Comparison of predicted survival curves and personalized prognosis among cox regression and machine learning approaches in glioblastoma
Highlight box
Key findings
• The multilayer perceptron model had the highest value of Harrell's concordance index, while the random survival forest model had the lowest root mean square error.
What is known and what is new?
• Cox hazard regression has been used to predict prognosis of patients with glioblastoma.
• Time-to-event machine learning (ML) algorithms have role of prognostication, particularly multilayer perceptron, and random survival forest.
What is the implication, and what should change now?
• Time-to-event ML algorithms could be utilized to provide personalized prognosis and therapy recommendations.
Introduction
Glioblastoma is the most common malignant tumor and the most aggressive primary brain tumor in adults (1,2). While advances in the treatment of the disease have been extensively researched, the disease’s prognosis has remained poor. Maximum safe surgical resection, postoperative radiation, and temozolomide (TMZ) chemotherapy are the current gold standards for the treatment of this disease (3). Moreover, O-6-methylguanine-DNA methyltransferase (MGMT) promoter methylation has been the key molecular factor that could predict TMZ sensitivity (4). From previous studies, patients with the methylated MGMT promoter had significantly more survival benefits than the unmethylated MGMT promoter group that were treated with TMZ (5-8).
Although TMZ is the standard treatment following tumor resection, the cost-effectiveness of this chemotherapy has been debated in several countries (9-12). Wu et al. studied the economic outcome of the treatment strategies for glioblastoma patients and found that TMZ was not a cost-effective treatment choice in health resource-limited settings (13). It has also been suggested to utilize individualized prognosis prediction to determine each patient’s treatment plan that could be one of the resource allocation methods (14,15).
At present, machine learning (ML) has been used for diagnosis and prognostication in various fields, particularly neuro-oncology (16-19). Priya et al. distinguished glioblastoma from brain metastasis using ML methods (16), whereas Tunthanathip et al. used ML-based algorithms to predict a 2-year survival in glioblastoma patients and reported that the area under the receiver operating characteristic curve (AUC) was 0.81–0.82 (17). From the literature review, the binary classification of ML is commonly used for predicting prognosis at a certain time point, such as 2-year survival. However, Katzman et al. used time-to-event ML algorithms for prognostication, while only a few studies in the literature review used time-to-event ML (20).
Because personalized prognostication is visualized as a survival curve for each patient by the time-to-event ML algorithms that have been challenged, it may assist the physician in deciding on an individual treatment approach as a concept of personalized medicine (17,20). Therefore, the present study aimed to compare the predictive performances among Cox hazard regression, parametric survival regression, and time-to-event ML algorithms. The secondary objective was to deploy a personalized survival curve for each patient’s condition. In addition, we present this article in accordance with the TRIPOD reporting checklist of prediction model development and validation (available at https://jmai.amegroups.com/article/view/10.21037/jmai-22-98/rc).
Methods
Study designs and study population
The study design of the present study was a retrospective cohort study. All newly diagnosed glioblastoma patients were hospitalized between December 1, 2007 and June 30, 2021 in a tertiary center in Southern Thailand. According to the 2021 World Health Organization’s central nervous system tumor classification (21), glioblastoma was diagnosed with histological findings found in microvascular proliferation or necrosis by pathologists or wild-type isocitrate dehydrogenase (IDH) gene (21,22). Hence, patients who lacked histological slides to confirm the diagnosis, comprehensive medical records, and the ability to assess an updated prognosis were excluded. For the data collection, computerized medical records were reviewed and obtained. Additionally, neurosurgeons reviewed the preoperative and postoperative imaging of individuals with glioblastoma.
Molecular analysis
As previously disclosed, the mutation of IDH and methylation of the MGMT promoter were studied following DNA extraction from the tumor specimens (23). Specifically, methylated MGMT was defined as having at least 30% methylation (24).
Statistical analysis
The demographics of the present cohort were explained using descriptive statistics. In detail, the categorical variables were reported as percentages, and the mean and standard deviation (SD) were utilized for the continuous variables. In addition, several continuous variables were separated into categorical variables based on cut-off point from average value.
Survival outcomes were analyzed in the present study as follows: overall survival, survival probability, and median survival time. In detail, the time from surgery until death was referred to as overall survival that was shown by the Kaplan-Meier curves, whereas the proportion of units that survive beyond a specified time was referred to as the survival probability. Furthermore, the median survival time was defined as the length of time from the date of surgery that half of a group of glioblastoma patients were still alive (21-23).
Time-to-event was performed for estimating the prognosis and the overall survival with the survival probability in each time-point was analyzed on October 31, 2022. In both the univariate and multivariable analyses, the prognostic variables were found using the Cox hazard regression with complete case analysis. In detail, the backward stepwise procedure was performed to select the potential predictors for the final predictive model that candidate risk factors with P<0.10 from the univariate regression analysis were entered into the multivariable regression model. As such, the Cox model assumption estimated that a patient’s variables were combined linearly to form the log risk of failure for that patient, which was known as the linear proportional hazards condition. P<0.05 was accepted as being statistically significant. Moreover, the prognostic nomogram was developed for patients with glioblastoma was performed by R version 4.0.5 (The R Foundation for Statistical Computing; Vienna, Austria).
In addition, parametric survival models of the time-to-event analysis were conducted for compared the performances with the Cox regression in two distinct distributions: Gompertz and Weibull. For hypothesis testing, the AUC formula was used to calculate sample size (25). The following parameters were used for estimation in previous studies: AUC of 0.82, alpha of 0.05, and estimation error of 0.15. As a result, a sample size of 40 patients was required for validation.
ML for the time-to-event analysis
Using the same predictors as the Cox hazard regression, the following ML algorithms were performed for comparison with the Cox hazard regression: random survival forest (RSF), conditional survival forest (CSF), and multilayer perceptron (MLP). Training processes among various algorithms was performed with 5-fold cross-validation. Moreover, the optimum value of Harrell’s concordance index (C-index) was used to evaluate the hyperparameter tuning of ML models by the manual tuning method. In detail, the number of trees and node size of the leaf node were turned for the RSF and CSF models. For the MLP model, the activation function, optimizer, and number of hidden layers were turned for the best value of C-index. The ML was performed using the Python program version 3.8.7 with the PySurvival package (26) (Python Software Foundation, USA).
Time-to-event performance metrics
The whole dataset was randomly split into a training dataset and a testing dataset with a 70:30 split. The training dataset was used to build the predictive model development by the Cox hazard regression, parametric survival model, and ML methods, while the testing dataset was used for performance validation. Therefore, the performances of the Cox hazard regression, parametric survival, and other ML models were compared using the following criteria: C-index, Brier score, and root mean square error (RMSE) (26).
Harrell’s C-index was used for the model’s predictive accuracy (27,28). The C-index is a measure of a model’s ability to forecast the sequence of patients’ deaths. Additionally, a C-index of 0.5 is the average for a random model, while a C-index of 1 is the best way to rank the times of death (27,28).
Brier score was used to estimate the accuracy of the predicted survival function with the censoring times among the models. Brier score of the model was below 0.25 that was preferred as the useful model, while the RMSE was used to compare the predicted and actual number of patients at risk over time among the various predictive models (26).
Additionally, Cox hazard regression, parametric survival models, and ML algorithms were used to build the Kaplan-Meier curves for showing the overall prognosis and creating personalized survival curves for each condition (28,29).
Ethical statement
The study was conducted in accordance with the Declaration of Helsinki (as revised in 2013). The study was approved by institutional ethics committee board of the Faculty of Medicine, Prince of Songkla University (No. REC 63-372-10-1). Due to the nature of the retrospective study design, patients were not required to provide informed consent. However, before analysis, patient identification numbers were encoded.
Results
A total of 212 patients were initially diagnosed with glioblastoma. Two patients lacked a histopathologically proven diagnosis, and two patients’ medical records were incomplete. Hence, two hundred and eight patients were included for the analysis, as shown in Figure 1. Their demographic data are shown in Table 1. The mean age of patients was 50.79 years (SD =16.02) and more than half of the present cohort was male. Additionally, a preoperative Karnofsky Performance Status score of more than 70 was observed at 51.4%.
Table 1
Factor | Total | Trains | Test | P value |
---|---|---|---|---|
Age (years) | 0.79 | |||
<50 | 96 (46.2) | 67 (45.6) | 29 (47.5) | |
>50 | 112 (53.8) | 80 (54.4) | 32 (52.5) | |
Mean age [SD], years | 50.79 [16.02] | 50.07 [15.10] | 52.51 [18.06] | 0.35 |
Gender | 0.83 | |||
Male | 117 (56.3) | 82 (55.8) | 35 (57.4) | |
Female | 91 (43.8) | 65 (44.2) | 26 (42.6) | |
Frontal lobe | 74 (35.6) | 45 (30.6) | 29 (47.5) | 0.03 |
Temporal lobe | 71 (34.1) | 50 (34.0) | 21 (34.4) | 0.95 |
Parietal lobe | 39 (18.8) | 33 (22.4) | 6 (9.8) | 0.04 |
Occipital lobe | 10 (4.8) | 9 (6.1) | 1 (1.6) | 0.16 |
Corpus callosum tumor | 26 (12.7) | 15 (10.3) | 11 (18.3) | 0.11 |
Preoperative KPS | 0.46 | |||
<70 | 101 (48.6) | 69 (46.9) | 32 (52.5) | |
≥70 | 107 (51.4) | 78 (53.1) | 29 (47.5) | |
Mean preoperative size (cm), mean [SD] | 5.37 [1.66] | 5.19 [1.66] | 5.80 [1.59] | 0.01 |
Mean preoperative volume (cm3), mean [SD] | 55.23 [41.70] | 51.34 [40.30] | 64.61 [43.82] | 0.04 |
Maximum diameter (cm) | 0.01 | |||
<5 | 88 (42.3) | 71 (48.3) | 17 (27.9) | |
≥5 | 120 (57.7) | 76 (51.7) | 44 (72.1) | |
Tumor volume (mL) | 0.14 | |||
<50 | 115 (55.3) | 86 (58.5) | 29 (47.5) | |
≥50 | 93 (44.7) | 61 (41.5) | 32 (52.5) | |
Extent of resection | 0.10 | |||
Biopsy | 26 (12.5) | 14 (9.5) | 12 (19.7) | |
Partial resection | 127 (61.1) | 91 (61.9) | 36 (59.0) | |
Total resection | 55 (26.4) | 42 (28.6) | 13 (21.3) | |
Gross total resection | 0.28 | |||
Total resection | 55 (26.4) | 42 (28.6) | 13 (21.3) | |
Non-total resection | 153 (73.6) | 105 (71.4) | 48 (78.7) | |
Postoperative KPS | 0.44 | |||
<70 | 128 (61.5) | 88 (59.9) | 40 (65.6) | |
≥70 | 80 (38.5) | 59 (40.1) | 21 (34.4) | |
Adjuvant therapy | 0.85 | |||
Radiotherapy alone | 135 (64.9) | 96 (65.3) | 39 (63.9) | |
Concomitant TMZ | 73 (35.1) | 51 (34.7) | 22 (36.1) | |
MGMT promoter methylation | >0.99 | |||
Unmethylation | 7 (3.4) | 3 (2.0) | 4 (6.6) | |
Methylation | 201 (96.6) | 144 (98.0) | 57 (93.4) |
Data are shown as n (%) or mean [SD]. KPS, Karnofsky Performance Status; MGMT, O6-alkylguanine-DNA alkyltransferase; SD, standard deviation; TMZ, temozolomide.
From the preoperative radiologic findings, the mean size of the tumor was 5.37 cm (SD =1.66) and more than half of all tumors had a tumor diameter of more than 5 cm. Glioblastoma was commonly involved in the frontal lobe and temporal lobe, while corpus callosal glioblastoma was observed in 12.7% of the total cohort. A frameless stereotactic biopsy was performed in 12.5% of the total cohort, while 26.4% of all operations were total tumor resections. After the operation, 35.1% of all patients received concurrent chemotherapy with TMZ because TMZ use would depend on the patients’ medical welfare in Thailand (30). Moreover, 3.4% of all patients had unmethylated MGMT promoters. After 70:30 data splitting, almost no differences in baseline characteristics were observed between the train and testing datasets. Although being considerably different between the train and test datasets in terms of tumor location, tumor size, and tumor volume, these parameters were not associated with prognosis after multivariable analysis of Cox hazard regression analysis.
Survival analysis
The mean follow-up time of 14.2 months (SD =16.8) and 1-, 2-, and 5-year probabilities of survival in the present study were 18%, 12%, and 1%, respectively. In addition, the median survival time was 11.7 months [95% confidence interval (CI): 9.22–12.77].
Factors associated with the prognosis of glioblastoma
Using the Cox hazard regression, a frontal tumor, temporal tumor, gross total resection (GTR), concomitant TMZ, and methylation of MGMT promoter had a P value of less than 0.1, as shown in Table 2. These factors were analyzed using multivariable analysis, and the remaining three factors associated with the prognosis were as follows: GTR, concomitant TMZ, and methylation of MGMT promoter. The traditional nomogram was built for prognostication of patients with glioblastoma, as shown in Figure 2.
Table 2
Factors | Univariate analysis | Multivariable analysis | |||
---|---|---|---|---|---|
HR (95% CI) | P value | HR (95% CI) | P value | ||
Age, years | |||||
≤50 | Ref | ||||
>50 | 1.09 (0.81–1.45) | 0.55 | |||
Age, years | |||||
≤60 | Ref | ||||
>60 | 1.22 (0.87–1.71) | 0.23 | |||
Gender | |||||
Male | Ref | ||||
Female | 0.94 (0.70–1.25) | 0.67 | |||
Frontal lobe | 0.74 (0.54–1.005) | 0.054 | |||
Temporal lobe | 1.33 (0.98–1.81) | 0.06 | |||
Parietal lobe | 1.05 (0.72–1.52) | 0.79 | |||
Occipital lobe | 1.38 (0.72–2.61) | 0.32 | |||
Corpus callosum tumor | 1.19 (0.77–1.85) | 0.42 | |||
Preoperative KPS score | |||||
<70 | Ref | ||||
≥70 | 1.18 (0.88–1.57) | 0.26 | |||
Maximum diameter, cm | |||||
<5 | Ref | ||||
≥5 | 1.01 (0.76–1.36) | 0.89 | |||
Tumor volume, mL | |||||
<50 | Ref | ||||
≥50 | 0.89 (0.67–1.20) | ||||
Total resection | |||||
Total resection | Ref | Ref | |||
Non-total resection | 0.44 (0.31–0.62) | <0.001 | 0.20 (0.09–0.44) | <0.001 | |
Postoperative KPS-score | |||||
<70 | Ref | ||||
≥70 | 0.97 (0.72–1.31) | 0.86 | |||
Adjuvant therapy | |||||
Radiotherapy alone | Ref | Ref | |||
Concomitant TMZ | 0.57 (0.42–7.84) | <0.001 | 0.54 (0.39–0.73) | <0.001 | |
MGMT promoter methylation | |||||
Unmethylation | Ref | Ref | |||
Methylation | 0.17 (0.07–0.38) | <0.001 | 0.20 (0.09–0.44) | <0.001 |
CI, confidence interval; HR, hazard ratio; KPS, Karnofsky Performance Status; MGMT, O6-alkylguanine-DNA alkyltransferase; Ref, reference group; TMZ, temozolomide.
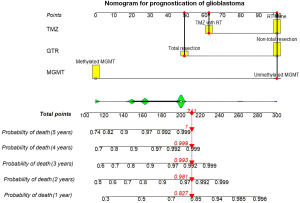
Comparison of the performance metrics among various methods
Following the data split, 146 patients were used for the development of the ML model, and the remaining 62 cases were used to assess the model’s performance. The shape of the Kaplan-Meier curves for training and testing the datasets was close, as shown in Figure 3A, while the Kaplan-Meier curves of other models were demonstrated in Figure 3B. The RSF and CSF had almost the same shape for the Kaplan-Meier curves from the Cox hazard regression.
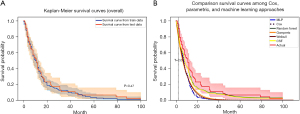
After hyperparameter turning, the setting of the RSF and CSF in the present study was 200 trees contained in the forest with 20 minimum node size of the leaf node, whereas the MLP structure comprised 1-hidden layer with 150 units and “Bent Identity” activation function. Therefore, the performances of the predictive models among various time-to-event methods were estimated by Harrell’s C-index, Brier score, and RMSE (Table 3). The predictive model of MLP had the highest value of Harrell’s C-index (P value of t-test <0.001), but the RSF model had the lowest RMSE and mean absolute error (P value of t-test =0.001 and 0.19, respectively).
Table 3
Approach | Harrell’s concordance index (95% CI) | Brier score (95% CI) | RMSE (95% CI) | Median absolute error (95% CI) | Mean absolute error (95% CI) |
---|---|---|---|---|---|
Cox | 0.648 (0.642–0.653) | 0.168 (0.165–0.172) | 1.006 (1.005–1.007) | 0.637 (0.635–0.638) | 0.855 (0.854–0.856) |
CSF | 0.640 (0.639–0.641) | 0.169 (0.166–0.171) | 1.032 (1.031–1.033) | 0.693 (0.691–0.696) | 0.859 (0.857–0.861) |
RSF | 0.640 (0.639–0.641) | 0.169 (0.168–0.170) | 0.980 (0.979–0.981) | 0.666 (0.664–0.667) | 0.815 (0.814–0.816) |
MLP | 0.659 (0.657–0.661) | 0.149 (0.146–0.151) | 1.001 (0.997–1.005) | 0.588 (0.587–0.589) | 0.848 (0.847–0.879) |
Gompertz | 0.649 (0.647–0.651) | 0.158 (0.155–0.162) | 1.572 (1.56–1.53) | 0.853 (0.851–0.856) | 0.954 (0.951–0.956) |
Weibull | 0.648 (0.644–0.652) | 0.158 (0.155–0.162) | 1.137 (1.136–1.138) | 0.769 (0.768–0.770) | 0.953 (0.952–0.955) |
ML, machine learning; CI, confidence interval; Cox, Cox proportional hazard regression; CSF, conditional survival forest; MLP, multilayer perceptron; RMSE, root mean square error; RSF, random survival forest.
A comparison of the predicted number of patients at risk over time among the various time-to-event approaches is demonstrated in Figure 4. The predicted graph of the Cox, CSF, RSF and MLP were close to the actual graph.
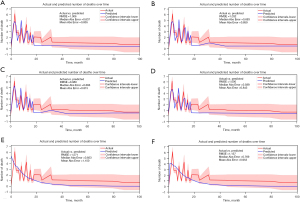
Personalized prognosis using the Kaplan-Meier curves
The prediction of the individual prognosis was performed according to the significant factors associated with the prognosis; therefore, eight possible conditions were estimated by the Kaplan-Meier curves (Figure 5). On ranking in the personalized prognosis, the poorest prognosis condition was patients with non-total resection, postoperative radiation alone, and an unmethylated MGMT promoter, while patients with total resection, postoperative radiation with TMZ, and methylated MGMT promoter had the longest survival time using the Cox hazard regression.
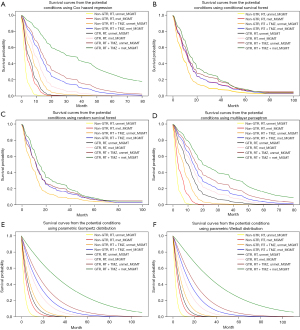
The rank of the survival curves by the MLP and parametric survival models were in concordance with the personalized survival curves by the Cox hazard regression, while several lines of personalized survival curves by the RSF and CSF models overlapped.
Discussion
The prognosis of glioblastoma was poor in the present study, and these findings were consistent with those of previous studies. Overall, the patients’ survival varied from 9 to 16 months, as found from the literature review (31-33). A longer survival time of patients with glioblastoma was associated with the extent of the resection, adjuvant chemoradiation, and molecular makers. The GTR, TMZ, and MGMT methylation were the prognostic factors in the present study, and these findings were consistent with those of previous studies (32-36). A clinical trial discovered that the median overall survival for TMZ with radiation and radiotherapy alone was 16.2 and 9.0 months, respectively (11). Additionally, TMZ plus radiation demonstrated a potential advantage in Stupp’s landmark clinical trial in 2005; this chemotherapy has since become the standard treatment in various countries (9,10,12). Nonetheless, the high price of TMZ would be a significant economic burden in a country with limited resources (33).
The cost-effectiveness of TMZ in a resource-constrained situation has been discussed, and it was noted that selecting patients and forecasting a good prognosis might increase the cost-effectiveness of standard treatment (13). Thus, various prediction tools have been developed and validated for prognostication in glioblastoma patients. Tunthanathip et al. used a nomogram and ML to predict the 2-year survival in glioblastoma patients and reported an acceptable AUC at 0.81–0.82 (17). However, the limitation of the binary outcome prediction showed unseen survival curves of each covariate for the oncologists’ decision of the appropriate treatment strategies. Therefore, prognostication by time-to-event analysis could more serve the physician’s decision-making than binary classification (20).
In the overall prognosis, the survival curve by Cox regression did not overlap with the actual survival curve, but the RSF had the closest to the actual survival curves. Moreover, this algorithm had the lowest RMSE for the prediction of the number of patients at risk over time during the follow-up. Furthermore, the RSF was one of the time-to-event algorithms that could manage right censored survival data and was used in prior studies. Li et al. (35) used the RSF for the recurrent prediction among breast cancer patients and found that the C-index was 0.936, whereas Qiu et al. (36) compared predictive progressive disease in high-grade glioma between the Cox hazard regression and the RSF. As a result, the C-index and Brier scores of the Cox hazard regression were 0.629 and 0.159, whereas the RSF model had C-index and Brier scores of 0.611 and 0.174, respectively. Additionally, deep learning methods with various packages, such as DeepSurv (20), DeepHit (37), and DNNSurv (38) have been used for the survival prediction of several cancers. Katzman demonstrated that the deep learning model performed complicated relationships between a patient’s covariates and their risk of failure, so this approach could be used as personalized treatment recommendations in the future (23). In the present study, we used MLP, which was the neural network from the PySurvival package that revealed an acceptable performance.
For the implication, the personalized survival curves were useful to apply for creating treatment strategies according to the covariates. The survival curves created by the Cox hazard regression, parametric survival model, and MLP were separated into eight conditions; however, eight treatment strategies may be more complicated to apply in real-world practice. While personalized survival curves created by the RSF may be more simplified for implication, the personalized survival curves could be categorized into three groups of poor, average, and good prognosis. This would be one of the resource allocation methods for selecting patients who had a likelihood of cost-effectiveness of high-cost standard treatment (13,14,15,17).
To the authors’ knowledge, the present study was the first paper to compare the prognostication performance among various time-to-event analyses and ML for glioblastoma patients. Moreover, the present study demonstrated the personalized survival curves of each covariate through various survival analyses. However, the limitations of the present study should be acknowledged. A multicenter trial or meta-analysis should be conducted to increase the sample size in the current study. A larger sample size would aid in the investigation of predictors associated with survival time of glioblastoma patients (39,40). The ML model should be estimated with more unseen data to confirm generalizability, which would be a challenge for future prospective studies evaluating these models’ performances (41). Furthermore, the number of patients eliminated due to missing data could lead to biased results for the model’s predictive performance; however, fewer than 10% of patients were excluded in the present study, which was acceptable (42).
Conclusions
In summary, these time-to-event survival approaches were designed to show the personalized survival curves in each condition for a physician to make a personal treatment recommendation. Therefore, choosing patients with a favorable prognosis would lead to cost-effectiveness management for the high-cost standard treatment.
Acknowledgments
The authors would like to offer their special thanks to Assist Professor Kanet Kanjanapradit (Department of Pathology, Faculty of Medicine, Prince of Songkla University, Hat Yai, Songkhla, Thailand) for confirming glioblastoma diagnosis from histological slides.
Funding: None.
Footnote
Reporting Checklist: The authors have completed the TRIPOD reporting checklist. Available at https://jmai.amegroups.com/article/view/10.21037/jmai-22-98/rc
Data Sharing Statement: Available at https://jmai.amegroups.com/article/view/10.21037/jmai-22-98/dss
Peer Review File: Available at https://jmai.amegroups.com/article/view/10.21037/jmai-22-98/prf
Conflicts of Interest: Both authors have completed the ICMJE uniform disclosure form (available at https://jmai.amegroups.com/article/view/10.21037/jmai-22-98/coif). The authors have no conflicts of interest to declare.
Ethical Statement: The authors are accountable for all aspects of the work in ensuring that questions related to the accuracy or integrity of any part of the work are appropriately investigated and resolved. The study was conducted in accordance with the Declaration of Helsinki (as revised in 2013). The study was approved by institutional ethics committee board of the Faculty of Medicine, Prince of Songkla University (No. REC 63-372-10-1). Due to the nature of the retrospective study design, patients were not required to provide informed consent. However, before analysis, patient identification numbers were encoded.
Open Access Statement: This is an Open Access article distributed in accordance with the Creative Commons Attribution-NonCommercial-NoDerivs 4.0 International License (CC BY-NC-ND 4.0), which permits the non-commercial replication and distribution of the article with the strict proviso that no changes or edits are made and the original work is properly cited (including links to both the formal publication through the relevant DOI and the license). See: https://creativecommons.org/licenses/by-nc-nd/4.0/.
References
- Stupp R, Mason WP, van den Bent MJ, et al. Radiotherapy plus concomitant and adjuvant temozolomide for glioblastoma. N Engl J Med 2005;352:987-96. [Crossref] [PubMed]
- Tunthanathip T, Sangkhathat S. Temozolomide for patients with wild-type isocitrate dehydrogenase (IDH) 1 glioblastoma using propensity score matching. Clin Neurol Neurosurg 2020;191:105712. [Crossref] [PubMed]
- Stupp R, Hegi ME, Mason WP, et al. Effects of radiotherapy with concomitant and adjuvant temozolomide versus radiotherapy alone on survival in glioblastoma in a randomised phase III study: 5-year analysis of the EORTC-NCIC trial. Lancet Oncol 2009;10:459-66. [Crossref] [PubMed]
- Esteller M, Garcia-Foncillas J, Andion E, et al. Inactivation of the DNA-repair gene MGMT and the clinical response of gliomas to alkylating agents. N Engl J Med 2000;343:1350-4. [Crossref] [PubMed]
- Hegi ME, Diserens AC, Gorlia T, et al. MGMT gene silencing and benefit from temozolomide in glioblastoma. N Engl J Med 2005;352:997-1003. [Crossref] [PubMed]
- Wick W, Platten M, Meisner C, et al. Temozolomide chemotherapy alone versus radiotherapy alone for malignant astrocytoma in the elderly: the NOA-08 randomised, phase 3 trial. Lancet Oncol 2012;13:707-15. [Crossref] [PubMed]
- Malmström A, Grønberg BH, Marosi C, et al. Temozolomide versus standard 6-week radiotherapy versus hypofractionated radiotherapy in patients older than 60 years with glioblastoma: the Nordic randomised, phase 3 trial. Lancet Oncol 2012;13:916-26. [Crossref] [PubMed]
- Perry JR, Laperriere N, O'Callaghan CJ, et al. Short-Course Radiation plus Temozolomide in Elderly Patients with Glioblastoma. N Engl J Med 2017;376:1027-37. [Crossref] [PubMed]
- Lamers LM, Stupp R, van den Bent MJ, et al. Cost-effectiveness of temozolomide for the treatment of newly diagnosed glioblastoma multiforme: a report from the EORTC 26981/22981 NCI-C CE3 Intergroup Study. Cancer 2008;112:1337-44. [Crossref] [PubMed]
- Uyl-de Groot CA, Stupp R, van der Bent M. Cost-effectiveness of temozolomide for the treatment of newly diagnosed glioblastoma multiforme. Expert Rev Pharmacoecon Outcomes Res 2009;9:235-41. [Crossref] [PubMed]
- Rønning PA, Helseth E, Meling TR, et al. A population-based study on the effect of temozolomide in the treatment of glioblastoma multiforme. Neuro Oncol 2012;14:1178-84. [Crossref] [PubMed]
- Messali A, Hay JW, Villacorta R. The cost-effectiveness of temozolomide in the adjuvant treatment of newly diagnosed glioblastoma in the United States. Neuro Oncol 2013;15:1532-42. [Crossref] [PubMed]
- Wu B, Miao Y, Bai Y, et al. Subgroup economic analysis for glioblastoma in a health resource-limited setting. PLoS One 2012;7:e34588. [Crossref] [PubMed]
- Yang C, Huang X, Li Y, et al. Prognosis and personalized treatment prediction in TP53-mutant hepatocellular carcinoma: an in silico strategy towards precision oncology. Brief Bioinform 2021;22:bbaa164. [Crossref] [PubMed]
- Tunthanathip T, Ratanalert S, Sae-Heng S, et al. Prognostic factors and clinical nomogram predicting survival in high-grade glioma. J Cancer Res Ther 2021;17:1052-8. [Crossref] [PubMed]
- Priya S, Liu Y, Ward C, et al. Machine learning based differentiation of glioblastoma from brain metastasis using MRI derived radiomics. Sci Rep 2021;11:10478. [Crossref] [PubMed]
- Tunthanathip T, Oearsakul T. Machine Learning Approaches for Prognostication of Newly Diagnosed Glioblastoma. Int J Nutr Pharmacol Neurol Dis 2021;11:57-63. [Crossref]
- Lam LHT, Do DT, Diep DTN, et al. Molecular subtype classification of low-grade gliomas using magnetic resonance imaging-based radiomics and machine learning. NMR Biomed 2022;35:e4792. [Crossref] [PubMed]
- Le NQK, Hung TNK, Do DT, et al. Radiomics-based machine learning model for efficiently classifying transcriptome subtypes in glioblastoma patients from MRI. Comput Biol Med 2021;132:104320. [Crossref] [PubMed]
- Katzman JL, Shaham U, Cloninger A, et al. DeepSurv: personalized treatment recommender system using a Cox proportional hazards deep neural network. BMC Med Res Methodol 2018;18:24. [Crossref] [PubMed]
- Louis DN, Perry A, Wesseling P, et al. The 2021 WHO Classification of Tumors of the Central Nervous System: a summary. Neuro Oncol 2021;23:1231-51. [Crossref] [PubMed]
- Weller M, van den Bent M, Preusser M, et al. EANO guidelines on the diagnosis and treatment of diffuse gliomas of adulthood. Nat Rev Clin Oncol 2021;18:170-86. [Crossref] [PubMed]
- Tunthanathip T, Sangkhathat S, Tanvejsilp P, et al. The clinical characteristics and prognostic factors of multiple lesions in glioblastomas. Clin Neurol Neurosurg 2020;195:105891. [Crossref] [PubMed]
- Brigliadori G, Foca F, Dall'Agata M, et al. Defining the cutoff value of MGMT gene promoter methylation and its predictive capacity in glioblastoma. J Neurooncol 2016;128:333-9. [Crossref] [PubMed]
- Thai Thanh Truc. Statistics and Sample Size Pro. Accessed 15 September 2020. Available online: https://play.google.com/store/apps/details?id=thaithanhtruc.info.sass&hl=en_US
- Square Inc. PySurvival. Accessed 15 September 2020. Available online: https://square.github.io/pysurvival/index.html
- Harrell FE, Lee KL, Califf RM, et al. Regression modeling strategies for improved prognostic prediction. Stat Med 1984;3:143-52. [Crossref] [PubMed]
- Löschmann L, Smorodina D. Deep learning for Survival Analysis. Accessed 15 September 2020. Available online: https://humboldt-wi.github.io/blog/research/information_systems_1920/group2_survivalanalysis/
- Pandey A. Survival Analysis: Intuition & Implementation in Python. Accessed 15 September 2020. Available online: https://towardsdatascience.com/survival-analysis-intuition-implementation-in-python-504fde4fcf8e
- The Health Intervention and Technology Assessment Program (HITAP), Thailand. Cost-effectiveness of temozolomide in patients with glioblastoma and anaplastic astrocytoma. Accessed 15 September 2020. Available online: https://www.hitap.net/documents/173270
- Brown NF, Ottaviani D, Tazare J, et al. Survival Outcomes and Prognostic Factors in Glioblastoma. Cancers (Basel) 2022;14:3161. [Crossref] [PubMed]
- Szylberg M, Sokal P, Śledzińska P, et al. MGMT Promoter Methylation as a Prognostic Factor in Primary Glioblastoma: A Single-Institution Observational Study. Biomedicines 2022;10:2030. [Crossref] [PubMed]
- Lacroix M, Abi-Said D, Fourney DR, et al. A multivariate analysis of 416 patients with glioblastoma multiforme: prognosis, extent of resection, and survival. J Neurosurg 2001;95:190-8. [Crossref] [PubMed]
- Wasserfallen JB, Ostermann S, Pica A, et al. Can we afford to add chemotherapy to radiotherapy for glioblastoma multiforme? Cost-identification analysis of concomitant and adjuvant treatment with temozolomide until patient death. Cancer 2004;101:2098-105. [Crossref] [PubMed]
- Li H, Liu RB, Long CM, et al. Development and Validation of a New Multiparametric Random Survival Forest Predictive Model for Breast Cancer Recurrence with a Potential Benefit to Individual Outcomes. Cancer Manag Res 2022;14:909-23. [Crossref] [PubMed]
- Qiu X, Gao J, Yang J, et al. A Comparison Study of Machine Learning (Random Survival Forest) and Classic Statistic (Cox Proportional Hazards) for Predicting Progression in High-Grade Glioma after Proton and Carbon Ion Radiotherapy. Front Oncol 2020;10:551420. [Crossref] [PubMed]
- Lee C, Zame WR, Yoon J, et al. DeepHit: A Deep Learning Approach to Survival Analysis With Competing Risks. Proceedings of the AAAI Conference on Artificial Intelligence; 2018 Feb 2-7, New Orlean, United State. Burnaby: PKP Publishing; 2018.
- Zhao L, Feng D. Deep Neural Networks for Survival Analysis Using Pseudo Values. IEEE J Biomed Health Inform 2020;24:3308-14. [Crossref] [PubMed]
- Tunthanathip T, Sae-Heng S, Oearsakul T, et al. Machine learning applications for the prediction of surgical site infection in neurological operations. Neurosurg Focus 2019;47:E7. [Crossref] [PubMed]
- Taweesomboonyat T, Kaewborisutsakul A, Tunthanathip T, et al. Necessity of in-hospital neurological observation for mild traumatic brain injury patients with negative computed tomography brain scans. J Health Sci Med Res 2020;38:267-74. [Crossref]
- Tunthanathip T, Duangsuwan J, Wattanakitrungroj N, et al. Comparison of intracranial injury predictability between machine learning algorithms and the nomogram in pediatric traumatic brain injury. Neurosurg Focus 2021;51:E7. [Crossref] [PubMed]
- Madley-Dowd P, Hughes R, Tilling K, et al. The proportion of missing data should not be used to guide decisions on multiple imputation. J Clin Epidemiol 2019;110:63-73. [Crossref] [PubMed]
Cite this article as: Tunthanathip T, Oearsakul T. Comparison of predicted survival curves and personalized prognosis among cox regression and machine learning approaches in glioblastoma. J Med Artif Intell 2023;6:10.