the dose distribution and makes the necessary adjustments on a trial-and-error basis in order to obtain the desired prescribed dose (8,67,69).
The concept of interaction of radiation with matter (i.e., the human body in this case) is not quite simple due to the many complex underlining theories associated with the process (70,71); this situation, therefore, requires expert medical physics knowledge. For this reason, dose computation, beam configuration, treatment source calibration, and dose delivery were previously handled by qualified medical physicists who acquired comprehensive knowledge in radiation interaction with matter at master and/or Ph.D. levels (2). As a result, it would be a challenge for radiotherapy procedures to be implemented on the back of AI technologies successfully without the input of a medical physicist. Therefore, it is recommendable for all academic institutions offering medical physics training to ensure that AI content is incorporated into the curriculum for training and education of trainee medical physicists (72), in order to harmonize and promote medical physics best practices in line with emerging advances in their practice (1).
At the moment, all the AI technologies developed by academic researchers when migrated into clinical practice would eventually be evaluated by clinical medical physicists. This is because, to adopt new technologies, more QA is needed at the beginning. QA of an AI system would be quite difficult, and it would necessitate the involvement of many physicists (28). The medical physicist must approach AI in the same way they have approached previous technological advancements: with cautious optimism and a desire to participate (69).
The application of AI in radiotherapy practice would not necessarily reduce or completely replace medical physicists but would rather assist them to spend less time during treatment planning, plan to check, and machine QA procedures. Medical physicists are needed to carefully perform an extensive check on treatment plans generated by AI technologies (28). After treatment plans have been completed and approved, the medical physicist performs plan checks and other QA checks to ensure that the performance of the radiation delivery machine is optimal and delivers the exact dose to the patient. The presence of a medical physicist to oversee the operation of these automated devices and the patient will continue to be vital.
Conclusions
AI has the capacity to outline complicated structures, produce autonomous treatment plans and ideal doses, and monitor patients’ treatment responses with accuracy comparable to the level of accuracy in manual procedures, but in a fraction of the time. However, more QA is required to implement new AI technologies. This is because the QA of an AI system can be very demanding and requires the participation of many clinical medical physicists. As a result, academic institutions that train medical physicists must incorporate AI content into their curricula, and radiotherapy equipment manufacturers must involve qualified medical physicists in the incorporation of AI technologies into their equipment.
Acknowledgments
Funding: None.
Footnote
Reporting Checklist: The authors have completed the Narrative Review reporting checklist. Available at https://jmai.amegroups.com/article/view/10.21037/jmai-22-27/rc
Peer Review File: Available at https://jmai.amegroups.com/article/view/10.21037/jmai-22-27/prf
Conflicts of Interest: All authors have completed the ICMJE uniform disclosure form (available at https://jmai.amegroups.com/article/view/10.21037/jmai-22-27/coif). The authors have no conflicts of interest to declare.
Ethical Statement: The authors are accountable for all aspects of the work in ensuring that questions related to the accuracy or integrity of any part of the work are appropriately investigated and resolved.
Open Access Statement: This is an Open Access article distributed in accordance with the Creative Commons Attribution-NonCommercial-NoDerivs 4.0 International License (CC BY-NC-ND 4.0), which permits the non-commercial replication and distribution of the article with the strict proviso that no changes or edits are made and the original work is properly cited (including links to both the formal publication through the relevant DOI and the license). See: https://creativecommons.org/licenses/by-nc-nd/4.0/.
References
- Eudaldo T, Huizenga H, Lamm IL, et al. Guidelines for education and training of medical physicists in radiotherapy. Recommendations from an ESTRO/EFOMP working group. Radiother Oncol 2004;70:125-35. [Crossref] [PubMed]
- Malicki J. Medical physics in radiotherapy: The importance of preserving clinical responsibilities and expanding the profession's role in research, education, and quality control. Rep Pract Oncol Radiother 2015;20:161-9. [Crossref] [PubMed]
- Jiang L, Wu Z, Xu X, et al. Opportunities and challenges of artificial intelligence in the medical field: current application, emerging problems, and problem-solving strategies. J Int Med Res 2021;49:3000605211000157. [Crossref] [PubMed]
- El Naqa I, Haider MA, Giger ML, et al. Artificial Intelligence: reshaping the practice of radiological sciences in the 21st century. Br J Radiol 2020;93:20190855. [Crossref] [PubMed]
- Vandewinckele L, Claessens M, Dinkla A, et al. Overview of artificial intelligence-based applications in radiotherapy: Recommendations for implementation and quality assurance. Radiother Oncol 2020;153:55-66. [Crossref] [PubMed]
- Chamunyonga C, Edwards C, Caldwell P, et al. The Impact of Artificial Intelligence and Machine Learning in Radiation Therapy: Considerations for Future Curriculum Enhancement. J Med Imaging Radiat Sci 2020;51:214-20. [Crossref] [PubMed]
- Sheng K. Artificial intelligence in radiotherapy: a technological review. Front Med 2020;14:431-49. [Crossref] [PubMed]
- Huynh E, Hosny A, Guthier C, et al. Artificial intelligence in radiation oncology. Nat Rev Clin Oncol 2020;17:771-81. [Crossref] [PubMed]
- Siddique S, Chow JCL. Artificial intelligence in radiotherapy. Rep Pract Oncol Radiother 2020;25:656-66. [Crossref] [PubMed]
- Li X, Tian D, Li W, et al. Artificial intelligence-assisted reduction in patients' waiting time for outpatient process: a retrospective cohort study. BMC Health Serv Res 2021;21:237. [Crossref] [PubMed]
- Santoro M, Strolin S, Paolani G, et al. Recent Applications of Artificial Intelligence in Radiotherapy: Where We Are and Beyond. Applied Sciences 2022;12:3223. [Crossref]
- Yakar M, Etiz DU. Artificial Intelligence in Radiation Oncology. Artif Intell Med Imaging 2021;2:13-31. [Crossref]
- Chen X, Diaz-Pinto A, Ravikumar N, et al. Deep learning in medical image registration. Progress in Biomedical Engineering 2021;3:012003.
- Fu Y, Lei Y, Wang T, et al. Deep learning in medical image registration: a review. Phys Med Biol 2020;65:20TR01. [Crossref] [PubMed]
- Islam KT, Wijewickrema S, O'Leary S. A deep learning based framework for the registration of three dimensional multi-modal medical images of the head. Sci Rep 2021;11:1860. [Crossref] [PubMed]
- Zou M, Hu J, Zhang H, et al. Rigid medical image registration using learning-based interest points and features. Computers, Materials and Continua. 2019;60:511-25. [Crossref]
- Liao R, Miao S, de Tournemire P, et al. An artificial agent for robust image registration. Proceedings of the AAAI Conference on Artificial Intelligence 2017;31: [Crossref]
- Boveiri HR, Khayami R, Javidan R, et al. Medical image registration using deep neural networks: a comprehensive review. Computers & Electrical Engineering 2020;87:106767. [Crossref]
- Lei Y, Harms J, Wang T, et al. MRI-only based synthetic CT generation using dense cycle consistent generative adversarial networks. Med Phys 2019;46:3565-81. [Crossref] [PubMed]
- Boulanger M, Nunes JC, Chourak H, et al. Deep learning methods to generate synthetic CT from MRI in radiotherapy: A literature review. Phys Med 2021;89:265-81. [Crossref] [PubMed]
- Guerreiro F, Koivula L, Seravalli E, et al. Feasibility of MRI-only photon and proton dose calculations for pediatric patients with abdominal tumors. Phys Med Biol 2019;64:055010. [Crossref] [PubMed]
- Han X. MR-based synthetic CT generation using a deep convolutional neural network method. Med Phys 2017;44:1408-19. [Crossref] [PubMed]
- Li J, Erdt M, Janoos F, et al. Medical image segmentation in oral-maxillofacial surgery. Computer-Aided Oral and Maxillofacial Surgery 2021:1-27
- Arabi H, Zaidi H. Applications of artificial intelligence and deep learning in molecular imaging and radiotherapy. Eur J Hybrid Imaging 2020;4:17. [Crossref] [PubMed]
- Tang X. The role of artificial intelligence in medical imaging research. BJR Open 2019;2:20190031. [Crossref] [PubMed]
- Yoon HJ, Jeong YJ, Kang H, et al. Medical image analysis using artificial intelligence. Progress in Medical Physics 2019;30:49-58. [Crossref]
- Liang G, Fan W, Luo H, et al. The emerging roles of artificial intelligence in cancer drug development and precision therapy. Biomed Pharmacother 2020;128:110255. [Crossref] [PubMed]
- Tang X, Wang B, Rong Y. Artificial intelligence will reduce the need for clinical medical physicists. J Appl Clin Med Phys 2018;19:6-9. [Crossref] [PubMed]
- Dhont J, Harden SV, Chee LYS, et al. Image-guided Radiotherapy to Manage Respiratory Motion: Lung and Liver. Clin Oncol (R Coll Radiol) 2020;32:792-804. [Crossref] [PubMed]
- Zhao W, Shen L, Islam MT, et al. Artificial intelligence in image-guided radiotherapy: a review of treatment target localization. Quant Imaging Med Surg 2021;11:4881-94. [Crossref] [PubMed]
- Zhou D, Nakamura M, Mukumoto N, et al. Development of AI-driven prediction models to realize real-time tumor tracking during radiotherapy. Radiat Oncol 2022;17:42. [Crossref] [PubMed]
- Lin H, Zou W, Li T, et al. A Super-Learner Model for Tumor Motion Prediction and Management in Radiation Therapy: Development and Feasibility Evaluation. Sci Rep 2019;9:14868. [Crossref] [PubMed]
- Kostovski A. Artificial Intelligence in Radiotherapy. Radiološki vjesnik: radiologija, radioterapija, nuklearna medicina 2021;25:37-40.
- Engberg L. Automated radiation therapy treatment planning by increased accuracy of optimization tools (Doctoral dissertation, KTH Royal Institute of Technology). Accessed May 24, 2022. Published 2018. Available online: https://www.diva-portal.org/smash/record.jsf?pid=diva2%3A1258306&dswid=-5326
- Xia X, Wang J, Li Y, et al. An Artificial Intelligence-Based Full-Process Solution for Radiotherapy: A Proof of Concept Study on Rectal Cancer. Front Oncol 2021;10:616721. [Crossref] [PubMed]
- Wang C, Zhu X, Hong JC, et al. Artificial Intelligence in Radiotherapy Treatment Planning: Present and Future. Technol Cancer Res Treat 2019;18:1533033819873922. [Crossref] [PubMed]
- Xu H, Lu J, Wang J, et al. Implement a knowledge-based automated dose volume histogram prediction module in Pinnacle3 treatment planning system for plan quality assurance and guidance. J Appl Clin Med Phys 2019;20:134-40. [Crossref] [PubMed]
- Cao W, Zhuang Y, Chen L, et al. Application of dose-volume histogram prediction in biologically related models for nasopharyngeal carcinomas treatment planning. Radiat Oncol 2020;15:216. [Crossref] [PubMed]
- Sutherland K. An Interactive Discussion on the Role of Knowledge-Based Planning in the Canadian Radiation Therapy Department. Journal of Medical Imaging and Radiation Sciences 2014;45:187. [Crossref]
- Momin S, Fu Y, Lei Y, et al. Knowledge‐based radiation treatment planning: A data‐driven method survey. Journal of Applied Clinical Medical Physics 2021;22:16-44. [Crossref] [PubMed]
- Zhong Y, Yu L, Zhao J, et al. Clinical Implementation of Automated Treatment Planning for Rectum Intensity-Modulated Radiotherapy Using Voxel-Based Dose Prediction and Post-Optimization Strategies. Front Oncol 2021;11:697995. [Crossref] [PubMed]
- PALO A. Varian Medical Systems Receives FDA 510(k) Clearance for RapidPlan™ Knowledge-Based Treatment Planning Software for Radiation Oncology. Publised October 2013. Accessed May 20, 2022. Available online: https://www.prnewswire.com/news-releases/varian-medical-systems-receives-fda-510k-clearance-for-rapidplan-knowledge-based-treatment-planning-software-for-radiation-oncology-228634081.html
- Wang W, Sheng Y, Wang C, et al. Fluence Map Prediction Using Deep Learning Models - Direct Plan Generation for Pancreas Stereotactic Body Radiation Therapy. Front Artif Intell 2020;3:68. [Crossref] [PubMed]
- Zhang Y, Merritt M. Fluence map optimization in IMRT cancer treatment planning and a geometric approach. In: Multiscale Optimization Methods and Applications. Boston: Springer, 2006:205-27.
- Ma L, Chen M, Gu X, et al. Deep learning-based inverse mapping for fluence map prediction. Phys Med Biol 2020; [Crossref] [PubMed]
- Lee H, Kim H, Kwak J, et al. Fluence-map generation for prostate intensity-modulated radiotherapy planning using a deep-neural-network. Sci Rep 2019;9:15671. [Crossref] [PubMed]
- Wu J. System and method for automated fluence map prediction and radiation treatment plan generation. Accessed May 25, 2022. Published 2021. Available online: https://otc.duke.edu/technologies/system-and-method-for-automated-fluence-map-prediction-and-radiation-treatment-plan-generation/
- Mission Search. Artificial Intelligence and Radiotherapy – Part One. Accessed May 24, 2022. Published May 26, 2021. Available online: https://missionsearch.com/artificial-intelligence-and-radiotherapy/
- Chaix B, Bibault JE, Pienkowski A, et al. When Chatbots Meet Patients: One-Year Prospective Study of Conversations Between Patients With Breast Cancer and a Chatbot. JMIR Cancer 2019;5:e12856. [Crossref] [PubMed]
- Feng M, Valdes G, Dixit N, et al. Machine Learning in Radiation Oncology: Opportunities, Requirements, and Needs. Front Oncol 2018;8:110. [Crossref] [PubMed]
- Simon L, Robert C, Meyer P. Artificial intelligence for quality assurance in radiotherapy. Cancer Radiother 2021;25:623-6. [Crossref] [PubMed]
- Nyflot MJ, Thammasorn P, Wootton LS, et al. Deep learning for patient-specific quality assurance: Identifying errors in radiotherapy delivery by radiomic analysis of gamma images with convolutional neural networks. Med Phys 2019;46:456-64. [Crossref] [PubMed]
- El Naqa I, Irrer J, Ritter TA, et al. Machine learning for automated quality assurance in radiotherapy: A proof of principle using EPID data description. Med Phys 2019;46:1914-21. [Crossref] [PubMed]
- Li H, Dong L, Zhang L, et al. Toward a better understanding of the gamma index: Investigation of parameters with a surface-based distance method. Med Phys 2011;38:6730-41. [Crossref] [PubMed]
- Park JM, Choi CH, Wu HG, et al. Correlation of the gamma passing rates with the differences in the dose-volumetric parameters between the original VMAT plans and actual deliveries of the VMAT plans. PLoS One 2020;15:e0244690. [Crossref] [PubMed]
- Inhwan JY, Yong OK. A Procedural Guide to Film Dosimetry, with Emphasis on IMRT. Chapter Four: Measurement and Analysis. Medical Physics Publishing, 2004:3-7.
- Chan MF, Witztum A, Valdes G. Integration of AI and Machine Learning in Radiotherapy QA. Front Artif Intell 2020;3:577620. [Crossref] [PubMed]
- Valdes G, Scheuermann R, Hung CY, et al. A mathematical framework for virtual IMRT QA using machine learning. Med Phys 2016;43:4323. [Crossref] [PubMed]
- Ma CM, Li JS, Pawlicki T, et al. A Monte Carlo dose calculation tool for radiotherapy treatment planning. Phys Med Biol 2002;47:1671-89. [Crossref] [PubMed]
- Ma CMC, Chetty IJ, Deng J, et al. Beam modeling and beam model commissioning for Monte Carlo dose calculation-based radiation therapy treatment planning: Report of AAPM Task Group 157. Med Phys 2020;47:e1-e18. [Crossref] [PubMed]
- Andreo P. Monte Carlo simulations in radiotherapy dosimetry. Radiat Oncol 2018;13:121. [Crossref] [PubMed]
- Reynaert N, Van der Marck S, Schaart D, et al. Monte Carlo treatment planning: an introduction. Nederlandse Commissie Voor Stralingsdosimetrie. 2006. Accesed on 9th May, 2022. Available online: https://radiationdosimetry.org/files/documents/0000015/68-ncs-rapport-16-monte-carlo-treatment-planning.pdf
- Stiglic G, Kocbek P, Fijacko N, et al. Interpretability of machine learning‐based prediction models in healthcare. Wiley Interdisciplinary Reviews: Data Mining and Knowledge Discovery 2020;10:e1379. [Crossref]
- Parkinson C, Matthams C, Foley K, et al. Artificial intelligence in radiation oncology: A review of its current status and potential application for the radiotherapy workforce. Radiography (Lond) 2021;27:S63-8. [Crossref] [PubMed]
- Park SH, Kim YH, Lee JY, et al. Ethical challenges regarding artificial intelligence in medicine from the perspective of scientific editing and peer review. Science Editing 2019;6:91-8. [Crossref]
- Clements JB, Baird CT, de Boer SF, et al. AAPM medical physics practice guideline 10.a.: Scope of practice for clinical medical physics. J Appl Clin Med Phys 2018;19:11-25. [Crossref] [PubMed]
- Avanzo M, Trianni A, Botta F, et al. Artificial intelligence and the medical physicist: welcome to the machine. Applied Sciences 2021;11:1691. [Crossref]
- Lam D, Zhang X, Li H, et al. Predicting gamma passing rates for portal dosimetry-based IMRT QA using machine learning. Med Phys 2019;46:4666-75. [Crossref] [PubMed]
- Murphy A, Liszewski B. Artificial Intelligence and the Medical Radiation Profession: How Our Advocacy Must Inform Future Practice. J Med Imaging Radiat Sci 2019;50:S15-9. [Crossref] [PubMed]
- Adlienė D. Radiation interaction with condensed matter. Applications of Ionizing Radiation in Materials Processing 2017;1:33-54.
- Bichsel H, Schindler H. The Interaction of Radiation with Matter. Particle Physics Reference Library 2020:5-44.
- Andersson J, Nyholm T, Ceberg C, et al. Artificial intelligence and the medical physics profession - A Swedish perspective. Phys Med 2021;88:218-25. [Crossref] [PubMed]
Cite this article as: Manson EN, Mumuni AN, Fiagbedzi EW, Shirazu I, Sulemana H. A narrative review on radiotherapy practice in the era of artificial intelligence: how relevant is the medical physicist? J Med Artif Intell 2022;5:13.