ChatGPT and medicine: a potential threat to science or a step towards the future?
Introduction
Natural language processing (NLP) is a subfield of artificial intelligence (AI) that focuses on enabling computers to understand, interpret, and generate human language. NLP has been applied to many industries, including healthcare (1,2). In medicine, NLP can be used to extract useful information from unstructured clinical notes, such as medical history, symptoms, and diagnoses (2,3). This information can then be used for clinical decision-making, population health management, and research. NLP can help identify patients with a high risk of readmission, predict patient outcomes, and improve documentation quality (3). In addition, NLP can be used to analyze social media data to track the spread of infectious diseases, monitor public health trends, and identify adverse drug reactions (3,4). As the volume of medical data continues to grow, NLP is becoming an increasingly valuable tool in healthcare, improving efficiency, accuracy, and patient outcomes (1-4).
Chat generative pre-trained transformer (ChatGPT) is a state-of-the-art NLP model developed by OpenAI. It is based on the GPT-3 architecture and is one of the largest and most advanced language models to date (5). ChatGPT is capable of processing and generating coherent and contextually appropriate responses to natural language prompts, making it ideal for a variety of applications such as chatbots, language translation, and text completion (5,6). It has been trained on a massive dataset of text and can generate responses that are often indistinguishable from those of a human (6,7). With its ability to process and generate human-like language, ChatGPT has the potential to revolutionize the way we communicate with machines and computers, and its applications are expected to grow rapidly in the coming years (8).
Since its creation, ChatGPT has shown great potential for use in medicine due to its ability to generate natural language responses that can be used to improve patient communication and clinical decision-making (1,2,8,9). One of the most promising applications of ChatGPT in medicine is in the development of conversational agents or chatbots that can provide patients with information and support for managing their health conditions. In addition, ChatGPT can be used to analyze large volumes of unstructured clinical data, such as electronic health records and clinical notes, to identify patterns and trends that may be missed by traditional analysis methods (9,10). This can help clinicians make more accurate diagnoses, predict patient outcomes, and develop personalized treatment plans. Overall, ChatGPT has the potential to revolutionize the way healthcare is delivered, improving efficiency, accuracy, and patient outcomes (10). Thus, the aim of the current paper is to give an overview about the use of ChatGPT in medicine and how it can impact each of the specialty.
History
The history of AI is marked by a series of significant breakthroughs and setbacks. In the 1950s, the British mathematician, Alan Turing, proposed the Turing test as a measure of a machine’s ability to exhibit intelligent behavior equivalent to, or indistinguishable from, that of a human (11). In the 1960s, the creation of the first chatbots, such as ELIZA and PARRY, marked a major milestone in AI development (12). However, the AI winter in the 1970s and 1980s saw a decline in funding and interest in AI research (13). In 1997, IBM’s Deep Blue became the first computer to defeat a world chess champion, Garry Kasparov, in a six-game match (14). Apple’s Siri, launched in 2011, brought voice-activated personal assistant technology to the mainstream (15). IBM’s Watson won the Jeopardy! quiz show in 2011, demonstrating the potential for AI to process and understand natural language (16). Amazon’s Alexa, released in 2014, was one of the first virtual assistants to use NLP to respond to user requests (15). Microsoft’s Tay, released in 2016, showed how AI could go wrong, when the chatbot began spewing racist and sexist comments (17). In 2016, AlphaGo, a computer program developed by Google’s DeepMind, beat the world’s best Go player (18). The development of large language models such as GPT-3, which was released in 2020, has revolutionized NLP, allowing for machines to generate coherent and contextually appropriate responses in natural language (19) (Figure 1).
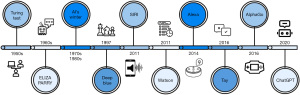
Main concepts
Artificial general intelligence, a hypothetical form of AI, requires significant advancements in machine learning techniques such as deep learning, neural networks, NLP, computer vision, reinforcement learning, supervised and unsupervised learning, and transfer learning to achieve human-level intelligence. Machine learning is a subset of AI that involves teaching computer systems to learn from data and improve their performance on specific tasks (20). Deep learning is a type of machine learning that uses neural networks with multiple layers to process and analyze complex data, such as images and sound. Neural networks are designed to mimic the structure and function of the human brain, allowing them to learn and make decisions based on patterns in data. NLP is a field of AI that focuses on enabling computers to understand, interpret, and generate human language (21). Computer vision is another field of AI that enables computers to understand and interpret visual data, such as images and videos. Reinforcement learning is a type of machine learning where an algorithm learns through trial-and-error interactions with an environment, receiving rewards for positive actions and punishments for negative actions (20,21). Supervised learning is a type of machine learning where the algorithm is trained on labeled data, while unsupervised learning is trained on unlabeled data. Transfer learning is a technique where a pre-trained model is used as a starting point for training a new model on a different but related task. Finally, AI is a hypothetical form of AI that would be capable of performing any intellectual task that a human can do (20,21) (Figure 2).
ChatGPT and medicine
ChatGPT is a powerful NLP model developed by OpenAI that has shown great promise for use in medicine (22,23). One of the most promising applications of ChatGPT in medicine is the development of conversational agents or chatbots that can provide patients with information and support for managing their health conditions. These chatbots can be used to remind patients to take their medication, answer questions about symptoms or side effects, and direct patients to appropriate healthcare services or resources (23). ChatGPT can generate natural language responses that are contextually appropriate and grammatically correct, making it ideal for conversational agents that interact with patients in natural language (22).
Another potential application of ChatGPT in medicine is the analysis of large volumes of unstructured clinical data. Electronic health records and clinical notes contain vast amounts of valuable information, but this data is often difficult to analyze using traditional methods (9). ChatGPT can be used to analyze this data and identify patterns and trends that may be missed by humans. This can help clinicians make more accurate diagnoses, predict patient outcomes, and develop personalized treatment plans (24). In addition, ChatGPT can be used to identify patients at risk of readmission and adverse drug reactions, improving patient safety and reducing healthcare costs (24,25).
ChatGPT may have vast applicability, and its use in medical specialties is of great importance (Figure 3). The Association of American Medical Colleges recognizes 20 specialties, and an overview of the use of ChatGPT in each specialty will be provided (26,27) (Figure 4).
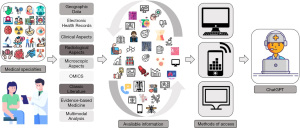
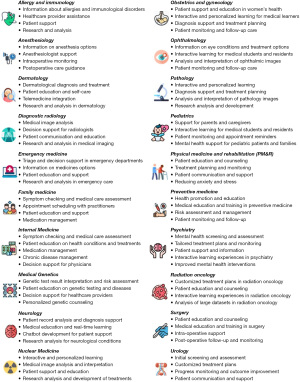
Advantages and limitations of using ChatGPT in medicine
ChatGPT offers a range of potential advantages in the field of medicine. It is accessible 24/7, offering patients convenient access to medical advice and assistance. With quick response times, it reduces waiting times for patients. As a cost-effective alternative to in-person consultations, it can help reduce healthcare costs (9,10). ChatGPT also reduces the risk of disease transmission by not requiring physical contact, making it a safer option. With multilingual support, ChatGPT can provide medical advice in multiple languages, making healthcare more accessible for non-native speakers (9). It can provide tailored responses to patients based on their medical history, symptoms, and other relevant information, ensuring personalized care (22). With consistency in care, ChatGPT provides reliable medical advice to patients, reducing the risk of errors or inconsistencies. Overall, ChatGPT has the potential to improve patient outcomes, increase accessibility, and represent an innovative approach to healthcare delivery (9,10,22).
ChatGPT, while a promising tool in the field of medicine, has several limitations that must be taken into account. One major limitation is its limited empathy, as it may not be able to provide emotional support to patients in the same way that a human healthcare provider can. Additionally, ChatGPT cannot perform physical examinations, which may be necessary for certain medical conditions (6,7,10). It also may lack the clinical judgment and experience necessary to diagnose complex medical conditions or make treatment decisions. ChatGPT may struggle with communication, cultural competency, and language skills, which can affect its ability to provide accurate medical advice to patients from diverse backgrounds (23). Furthermore, it may not have access to all the information necessary to make accurate diagnoses, and may be limited in its scope of medical issues it can address (9,10,19). Patient privacy and data security may also be concerns, as well as the potential for cyber attacks. Overall, while ChatGPT has potential in medicine, its limitations must be carefully considered before relying on it as a primary source of medical advice or treatment (19,23) (Table 1).
Table 1
Benefits |
1. Efficient and quick access to medical information (9,10) |
2. 24/7 availability for medical professionals to ask questions and receive answers (10) |
3. Reduction of research time, leading to faster diagnosis and treatment (22) |
4. Improved accuracy in diagnoses due to access to vast amounts of medical data (9) |
5. Real-time assistance in emergency situations (10,22) |
6. Language translation services for patients who do not speak the local language (5,6) |
7. Personalized medical advice for patients based on their symptoms and medical history (10) |
8. Assistance with clinical decision-making and treatment planning (1,2,8) |
9. Continuous medical education for healthcare professionals (22) |
10. Integration with electronic medical records for seamless information exchange (9) |
11. Increased patient engagement through personalized messaging and reminders (22) |
12. Early detection of potential health issues through symptom tracking (8,22) |
13. Assistance with medication management, including dosing and side effects (23,24) |
14. Provision of mental health support and counseling services (22) |
15. Improved patient satisfaction through faster response times and personalized care (24,25) |
16. Assistance with public health campaigns and disease prevention strategies (24,25) |
17. Improved access to medical care in remote areas with limited medical resources (2,17) |
18. Assistance with medical research and clinical trials (26) |
19. Improved efficiency in hospital administration through automated processes (8,9) |
20. Improved accuracy in medical coding and billing (15,18) |
Limitations |
1. Lack of contextual understanding and empathy compared to human healthcare providers (6) |
2. Limited ability to diagnose complex medical conditions accurately (6,7) |
3. Dependence on accurate input data, which may be difficult to obtain or incomplete (6,10) |
4. Limited ability to analyze and interpret medical images and test results accurately (23) |
5. Inability to perform physical examinations or conduct medical procedures (9) |
6. Lack of ability to handle unexpected or emergency situations (9,10) |
7. Limited ability to account for individual patient differences, such as comorbidities or allergies (10,19) |
8. Dependence on accurate translations for non-native speakers, which may be challenging or result in mistranslations (19) |
9. Inability to provide emotional support and counseling to patients (19,23) |
10. Dependence on reliable and secure technology infrastructure (11,12) |
11. Limited ability to incorporate patient preferences and values into treatment recommendations (8,14) |
12. Dependence on standardized medical language and terminologies, which may not be universally accepted or applicable (9) |
13. Limited ability to identify and address biases in medical data and decision-making (11) |
14. Dependence on the quality and availability of medical data sources, which may be limited or biased (28) |
15. Lack of regulation and accountability for the accuracy and safety of ChatGPT-powered healthcare services (18) |
16. Limited ability to account for ethical and legal considerations in medical decision-making (8,21) |
17. Inability to form meaningful long-term relationships with patients (4) |
18. Dependence on reliable internet connectivity and access to digital devices (7,9) |
19. Limited ability to integrate with existing healthcare systems and infrastructure (13,14) |
20. Lack of privacy and security for patient data, which may be vulnerable to hacking or misuse (29) |
ChatGPT, chat generative pre-trained transformer.
Evidence-based medicine
ChatGPT has the potential to revolutionize evidence-based medicine by providing clinicians with quick and accurate information at their fingertips (8). With its ability to generate text based on large datasets, ChatGPT can assist clinicians in making informed decisions by providing them with the latest research and clinical guidelines. By analyzing patient data, ChatGPT can also help identify potential diagnoses and treatment options, enabling clinicians to make evidence-based decisions (19). Additionally, ChatGPT can assist in the creation of patient education materials, ensuring that patients receive accurate and up-to-date information about their condition and treatment (8). With its vast knowledge base and ability to learn from new data, ChatGPT has the potential to become an invaluable tool in the practice of evidence-based medicine (19).
Ethics and transparency
ChatGPT may provide valuable insights and perspectives on ethical issues that arise in medical decision-making (3,6,8). It can help healthcare providers explore various ethical frameworks, such as autonomy, beneficence, non-maleficence, and justice, to guide their actions (28,29). The tool can assist in discussing the risks and benefits of different treatment options, weighing the considerations of patient privacy and confidentiality, and understanding the implications of emerging technologies like genetic testing or AI in healthcare (28). By facilitating ethical discussions and considerations, ChatGPT can contribute to fostering a more conscientious and responsible approach to medical practice. Moreover, it may enhance transparency in medicine by enabling open and accessible communication between healthcare providers, patients, and the general public (30). It can provide accurate and up-to-date information about medical conditions, treatment options, and research findings, promoting informed decision-making and patient empowerment. Furthermore, it can assist in clarifying complex medical terminology, deciphering scientific jargon, and explaining the rationale behind medical guidelines and recommendations. By facilitating clear and understandable communication, ChatGPT contributes to creating a more transparent healthcare system that fosters trust, collaboration, and shared decision-making among all stakeholders involved (6,30).
While ChatGPT holds significant potential in the realm of medicine, there are a few challenges and concerns that need to be addressed regarding its role in evolving ethics and transparency (8). One of the primary concerns is the lack of accountability and bias in ChatGPT’s responses. As an AI language model, ChatGPT learns from large datasets that may contain biases present in society. These biases can inadvertently influence its responses, potentially leading to unfair or discriminatory suggestions in medical contexts. It is crucial to continuously monitor and mitigate these biases to ensure that ChatGPT’s recommendations align with ethical standards and promote inclusivity in healthcare (3,6,29). Another challenge is the potential for misinformation and inaccuracies in ChatGPT’s responses. Despite its extensive training, ChatGPT may not always possess the most up-to-date medical knowledge or be aware of recent advancements in the field (3). This can be problematic, as healthcare decisions rely heavily on accurate and reliable information. It is essential to regularly update ChatGPT’s knowledge base and integrate mechanisms to verify the accuracy of its responses to minimize the risk of misinformation. Additionally, maintaining transparency about ChatGPT’s limitations is crucial (28). Users should be made aware that ChatGPT is an AI tool and not a substitute for professional medical advice. Transparency is necessary to ensure that patients and healthcare providers understand the boundaries of ChatGPT’s capabilities and use it as a supportive tool rather than relying solely on its suggestions. Lastly, privacy and data security are critical considerations (29). As ChatGPT interacts with users and collects data, safeguarding patient information and complying with privacy regulations become paramount. Stricter protocols and robust encryption methods should be implemented to protect sensitive medical data and ensure patient confidentiality (6,30).
Conclusions
In conclusion, ChatGPT represents a powerful tool in the field of medicine, offering great potential to improve patient care and outcomes. As a language model trained on vast amounts of text data, ChatGPT can assist in clinical decision-making, medical research, and education, among other applications. Its ability to generate human-like responses and understand natural language input makes it an appealing tool for healthcare providers and researchers. While there are still challenges and limitations to overcome, the integration of ChatGPT into medical practice is a promising development that has the potential to transform the way healthcare is delivered and practiced.
Acknowledgments
Funding: This study was supported by the São Paulo State Research Foundation (FAPESP #22/03123-5).
Footnote
Peer Review File: Available at https://jmai.amegroups.com/article/view/10.21037/jmai-23-70/prf
Conflicts of Interest: All authors have completed the ICMJE uniform disclosure form (available at https://jmai.amegroups.com/article/view/10.21037/jmai-23-70/coif). The authors have no conflicts of interest to declare.
Ethical Statement: The authors are accountable for all aspects of the work in ensuring that questions related to the accuracy or integrity of any part of the work are appropriately investigated and resolved.
Open Access Statement: This is an Open Access article distributed in accordance with the Creative Commons Attribution-NonCommercial-NoDerivs 4.0 International License (CC BY-NC-ND 4.0), which permits the non-commercial replication and distribution of the article with the strict proviso that no changes or edits are made and the original work is properly cited (including links to both the formal publication through the relevant DOI and the license). See: https://creativecommons.org/licenses/by-nc-nd/4.0/.
References
- Kreimeyer K, Foster M, Pandey A, et al. Natural language processing systems for capturing and standardizing unstructured clinical information: A systematic review. J Biomed Inform 2017;73:14-29. [Crossref] [PubMed]
- López-Úbeda P, Martín-Noguerol T, Juluru K, et al. Natural Language Processing in Radiology: Update on Clinical Applications. J Am Coll Radiol 2022;19:1271-85. [Crossref] [PubMed]
- Voytovich L, Greenberg C. Natural Language Processing: Practical Applications in Medicine and Investigation of Contextual Autocomplete. Acta Neurochir Suppl 2022;134:207-14. [Crossref] [PubMed]
- Cai T, Giannopoulos AA, Yu S, et al. Natural Language Processing Technologies in Radiology Research and Clinical Applications. Radiographics 2016;36:176-91. [Crossref] [PubMed]
- OpenAI. ChatGPT: Optimizing language models for dialogue. Available online: https://openai.com/blog/chatgpt/ (April 19, 2023).
- Doshi RH, Bajaj SS, Krumholz HM. ChatGPT: Temptations of Progress. Am J Bioeth 2023;23:6-8. [Crossref] [PubMed]
- van Dis EAM, Bollen J, Zuidema W, et al. ChatGPT: five priorities for research. Nature 2023;614:224-6. [Crossref] [PubMed]
- Stokel-Walker C, Van Noorden R. What ChatGPT and generative AI mean for science. Nature 2023;614:214-6. [Crossref] [PubMed]
- Xue VW, Lei P, Cho WC. The potential impact of ChatGPT in clinical and translational medicine. Clin Transl Med 2023;13:e1216. [Crossref] [PubMed]
- Sallam M. ChatGPT Utility in Healthcare Education, Research, and Practice: Systematic Review on the Promising Perspectives and Valid Concerns. Healthcare (Basel) 2023;11:887. [Crossref] [PubMed]
- Mintz Y, Brodie R. Introduction to artificial intelligence in medicine. Minim Invasive Ther Allied Technol 2019;28:73-81. [Crossref] [PubMed]
- Ait-Mlouk A, Jiang L. KBot: A Knowledge Graph Based ChatBot for Natural Language Understanding Over Linked Data. IEEE Access 2020;8:149220-30.
- Kahn K, Winters N. Constructionism and AI: A history and possible futures. Br J Educ Technol 2021;52:1130-42. [Crossref]
- Aylett R, Vargas PA, Sharkey N. 6 Touch and Handling: Could I Shake Hands With A Robot? MIT Press 2021:91-108. doi:
10.7551/mitpress/13487.001.0001 .10.7551/mitpress/13487.001.0001 - Berdasco A, López G, Diaz I, et al. User experience comparison of intelligent personal assistants: Alexa, Google Assistant, Siri and Cortana. Proceedings 2019;31:51.
- Strickland E. IBM Watson, heal thyself: How IBM overpromised and underdelivered on AI health care. IEEE Spectrum 2019;56:24-31. [Crossref]
- Criado N, Such JM. Digital discrimination. In: Yeung K, Lodge M. editors. Algorithmic regulation. Oxford University Press; 2019:82-97.
- Bory P. Deep new: The shifting narratives of artificial intelligence from Deep Blue to AlphaGo. Convergence 2019;25:627-42. [Crossref]
- Lund BD, Wang T, Mannuru NR, et al. ChatGPT and a new academic reality: Artificial Intelligence‐written research papers and the ethics of the large language models in scholarly publishing. J Assoc Inf Sci Technol 2023;74:570-81. [Crossref]
- de Souza LL, Fonseca FP, Araújo ALD, et al. Machine learning for detection and classification of oral potentially malignant disorders: A conceptual review. J Oral Pathol Med 2023;52:197-205. [Crossref] [PubMed]
- Pakdemirli E. A preliminary glossary of artificial intelligence in radiology. Acta Radiol Open 2019;8:2058460119863379. [Crossref] [PubMed]
- Gilson A, Safranek CW, Huang T, et al. How Does ChatGPT Perform on the United States Medical Licensing Examination? The Implications of Large Language Models for Medical Education and Knowledge Assessment. JMIR Med Educ 2023;9:e45312. [Crossref] [PubMed]
- Goodman RS, Patrinely JR Jr, Osterman T, et al. On the cusp: Considering the impact of artificial intelligence language models in healthcare. Med 2023;4:139-40. [Crossref] [PubMed]
- Kalla D, Smith N. Study and Analysis of Chat GPT and its Impact on Different Fields of Study. International Journal of Innovative Science and Research Technology 2023;8:827-33.
- Nori H, King N, McKinney SM, et al. Capabilities of gpt-4 on medical challenge problems. arXiv:2303.13375 [Preprint]. 2023. Available online: https://doi.org/
10.48550/arXiv.2303.13375 - Compton MT, Frank E, Elon L, et al. Changes in U.S. medical students' specialty interests over the course of medical school. J Gen Intern Med 2008;23:1095-100. [Crossref] [PubMed]
-
Johnson D Goodman R Patrinely J Assessing the Accuracy and Reliability of AI-Generated Medical Responses: An Evaluation of the Chat-GPT Model. Preprint. Res Sq 2023 . [Preprint]. doi: .10.21203/rs.3.rs-2566942/v1 - Graf A, Bernardi RE. ChatGPT in Research: Balancing Ethics, Transparency and Advancement. Neuroscience 2023;515:71-3. [Crossref] [PubMed]
- Rahimi F, Talebi Bezmin Abadi A. ChatGPT and Publication Ethics. Arch Med Res 2023;54:272-4. [Crossref] [PubMed]
- Kim BJ. The Intersection of Technology and Humanity: Exploring the Ethics and Potential of Artificial Intelligence in Medicine. J Clin Neurol 2023;19:215-6. [Crossref] [PubMed]
Cite this article as: de Souza LL, Fonseca FP, Martins MD, de Almeida OP, Pontes HAR, Coracin FL, Lopes MA, Khurram SA, Santos-Silva AR, Hagag A, Vargas PA. ChatGPT and medicine: a potential threat to science or a step towards the future? J Med Artif Intell 2023;6:19.