Implementing artificial intelligence in clinical practice: a mixed-method study of barriers and facilitators
Highlight box
Key findings
• The current tension for change in healthcare is insufficient to facilitate AI implementation. Implementation strategies are needed to facilitate sustainable adoption.
What is known and what is new?
• A wide variety of AI algorithms have been created for the healthcare industry, but few make it into clinical practice. Barriers and facilitators in this process are ill-defined.
• This study revealed barriers and facilitators healthcare professionals may experience with AI (implementation) and suggests implementation strategies.
What is the implication, and what should change now?
• The introduction of AI tools in practice should systematically be supported by various implementation strategies, such as increasing knowledge and information through local leaders, using a trial phase to let users test and compare AI algorithms, and tailoring the tools to the local context and existing workflows.
Introduction
The start of the Information Age in the mid-20th century has been a catalyst for the use of data in medicine (1). The early days were characterized by limited datasets, created to answer specific questions, but developments were accelerated with the widespread introduction of monitoring devices and Electronic Health Record (EHR) systems around the turn of the century (2). This enhanced labeled big-data, together with increased computing power and cloud storage, boosted the use of artificial intelligence (AI) in medicine (3). Today’s healthcare professionals often feel overwhelmed by vast amounts of data from various sources (4). As medicine enters the Age of AI, there is great potential for algorithms to help make sense of all the data and augment clinical decision-making (3,5). Algorithms can use data points from large numbers of patients to detect subtle patterns that healthcare professionals may overlook (6). These insights can support the clinical assessment of a patient, decrease diagnostic uncertainty, and improve the overall quality of care. However, medicine has been slow to adopt AI tools. Up until 2020, only 222 AI tools were approved by the US Food & Drug Administration (FDA) and 240 in Europe (of which 124 in both) (7).
The low number of approved medical AI tools is surprising considering the fact that over 50,000 studies of clinical AI model development were available in through MEDLINE alone as of October 2022, according to an interactive dashboard (8). There seems to be a significant gap between the development and deployment of AI in the healthcare industry (6). Recent reviews have shown that about 95% of the published studies on AI only address the development of a particular algorithm (9,10). In comparison, only 1–2% of those studies evaluate the use of the algorithms against clinically relevant outcomes, and few are integrated in practice. It has been suggested that a key factor of the poor implementation of AI algorithms is the lack of inclusivity and engagement of the end-users and their domain knowledge during the development of these tools (11).
Barriers and facilitators to clinical AI implementation among healthcare professionals are ill defined, nor have they been linked to appropriate implementation strategies to overcome them. The Consolidated Framework for Implementation Research (CFIR) is a tool to identify these types of contextual influences and explain the strikingly low implementation rates of medical AI (12). Furthermore, the barriers identified by the CFIR can be entered into the Expert Recommendations for Implementing Change (ERIC) tool for implementation strategies, to create a well-tailored approach (13).
In the current study, we applied the CFIR framework to identify barriers and facilitators to AI implementation in the clinical practice. We aim to find general insights that could be applicable to a wide variety of AI-tool implementations, so that this information can be used to facilitate implementation of future AI tools in medical practice, and realize their potential to improve patient care. We present the following article in accordance with the COREQ reporting checklist (available at https://jmai.amegroups.com/article/view/10.21037/jmai-22-71/rc).
Methods
We conducted a mixed-methods study consisting of three inclusion phases: individual interviews, a focus group discussion, and a nationwide survey. The Amsterdam University Medical Centers’ (UMC) local medical ethics review committee waived the review of this study as the Medical Research involving Human Subjects Act did not apply (IRB number: IRB00002991; case: 2021.0396). All participants provided informed consent. The study was conducted in accordance with the Declaration of Helsinki (as revised in 2013).
Inclusion phase I—individual interviews
The first phase of this study encompassed semi-structured individual interviews with the end-users (physicians) of potential AI algorithms in healthcare, regarding barriers and facilitators to clinical AI implementation. We designed a topic list for the interview, based on CFIR literature and expertise of the research team (physicians and a psychologist) (12). The topic list was structured as an hourglass: started broadly with questions and prompts on AI in healthcare in general. Then the topic narrowed down to a clinical case vignette about an AI blood culture tool to provide physicians with specific details, questions and prompts. This AI tool was recently developed by our research group and it predicts the outcomes of blood cultures in the emergency department, which may help avoid unnecessary testing and associated harmful effects (14). During the time of interview, focus group and survey (and to date) the blood culture tool was not implemented in clinical practice. We included this tool as clinical case vignette to provide interviewed physicians with real examples from a real project, and enhancing discussions. The topic list ended with AI in healthcare in the broad sense. The topic list can be consulted in Appendix 1 (translated, English). The first few interviews were used to pilot the topic list, and we then concluded that the list was comprehensive, hence no additions or revisions were made to the initial list.
We included physicians from the emergency, internal medicine (including infectious disease specialists), and microbiology departments of our Amsterdam UMC hospitals (location VUmc and AMC). We chose to target these specific specialties as they will be the main end-users of our developed AI algorithm, and they represent a substantial percentage of all hospital physicians (15). Physicians of all experience levels were eligible for inclusion, to gain a broad and generally applicable picture.
Physicians were recruited through the department secretariats with an invitational email. When interested in participation, a research team member provided additional information regarding the study, and scheduled a date for the interview. Interviews were conducted face-to-face at one of the two hospital locations, in a private room (either a meeting room, or a personal workspace). All interviews were conducted by two researchers: a female psychologist (BS) and a male medical doctor (MS). Both interviewers had interview experience, gained through previous qualitative research projects and their education. Interviewed physicians were not close colleagues of the interviewers, nor was there a prior (work) relationship. The duration of the interviews was approximately 45 minutes. Interviews were recorded with an audio recorder and field notes were taken during interviews.
We aimed to include until data saturation for this phase was deemed to be reached. The inclusion of the individual interviews ran between August 2021 and September 2021.
Inclusion phase II—focus group
To complement the information that emerged from the individual interviews, the second phase of this study encompassed a focus group. The expected complementary value of the focus group was that it enables and stimulates interaction and discussion, which could therefore provide additional information. By performing both individual interviews and a focus group, we have the information on the topic that people are willing to share both ‘publicly’ and ‘privately’ (16). For this phase we used equal inclusion- criteria and processes as for the individual interviews. The topic list used in the focus group was identical to the individual interviews. The physicians included in the interviews were not allowed to participate in the focus group. The focus group discussion was led by an experienced focus group interviewer (MvB), and supported by BS and MS. There was no prior relationship between MvB and the focus group participants. Due to COVID-19 restrictions, the focus group was conducted online and audio recorded through Microsoft Teams. The focus group took place in September 2021 and took 90 minutes.
Inclusion phase III—nationwide survey
Based on the themes identified in phase I and II, we created a quantitative survey (Appendix 2). The aim of this survey was to rank the most prominent barriers and facilitators from the interviews, and identify those endorsed by a large population of potential AI end-users. To keep the survey concise, we only incorporated questions on important topics identified in the interviews. A power calculation for the number of survey participants was irrelevant since we did not plan to perform any statistical tests. However, we aimed to include at least 100 survey participants, to ensure we had a variety of medical specialists of different ages. The survey thus ran between December 2021 and February 2022. An anonymous link was distributed to practitioners across the country through hospital secretariats, medical associations, and social media.
Data collection and privacy
Audio recordings of the interviews and focus group were transcribed. Transcripts or study results were not returned to participants for review and feedback. Audio recordings and transcripts were stored digitally at the Amsterdam UMC location VUmc. Characteristics data on the participants were stored in a separate file, and not included in the audio files nor transcripts. All data materials could only be accessed by the local study researchers.
As for the survey, no directly identifiable data were collected, and participants could stay completely anonymous.
Statistical analysis
Qualitative data analysis
We performed deductive direct content analysis (16), using the CFIR (12) to code the interviews and focus group transcripts. Transcripts were coded independently by both BS and MS. After two independently coded transcripts, an interim consensus procedure followed, to ensure inter-coder agreement. After which both coders continued independently coding all transcripts, followed by an extensive consensus process, leading up to the final codes. Coding was performed in MaxQDA 2022 (VERBI Software, 2021).
To match the barriers to implementation of AI algorithms in healthcare to implementation strategies, we used the ERIC tool (13).
Quantitative data analysis
The survey was conducted using Phase Zero (Phase Zero Software, 2021). The cohort of participants was described using means and medians when appropriate. The answers to the survey questions were reported using counts and percentages. Some questions were not answered by all participants. The number of answers and total number of responses are presented with all results.
Results
We first conducted ten individual interviews with physicians, and one focus group with five physicians. Interim analyses showed data saturation was reached after these interviews and the focus group, as no novel information emerged. Table 1 reports demographic characteristics per interview- and focus group participant. The total sample consisted of 15 physicians, 33% of whom were female, with a median age of 40 (IQR, 34–45). The most prominent constructs identified during the qualitative interim analyses were incorporated in the nationwide survey. The demographic characteristics of the 106 survey respondents are presented in Table 2. Most respondents were aged between 31 and 40 (49%), they had a median of 9 years of experience (IQR, 3–17), and were mostly associated with Internal Medicine (77%).
Table 1
Participant No. | Age, years | Sex | Specialty | Experience level |
---|---|---|---|---|
Interview | ||||
1 | 33 | Female | Internal Medicine | Resident |
2 | 43 | Female | Internal Medicine | Specialist |
3 | 31 | Female | Microbiology | Researcher |
4 | 37 | Male | Emergency Medicine | Specialist |
5 | 60 | Male | Internal Medicine | Specialist |
6 | 43 | Female | Internal Medicine | Specialist |
7 | 33 | Male | Emergency Medicine | Specialist |
8 | 35 | Male | Microbiology | Resident |
9 | 38 | Male | Internal Medicine | Resident |
10 | 28 | Male | Intensive Care | Resident |
Focus group | ||||
1 | 47 | Male | Internal Medicine | Specialist |
2 | 54 | Male | Internal Medicine | Specialist |
3 | 51 | Male | Microbiology | Specialist |
4 | 40 | Female | Internal Medicine | Specialist |
5 | 43 | Male | Emergency Medicine | Specialist |
Table 2
Characteristic | Value |
---|---|
Age, years, n (%) | |
18–25 | 2 (1.9) |
26–30 | 16 (15.1) |
31–35 | 26 (24.5) |
36–40 | 26 (24.5) |
41–45 | 15 (14.2) |
46–50 | 5 (4.7) |
51–55 | 6 (5.7) |
56–60 | 5 (4.7) |
61–65 | 5 (4.7) |
Specialty, n (%) | |
Internal Medicine | 82 (77.4) |
Microbiology | 15 (14.2) |
Intensive Care | 3 (2.8) |
Emergency Medicine | 3 (2.8) |
Orthopedics | 1 (0.9) |
Pulmonology | 1 (0.9) |
Other | 1 (0.9) |
Experience with AI*, n (%) | |
None | 25 (23.6) |
Clinical use | 17 (16.0) |
Research use | 18 (17.0) |
Research development | 22 (20.8) |
Personal interest | 42 (39.6) |
Use in daily life | 44 (41.5) |
Clinical experience, years, median [IQR] | 9 [3–17] |
*, total exceeds 100% since multiple answers were possible (except when “None” was selected). Totals per question may not add up to the total of 106 participants, as some questions were not answered by all participants. AI, artificial intelligence; IQR, interquartile range.
Figure 1 shows a word cloud of CFIR constructs, coded based on the interview and focus group data (minimum frequency of 2). In the following section we will elaborate on the relevant CFIR constructs. Some CFIR constructs were rarely or never coded. Therefore, they were deemed irrelevant to the topic and are not included in the results. For constructs which were also addressed in the survey, the qualitative data were enriched by the corresponding quantitative data.
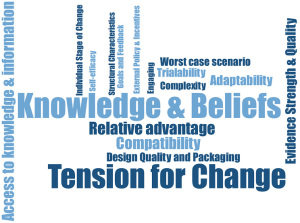
Intervention characteristics—evidence strength
Interviewed physicians found evidence strength an important facilitator, although they did not agree on the type of evidence that would be sufficient. Some voiced that comprehensive retrospective and prospective validation of an algorithm would be sufficient, while others wouldn’t settle for less than a high impact intervention study: “conduct a rigorous study and publish in [high impact journal] that will be adopted worldwide.”—physician in interview 7, hereafter I7. All interviewed physicians agreed that an internationally published RCT indicating the AI tool X led to better outcomes for patient category Y would provide the best evidence and could facilitate AI implementation by enhancing trust: “a randomized study would be a good result. That way you’ll have to accept that AI has added value.”—I4, and: “building trust in an AI algorithm has to be based on scientific research.”—I5.
There was no consensus regarding which study outcomes should be pursued in such a trial to best facilitate implementation. Some interviewed physicians considered cost-effectiveness and/or process optimization valuable outcomes, whereas others would only be willing to deploy AI if evidence shows benefits on patient outcomes.
To study the potential benefits of AI that would be considered valuable by a large population of physicians, we asked the survey participants to rank the following topics according to importance: cost-effectiveness, work process optimization, and patient outcomes. Surveyed physicians most often ranked these items in the following order of importance: patient outcomes, work process optimization, and cost-effectiveness (56/105; 53.3%). Some found costs to be more important than work processes, ranking them: patient outcomes, cost-effectiveness, and work process optimization (25/105; 23.8%). Among those who selected a ranking in which patient outcomes were not the most important potential benefit, the most common selection was: work process optimization, patient outcomes, and cost-effectiveness (17/105; 16.2%).
Intervention characteristics—relative advantage
To evaluate the potential benefits of AI, many interviewed physicians compared AI to their current practice. Often, AI was compared to the brain of a medical specialist, and put forward as both an influential facilitator and barrier. Some interviewed physicians argued that healthcare professionals base their decision making on certain aspects which are hard to capture in algorithms: “the computer has not seen that patient, and I have.”—I1, and: “The gut feeling [that physicians have], (…) is intangible and not easily measurable. You cannot put that in an algorithm.”—I2. They see this as barrier for implementing AI, as they do not believe AI has relative advantage over experienced healthcare professionals. However, for the less experienced physicians, AI was thought to be beneficial: “old-school physicians are like encyclopedias, they see a patient and instantly know what is wrong. (..) Less experienced physicians do not have the benefit of this pattern recognition due to less experience. For them, AI can be useful.”—focus group.
Relative advantage can also be a facilitator, as some interviewed physicians voiced that the ability to analyze complex data, endless opportunities for combining data, and the speed that AI is capable of, could never be reached by the human brain. For example: “[AI] establishes links that we cannot establish. The computer associates observations, and is less dependent on cause-effect. So when we as physicians cannot consider a logical solution because of this dependency, the model can actually draw logical conclusions.”—focus group, and: “we figure things out after three hours, while AI could figure it out within the first hour.”—I4.
Intervention characteristics—adaptability
The adaptability of an AI algorithm has two important aspects for the interviewed physicians. An algorithm has to be adaptable to their patient population (regarding predictive performance), and be easy to integrate with existing workflows.
Currently, adaptability is a barrier as many interviewed physicians tend to believe an algorithm would not be applicable to their patients, even when it is validated in their specific patient population. A few examples: “I would be stubborn, (…) and think: this is not applicable to my patient.”—focus group, “you would worry about this, even though you know [the algorithm] has really been researched in this population.”—focus group, and: “I need to know how my patients fits into the picture.”—I1.
Intervention characteristics—trialability
An important facilitator for sustainable implementation of AI is trialability. All interviewed physicians argued that having a trial-and-error phase to get used to an algorithm, and compare the outcomes of an algorithm to their own clinical decision making, would enhance trust. An example from I10: “Look, everybody is talking about AUC’s and how well [their algorithm] performs etc. But, in the end of the day, that does not show me what it adds in clinical practice. It needs to be implemented, it needs to be tangible. You need to see for yourself what it adds. (…) You need time to learn to trust the algorithm.”. Another example is: “I want to see an algorithm work in practice. To be able to work with it, and know exactly how it operates. I need to build trust. (…) If we can try an algorithm for a while, we can quickly see the benefits”—I4.
Similar results were found during the survey. When asked whether the surveyed physicians would trust an algorithm more if they would first be able to use it next to their own judgement, 76/106 (71.7%) agreed or strongly agreed, while just 18/106 (17.0%) disagreed or strongly disagreed.
Inner setting—structural characteristics
Structural characteristics (e.g., the social architecture or maturity of an organization) also play a role during the implementation of AI algorithms in healthcare. Although all interviewed physicians believed AI has some value in healthcare, they were not unanimously convinced that the traditional character of hospitals in general is well suited to its implementation in the short term: “hospital care is highly conservative. We as physicians are highly conservative. So, I don’t know whether [implementation of AI] will go fast to be honest.”—I9.
Inner setting—tension for change
Tension for change, either positive or negative, was one of the most influential constructs on implementation of AI algorithms in healthcare. Some interviewed physicians did not feel the need for AI algorithms, because they rather continue working in the status quo. They explain that they learned certain ways of diagnosing/treating patients during medical education, which has become a behavioural habit that is hard to deviate from: “it is habit. We are used to doing things a certain way. It is hard to learn something new. It has to do with trust also. Because you trust the things you already do, but if you need to implement something new you need time in order to trust such an algorithm.”—I1. Another example was voiced by the physician in I10 about the blood culture algorithm: “I could definitely accept that prediction, because 1% is a low chance so then we won’t perform the blood culture because that would be unnecessary care. But, it is so deeply rooted in our workflow, so actually I would still just perform the blood culture.”.
However, many interviewed physicians did see potential value of using in AI algorithms in healthcare: “there sure is room for improvement [in ED diagnostics], AI could play a very important role in this.”—I4, and: “modern physicians need to be open to the idea that computers might perform better than they do (…). We only have experience of a couple of years, while the computer could have experience of thousands of human years.”—I5.
For the interviewed physicians, the potential benefits of AI in healthcare lie within process optimization, time-efficiency, cost-efficiency, and enhanced patient-centeredness, patient safety, and quality of care, as voiced by I7: “for example at the ED, but also at multiple wards. The shortage in nurses is a substantial problem, AI could help with this. But also with regard to timeliness of processes, efficiency, and maybe costs. For example, if you would perform less blood cultures, that would help. That is of course a small example, but you could broaden the scope. I think [AI] could have value in many different areas.”. Another example is: “you could see more patients: perform the same work with less staff. That way you can spend more time on each patient, that is very important. Patients currently get way too little time with physicians. Especially on the wards, they lie in bed for 24 hours and the physician comes to see them for 5 minutes. That is too little. While the patient and their families have many questions, and need for conversation. If we would gain more time for these things, that would be golden.”—I9.
Since the qualitative results showed that interviewed physicians can feel tension for change, but that this could be both a barrier and a facilitator, we asked the survey participants specifically how they felt about our blood culture prediction tool. In 81/104 (77.9%) answers, the surveyed physicians agreed or strongly agreed that the use of our AI algorithm for blood culture indication could add value, while just 7/104 (6.7%) disagreed or strongly disagreed.
Inner setting—compatibility
Another influential construct is compatibility. Interviewed physicians found it important that AI algorithms are implemented to support their clinical decision making, while they keep their autonomy (to overrule an algorithm) and the responsibility for their patients. In other words, AI algorithms need to be compatible to existing workflows and decision-making and enhance/support this, as opposed to taking them over completely. For example: “in the end the physician or nurse decides what they adopt from the algorithm. But keeping that autonomy is very important.”—I10, “I think [AI] can be of value and support us, but we shouldn’t just blindly trust it.”-I1, and: “[AI] should become some sort of advice, and not an obligation. Because, well, advices are there to sometimes not be followed.”—I7.
All interviewed physicians believed that the responsibility for patient outcomes is always that of the physician, regardless of whether they followed or deviated from the algorithm: “the physician has the final responsibility. For example when it comes to a medical disciplinary court, the computer is not the one to get the reprimand.”—I5.
Inner setting—access to knowledge and information
To enable trust in AI algorithms and facilitate proper implementation, the interviewed physicians felt they need access to knowledge and information about the algorithm. More specifically, they voiced the need to be informed regarding: the overall evidence, the data used (which patient populations and variables), the validation, how it operates, and how this translates to their clinical practice. This is particularly evident for highly complex algorithms. Examples are: “I would like to receive some sort of package: what is the rationale, what are the studies, which datasets is it developed and trained in, what is the aim.”—I6, and: “of course AI can sometimes be challenging to explain. So you have to take end-users by the hand. I think it is most important that we understand how the model is functioning in practice.”—I3. Another example is periodic feedback information to end-users: “to show results and effects of the AI based decisions periodically. This way we can create trust in the algorithm.”—I3. In addition, some interviewed physicians felt that they would sooner use decision support provided by algorithms when they understand how the predictions are made. An example is: “But if I would understand why it would predict a certain outcome, then I would be more inclined to consider whether I would or would not use it.”—I1.
In the survey, we followed-up with this frequently mentioned construct and asked how surveyed physicians would like to be informed about future AI algorithms. There was a clear preference for information integrated in the existing workflow (41/106; 38.7%) [e.g., in the EHR system where the algorithms is implemented), or frequent reminders and presentations during handover moments and teaching sessions (41/106; 38.7%)]. Documentation stored in separate systems, or training periods prior to implementation were less favoured with 11/106 (10.4%) positive answers each. When asked specifically how the participants would prefer AI algorithm outputs to be presented, there was no clear preference for absolute risk percentages (31/106; 29.2%), binary suggestions to take or not take a certain action (37/106; 34.9%), or risk categories (38/106; 35.8%).
Characteristics of the individual—knowledge and beliefs about the innovation
Knowledge and beliefs about AI algorithms in healthcare was also one of the most influential constructs. There was a wide variation of attitudes, values, familiarity with facts, etc. related to AI algorithms both between and within individual interviewed physicians. Even though there was this wide variation, many interviewed physicians voiced to have little to no prior experience with AI, especially not in their clinical work: “Maybe there are algorithms that play a role in my life. But I don’t use them myself. No, not at all.”—I9.
During the nationwide survey, 25 out of 106 (23.6%) surveyed physicians shared the belief that they had never come into contact with an AI algorithm, either in their work or outside.
Worst case scenarios
To identify out of the box barriers, additional to the CFIR constructs, we asked interviewed physicians about potential worst case scenarios in the clinical use of AI. Interviewed physicians mostly worry about adverse outcomes for patients, i.e., delayed or wrong diagnosis, suboptimal treatment, inappropriate discharge, or even death. The physician in interview 4 voiced: “harm to the patient. Like missing an important diagnosis, that has major negative outcomes for the patient. This could turn into a complication or even adverse event.”.
Other worst case scenarios were regarding the professional stature of physicians. This includes that deployment of AI could lead to losing their job altogether, lose the enjoyable aspects of the job, or to become a ‘lazy’ physician. For example: “if all you have to do is follow the [AI] model you don’t have to go to medical school. Then you will just sit behind your desk and approve everything [the AI model predicts]. That would be completely worthless. At least for the physician. Although maybe it would be better for patient care.” (I10).
The survey results do not fully match the findings from the qualitative part of the study in this instance. When we asked surveyed physicians whether they were worried that AI would take over the enjoyable and interesting parts of job, 74/105 (70.5%) disagreed or strongly disagreed, while just 14/105 (13.3%) agreed.
Implementation strategies—ERIC tool
We used the CFIR to identify potential barriers to implementation of AI algorithms in healthcare, and then linked these barriers to implementation strategies using the ERIC tool. We included all nine CFIR constructs that are described above, leading to a top 3 of the following implementation strategies: identify and prepare champions (cumulative percentage: 280%); conduct educational meetings (258%); promote adaptability (235%). Two other important strategies are: develop educational materials (153%) and distribute educational materials (149%), due to their high individual endorsement percentages. For the total output of ERIC strategies, see Appendix 3.
Discussion
This study identified barriers and facilitators to AI implementation in clinical practice. Through individual interviews and a focus group with end-users (physicians), we found nine CFIR constructs important to AI implementation: evidence strength, relative advantage, adaptability, trialability, structural characteristics, tension for change, compatibility, access to knowledge and information, and knowledge and beliefs about the intervention (12). When linking these constructs to implementation strategies using the ERIC tool, we found that the following strategies should be used for AI implementation: identify and prepare champions, conduct educational meetings, promote adaptability, develop educational materials, and distribute educational materials (13).
AI has the potential to change medicine through its ability to augment clinical decision-making by detecting subtle patterns in vast amounts of patient data, and do so tirelessly for 24 hours a day. To reach this potential, physicians need to see the relative advantage of integrating AI in their current practice, and feel a tension for change. In general, physicians acknowledge the potential value of AI in healthcare, which is a facilitator for implementation. However, physicians in our study expressed that current behavioural habits and standard practices are hard to deviate from. To create new norms and behaviour, we need to go beyond sole awareness creation (17). As highlighted by the ERIC tool, local champions are the key to success in this process. One needs committed local leaders to inspire and actively remind others to use a specific AI tool. Lasting change and sustainable implementation of AI can then be achieved through several key CFIR constructs. Firstly, evidence strength is important, because physicians view a peer-reviewed and internationally published article as a facilitator to AI implementation. As described in the introduction section, there are many published papers on AI algorithms, but these are neither implemented nor deployed in clinical practice (10). This suggests that an international publication in itself is not sufficient for sustainable implementation. In addition, access to knowledge and information about the algorithm is essential. This was one of the most prominent CFIR constructs in our study. Access to knowledge and information about an AI algorithm can be realized through the following ERIC implementation strategies: conduct educational meetings, develop educational materials, and distribute educational materials, which can be expedited by local champions. Hence, to provide physicians with digestible information regarding an AI algorithm, it is important to develop a toolkit with manuals and other supporting material, to distribute these toolkits, and explain and educate further in meetings (13). Our data shows that physicians favour the following information in their toolkit: overall evidence, the data used, the validation, how it operates, and how this translates into clinical benefits. Both qualitative and survey data display that physicians prefer their source of knowledge and information integrated in the existing workflow, e.g., EHR system. This is in line with the CFIR construct adaptability, and ERIC strategy promote adaptability (18). Moreover, integrating AI in the EHR will promote sustainable implementation (19,20). Besides adapting AI to existing workflows, it is necessary to provide physicians with information regarding how well an AI algorithm is adapted to their patient population. We found that physicians tend to expect that a certain algorithm does not apply to ‘their’ patient, even when the algorithm has been validated in similar patients. This could be due to frequency bias in physicians, leading to the belief that the frequency of patients that will fall within the small margin of error of the algorithm (and therefore lead to a wrong prediction) is much higher than it actually is. Moreover, even though evidence-based medicine is considered the gold standard in clinical reasoning, the review by Nicolini et al. show the importance of local -context and knowledge for physicians when making clinical decisions, and how this is usually valued more than evidence from research (21). This could be overcome by access to knowledge and information, and trialability (22). Trust is another important dynamic in the interaction between AI and end-users, which has been well-studied in the literature. Factors such as explainability, transparency, and interpretability seem to be key to facilitate adoption (23). Our study further adds that physicians need a trial-and-error phase in implementation to experience these factors themselves, which has been described before (22). Besides increased trust, this will allow physicians to gain expertise with the algorithm, experience clinical benefits for patients, and will help further refinement and adaptation (24). Trialability also fits within the cycle of ‘plan-do-study-act’, which is a tool widely used in healthcare for quality improvement (25). The broad range of knowledge and beliefs regarding AI underpins the importance of trialability and access to knowledge and information. Lastly, compatibility emerged as a primary construct which may form a barrier to AI implementation. When end-users view an intervention as threat to their autonomy, it is less likely that implementation will be successful (12). In our study, physicians feel strongly about retaining their autonomy. They argue that they should always have the final responsibility over the patients, even when AI algorithms influence their decision making. Therefore, it is important for physicians to be able to deliberately deviate from the AI recommendations, like they can do with general clinical guidelines.
The results of this study should be interpreted in the light some limitations. Firstly, the interviewees and participants of the survey were mostly physicians from the Internal Medicine, Emergency Medicine, and Microbiology departments. However, we still feel these results are generalizable to a broader group of physicians, since most questions were not specialty dependent, and some of the themes we found have been described in other cohorts before (22). Still, it would be helpful to tailor any implementation of an AI tool to the local context and end-users, for which additional surveys and interviews in those settings are needed to confirm the generalizability of our results. Secondly, it could be possible that the survey was subject to self-selection bias, i.e., the physicians who chose to respond to the survey might have differed from the group of physicians that chose not to respond. Lastly, in our survey we included n=106 participants. We did not perform a-priori power calculation, as it was not feasible to make assumptions about effect sizes due to the novelty of the studied subject. It is therefore challenging to make a statement regarding the representativeness of our sample size. However, we do believe that this sample size is sufficient to ensure a range of variety in the participants.
Conclusions
The healthcare industry has been slow to adopt AI algorithms. We identified several widely endorsed constructs important to AI in healthcare and linked them to appropriate implementation strategies. Though the potential value of AI in healthcare is acknowledged by end-users (physicians), the current tension for change is insufficient to facilitate implementation and adoption. The tension for change can be sparked by conducting educational meetings, and developing and distributing educational materials to increase access to knowledge and information. Committed local leaders are indispensable to expedite this process. Moreover, a trial phase in which physicians can test the AI algorithms and compare them to their own judgement, may further support implementation. Finally, AI developers should try and tailor their algorithms to be both adaptable and compatible with the values and existing workflows of the users. As physicians have the final responsibility for the patient, they should be able to overrule any decision of the algorithm and keep their autonomy. Applying these appropriate implementation strategies will bring us one step closer to realizing the value of AI in healthcare.
Acknowledgments
We would like to thank all the healthcare professionals who participated in this study for their time and enthusiasm.
Funding: This work was supported through the 2021 innovation grant of Amsterdam Public Heath, Quality of Care program: Quality of Care Research Program (amsterdamumc.org).
Footnote
Reporting Checklist: The authors have completed the COREQ reporting checklist. Available at https://jmai.amegroups.com/article/view/10.21037/jmai-22-71/rc
Data Sharing Statement: Available at https://jmai.amegroups.com/article/view/10.21037/jmai-22-71/dss
Peer Review File: Available at https://jmai.amegroups.com/article/view/10.21037/jmai-22-71/prf
Conflicts of Interest: All authors have completed the ICMJE uniform disclosure form (available at https://jmai.amegroups.com/article/view/10.21037/jmai-22-71/coif). The authors have no conflicts of interest to declare.
Ethical Statement: The authors are accountable for all aspects of the work in ensuring that questions related to the accuracy of integrity of any part of the work are appropriately investigated and resolved. The study was conducted in accordance with the Declaration of Helsinki (as revised in 2013). The Amsterdam University Medical Centers’ (UMC) local medical ethics review committee waived the review of this study as the Medical Research involving Human Subjects Act did not apply (IRB number: IRB00002991; case: 2021.0396). All participants signed written informed consent.
Open Access Statement: This is an Open Access article distributed in accordance with the Creative Commons Attribution-NonCommercial-NoDerivs 4.0 International License (CC BY-NC-ND 4.0), which permits the non-commercial replication and distribution of the article with the strict proviso that no changes or edits are made and the original work is properly cited (including links to both the formal publication through the relevant DOI and the license). See: https://creativecommons.org/licenses/by-nc-nd/4.0/.
References
- Wartman SA, Combs CD. Medical Education Must Move From the Information Age to the Age of Artificial Intelligence. Acad Med 2018;93:1107-9. [Crossref] [PubMed]
- Brown N. Healthcare Data Growth - An Exponential Problem 2015. Available online: https://www.nextech.com/blog/healthcare-data-growth-an-exponential-problem
- Topol EJ. High-performance medicine: the convergence of human and artificial intelligence. Nat Med 2019;25:44-56. [Crossref] [PubMed]
- Paranjape K, Schinkel M, Nanayakkara P. Short Keynote Paper: Mainstreaming Personalized Healthcare-Transforming Healthcare Through New Era of Artificial Intelligence. IEEE J Biomed Health Inform 2020;24:1860-3. [Crossref] [PubMed]
- Paranjape K, Schinkel M, Nannan Panday R, et al. Introducing Artificial Intelligence Training in Medical Education. JMIR Med Educ 2019;5:e16048. [Crossref] [PubMed]
- Schinkel M, Paranjape K, Nannan Panday RS, et al. Clinical applications of artificial intelligence in sepsis: A narrative review. Comput Biol Med 2019;115:103488. [Crossref] [PubMed]
- Muehlematter UJ, Daniore P, Vokinger KN. Approval of artificial intelligence and machine learning-based medical devices in the USA and Europe (2015-20): a comparative analysis. Lancet Digit Health 2021;3:e195-203. [Crossref] [PubMed]
- Zhang J, Whebell S, Gallifant J, et al. An interactive dashboard to track themes, development maturity, and global equity in clinical artificial intelligence research. Lancet Digit Health 2022;4:e212-3. [Crossref] [PubMed]
- Fleuren LM, Thoral P, Shillan D, et al. Machine learning in intensive care medicine: ready for take-off? Intensive Care Med 2020;46:1486-8. [Crossref] [PubMed]
- van de Sande D, van Genderen ME, Huiskens J, et al. Moving from bytes to bedside: a systematic review on the use of artificial intelligence in the intensive care unit. Intensive Care Med 2021;47:750-60. [Crossref] [PubMed]
- Verma AA, Murray J, Greiner R, et al. Implementing machine learning in medicine. CMAJ 2021;193:E1351-7. [Crossref] [PubMed]
- Damschroder LJ, Aron DC, Keith RE, et al. Fostering implementation of health services research findings into practice: a consolidated framework for advancing implementation science. Implement Sci 2009;4:50. [Crossref] [PubMed]
- Powell BJ, Waltz TJ, Chinman MJ, et al. A refined compilation of implementation strategies: results from the Expert Recommendations for Implementing Change (ERIC) project. Implement Sci 2015;10:21. [Crossref] [PubMed]
- Schinkel M, Boerman AW, Bennis FC, et al. Diagnostic stewardship for blood cultures in the emergency department: A multicenter validation and prospective evaluation of a machine learning prediction tool. EBioMedicine 2022;82:104176. [Crossref] [PubMed]
- Boerman AW, Schinkel M, Meijerink L, et al. Using machine learning to predict blood culture outcomes in the emergency department: a single-centre, retrospective, observational study. BMJ Open 2022;12:e053332. [Crossref] [PubMed]
- Green J TN. Qualitative Methods for Health Research: SAGE Publications Inc; 2018.
- Gawande A. Slow Ideas. The New Yorker, 2013 July 29.
- Mendel P, Meredith LS, Schoenbaum M, et al. Interventions in organizational and community context: a framework for building evidence on dissemination and implementation in health services research. Adm Policy Ment Health 2008;35:21-37. [Crossref] [PubMed]
- Catho G, Centemero NS, Waldispühl Suter B, et al. How to Develop and Implement a Computerized Decision Support System Integrated for Antimicrobial Stewardship? Experiences From Two Swiss Hospital Systems. Front Digit Health 2021;2:583390. [Crossref] [PubMed]
- Sim I, Gorman P, Greenes RA, et al. Clinical decision support systems for the practice of evidence-based medicine. J Am Med Inform Assoc 2001;8:527-34. [Crossref] [PubMed]
- Nicolini D, Powell J, Conville P, et al. Managing knowledge in the healthcare sector. A review. Int J Manag Rev 2008;10:245-63. [Crossref]
- Paranjape K, Schinkel M, Hammer RD, et al. The Value of Artificial Intelligence in Laboratory Medicine. Am J Clin Pathol 2021;155:823-31. [Crossref] [PubMed]
- Tucci V, Saary J, Doyle TE. Factors influencing trust in medical artificial intelligence for healthcare professionals: a narrative review. J Med Artif Intell 2022;5:4. [Crossref]
- Feldstein AC, Glasgow RE. A practical, robust implementation and sustainability model (PRISM) for integrating research findings into practice. Jt Comm J Qual Patient Saf 2008;34:228-43. [Crossref] [PubMed]
- Taylor MJ, McNicholas C, Nicolay C, et al. Systematic review of the application of the plan-do-study-act method to improve quality in healthcare. BMJ Qual Saf 2014;23:290-8. [Crossref] [PubMed]
Cite this article as: Schouten B, Schinkel M, Boerman AW, van Pijkeren P, Thodé M, van Beneden M, Nannan Panday R, de Jonge R, Wiersinga WJ, Nanayakkara PWB. Implementing artificial intelligence in clinical practice: a mixed-method study of barriers and facilitators. J Med Artif Intell 2022;5:12.