Applying large language model artificial intelligence for retina International Classification of Diseases (ICD) coding
Highlight box
Key findings
• In this study, ChatGPT can analyze retina encounters to generate International Classification of Diseases (ICD) codes with a 70% true positive rate for retina specialists to select from.
What is known and what is new?
• Large language models (LLMs) are emerging artificial intelligence (AI) technologies with numerous benefits in medicine.
• ICD coding is an important, yet time-consuming aspect in many medical specialties.
• This study analyzes ChatGPT, a relatively new and popular LLM, in providing retina specialist providers with a correct ICD code after analyzing the clinic encounter with the goal of increasing efficiency in busy retina clinics.
What is the implication, and what should change now?
• Utilizing the LLM ChatGPT, there may be benefit for applying LLM AI to medical encounters to optimize efficiency and accurate medical coding.
Introduction
The advent of a new generation of chatbots, built using large language models (LLMs), has led to many conversations about the potential benefits and risks of integrating this technology into medicine, including ophthalmology. The most popular LLM-based chatbot, ChatGPT (OpenAI, San Francisco, CA, USA), has been evaluated for its ability to accurately answer ophthalmology exam questions, provide patient information on retinal diseases, and generate differential diagnosis from case reports (1-4). These studies have shown impressive capabilities of ChatGPT, especially compared to previous artificial intelligence (AI) based language models. Many researchers and clinicians have speculated on uses for this technology, with advocates suggesting that LLMs may be useful in decreasing physician burden if integrated into the electronic health record. It has been suggested that ChatGPT could be used to fill out orders or generate International Classification of Diseases (ICD) codes based on medical notes (5). Here, we specifically explore the capability and accuracy of ChatGPT in the proposed task of generating ICD codes.
The ICD classification was designed by the World Health Organization (WHO) to classify medical diseases and procedures, with uses in medical research, tracking healthcare, and billing (6). Specifically, the ICD-10 classification became required in the United States in October of 2015, and its implementation has been found to help provide a more reliable database for research, compared to previous iteration of the ICD (7). ICD-10 codes can be up to seven characters long and a single diagnosis can account for several codes. For example, coding diagnostic retinopathy requires choosing the ICD-10 code based on type, stage, laterality, and includes presence of macular edema or retinal detachment (7,8). Thus, choosing a specific code for a clinical encounter is not a trivial task and requires knowledge of not only the diagnosis, but also familiarity with this coding system.
Currently, the ICD-10 code is assigned by the medical provider which can be a time-consuming task, especially with complex patients who may have multiple ongoing medical problems. This coding system also introduces potential for human errors and there may be inconsistencies in coding practices among various practices and providers. Leveraging AI for this task poses the ability to streamline the coding process, increasing efficiency in clinical settings and providing more consistency in coding practices. Additionally, OpenAI has released a “research demo” version of ChatGPT that is free and easy to use, unlike some of the other language models. In this work, we evaluate ChatGPT in its ability to generate ICD-10 codes based on mockup patient encounters generated by three retina specialists. In this study, we provide ChatGPT with example cases and compare the ChatGPT-generated ICD codes to that of specified by the retina specialist.
Methods
Text of a mockup retina clinic encounter of various types of visits including new patient visit, return visit, post-operative visit, and injection-only visit were generated by three retina specialists. The text was inputted into ChatGPT with a request to provide an ICD-10 code. No feedback was given to ChatGPT following any encounter and the encounters were entered sequentially without starting a new chat. Considering the nature of the study, the study was exempted from institutional review board. The result provided by ChatGPT included a definition in addition to the ICD code, as seen in Figure 1, however evaluation was based on the numeric ICD code provided, rather than the definition. The ICD code provided by ChatGPT was then converted into a formal diagnosis based on the ICD handbook. The diagnosis given by the ChatGPT-generated ICD code was compared to the diagnosis provided by the retinal specialist at the retina clinic.
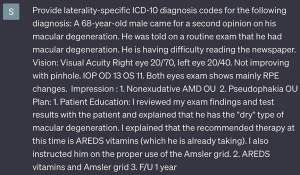
If a retina clinic encounter was treating both eyes for retinal pathologies, both eyes would be evaluated for ChatGPT’s ICD code generation. As this pilot study was aimed at a retina encounter, evaluation of ChatGPT output was done for the retinal pathologies mentioned; notes often mentioned phakic status and thus a code for cataract or intraocular lens was also generated. If multiple retinal diagnoses were mentioned in the retinal encounter, the primary code would be evaluated (e.g., a visit for exudative age-related macular degeneration would be evaluated for the exudative age-related macular degeneration code, even if a code for an epiretinal membrane was described. However, if the patient were coming to the clinic for epiretinal membrane, the epiretinal membrane code would be evaluated). A code that may have been generated for a retinal diagnosis that was not mentioned in the retina encounter was included in the sub-analysis but not for evaluation of the determined ICD code from ChatGPT on whether it got the ICD code correct unless it was the only retina ICD available. Additionally, ChatGPT often provided an “X” in its ICD output [e.g., “non-exudative age-related macular degeneration: right eye (OD): H35.31X1”]. There is no “X” in ICD codes but looking at online sources, these “X”s are utilized as placeholders. If an “X” was provided, this “X” was omitted to generate a true numeric-only code e.g., “H35.31x1” was evaluated as “H35.311”. All encounters without a retinal pathology or diagnosis in the note were excluded. A true positive was assigned for an encounter when any of the ICD codes generated by ChatGPT matched the actual retinal diagnosis from the clinic encounter. The rationale for having any ICD code correct as the criteria for true positive is that LLMs may help to optimize clinic flow and allow for retina specialists to quickly select from generated ICDs, rather than fully relying on the LLM for providing the only correct ICD without a retina specialist confirming.
Results
A total of 181 mockup retina encounters were evaluated, with 84 eyes as right eyes, 97 eyes as left eyes. A total of 597 ICD codes were generated, with 305 consisting of retina codes (51% of total consisting of retina codes, 1.68 retina codes per eye). This total code count also included past medical history included in the note including hyperlipidemia, hypertension, and hyperthyroidism. In total, 127/181 (70%) of responses resulted in a true positive result with at least one code provided matching a correct code. In total, 54/181 (30%) responses did not generate a correct code from the text. An additional sub-analysis analyzed whether ChatGPT coded the retina encounter and diagnosis completely correct. If ChatGPT got any of it incorrect, it would be counted as incorrect in this analysis even if some were correct. In this analysis, ChatGPT achieved a “correct only” in 106 of 181 encounters (59%) with the remaining 75 encounters (41%) having some form of incorrect diagnosis even if it included the correct diagnosis (Table 1). Additionally, all of the ChatGPT responses included one of the following notes: “Please note that the codes provided are for reference purposes only. It is important to consult the complete ICD-10 coding manual and validate the codes with the most recent version for accurate and specific coding. The assigned codes should be based on the patient’s medical records and condition, and they should be assigned by a healthcare professional.” or “Please note that ICD-10 codes may change over time, so it’s always a good idea to refer to the most current coding guidelines and resources for accurate coding”. Figure 2 showcases ChatGPT’s ability to provide a correct ICD code for retina specialists to select from (“H35.31—non-exudative age-related macular degeneration” for a bilateral non-exudative age-related macular degeneration visit), but may also fall into traps to provide incorrect codes at times (“H35.32—exudative age-related macular degeneration” for the same visit with no exudative age-related macular degeneration diagnosis).
Table 1
Subgroup | Results | Percentage |
---|---|---|
Correct | 137/181 | 70 |
Correct only | 106/181 | 59 |
Incorrect | 54/181 | 30 |
“Correct” was defined by producing at least one correct ICD code for a clinician to choose from; “correct only” was defined as generating only the correct ICD codes for the encounter; “incorrect” was defined as not generating any correct ICD codes for the mockup retina encounter. ICD, International Classification of Diseases.
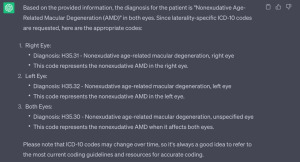
Discussion
Overall, ChatGPT provided the correct ICD codes most of the time, with a 70% true positive rate. As a strength, ChatGPT was able to withhold from giving a diagnosis from an eye if only one eye was affected. In addition, it would occasionally note when laterality (right eye/left eye) was not indicated in the encounter with the following message: “Please note that the information does not indicate any specific laterality for the vitreous degeneration or refractive error”.
However, despite the 70% true positive rate, there were several weaknesses in ChatGPT’s ability to provide ICD codes. First, there were instances when ChatGPT provided an impressive description of the pathology which should have resulted in the correct ICD code, but the ICD code provided was completely fabricated. For example, ChatGPT gave an output of “advanced non-exudative age-related macular degeneration, familial variant: H35.3491” which does not exist as an ICD-10 code. Interestingly, ChatGPT would occasionally also give an ICD code for the absence of a disease. For example: “no evidence of diabetic retinopathy: E11.329”.
Another weakness was that if there was non-exudative age-related macular degeneration in both eyes, ChatGPT would occasionally code the right eye correctly but then code the left eye as exudative. Wrong eye designation by ChatGPT was done a total of 12 times (6.6%). Looking at the ICD codes, the numerical difference between the right and left eye is 0.001 (e.g., H35.311 is non-exudative age-related macular degeneration, right eye, and H35.312 is non-exudative age-related macular degeneration, left eye), but ChatGPT instead added 0.01 to H35.31 (non-exudative age-related macular degeneration) to become H35.32 (exudative age-related macular degeneration) which led to an error. This trend often occurred sequentially, possibly because no feedback was given to ChatGPT. Towards the end of the analysis, ChatGPT started making more incorrect assignments solely on eye designation, but the underlying pathology was correct (e.g., “H35.322” for the right eye which equates to “exudative age-related macular degeneration, left eye”). This mistake may have been avoided by creating a new chat every time. Providing ChatGPT with feedback when it made such a mistake may have also improved its performance, which is a future direction in this research. It has been shown that the quality of output is sensitive to the prompt given. For example, one work found that adding the phrase “Let’s think step by step” significantly improved the reasoning capabilities of language models (9). Similarly, providing ChatGPT with feedback may have improved its ability to generate sensible ICD codes.
It is also well-documented that ChatGPT can “hallucinate” information with the creators of ChatGPT warning that the chatbot can sometimes “write plausible-sounding but incorrect or nonsensical answers” (10). This may account for instances in which ChatGPT was able to provide an accurate description of the condition but proceeded to fabricate an ICD code. Additionally, the ICD codes are updated yearly, whereas ChatGPT was trained on data prior to 2021 and is not regularly updated with new information (11). There are plug-ins available that allow it to access the internet but these currently limited in scope (12). However, with availability to the internet and the updated ICD code guidelines, perhaps ChatGPT would have provided more accurate results.
There are also potential ethical and academic implications for the deployment of ChatGPT for diagnostic coding. Medical billing is a process that requires utmost professionalism and integrity from clinicians. Ensuring consistency and accuracy of ICD coding for matching clinical documentation encounters serves to fair reimbursement for providers. Mismatches in ICD coding may result in dilemmas for various individuals including the clinician, patient, insurance, and stakeholders; thus, it is important to note that the utilization of LLMs may be utilized to assist in the efficiency of a medical practice, but the clinician should review and finalize the final selection provided. Another point of discussion is that this utilization of LLMs for ICD coding may generate further discussions in the academic research and quality-improvement setting such as registry-based studies. These studies may be impacted under full-autonomous ICD coding, thus, highlighting the importance of the clinician to ensure that the ICD codes provided by the LLM match the clinical encounter.
In addition to the current limitations to ChatGPT, there are also several limitations to this study. First, we applied no feedback to ChatGPT. Given the strength of LLMs to learn based on feedback, this was a major limitation to fully utilizing the potential of LLM technology. Another limitation is that not all ICDs provided by ChatGPT were evaluated during the encounter. This limitation may not be as critical for a sub-specialty clinic such as a retina clinic that is actively examining AMD, however, this may be a limitation regarding understanding ChatGPT for specialties such as primary care where multiple pathologies may be extensively discussed for each patient. Additionally, ICD codes are often reviewed by billing specialists and may sometimes be requested to be revised; both the clinic ICD and ChatGPT generated ICD were not compared side-by-side regarding accuracy in the real-life setting of billing specialists, however, this may serve as a future study to determine if it’s a real-life applicability.
This study also utilizes the version of ChatGPT which is built on GPT-3.5. Several studies have shown that the newer GPT-4 model outperforms GPT-3.5 with higher performance on a number of tasks (11,13). However, for this initial analysis, we focused on GPT-3.5 since it is freely available whereas GPT-4 requires a paid subscription. Other less popular and less accessible, LLMs have also been developed that are specifically trained with data from the EHR and thus may perform better at this task. For example, ClinicalBERT and GatorTron are LLMs that were developed based on clinical notes from different medical centers (14-16). Unlike GPT-3.5, which was trained on a large corpus of text, these types of models are more targeted towards clinical tasks and may result in better ICD code generation. Furthermore, providing the ICD codes with their definitions in the original prompt to ChatGPT may have greatly improved performance since then the model would not rely on its original training, which includes texts from across the internet, but instead focused on the definitions of ICD codes given to it in the prompt. Future research may be utilized to analyzing prompt engineering and feedback fine-tuning of LLMs in ICD coding against LLMs without feedback fine-tuning to stratify and compare the benefits of improving.
ICD coding has also been an interest in other forms of AI. Teng et al. discuss the application of deep neural networks for ICD coding (17). As ICD coding is vulnerable to human error, there has been prior research with machine learning and deep learning techniques. One of the large challenges noted in ICD coding includes distinct writing styles, non-relevant information for coding, and long documents. These variables represent challenges in consistent ICD coding, however, Teng et al. describe a general deep neural network architecture for ICD coding that employs an input layer, representation layer, feature layer, and output layer. The input layer employs multi-source data input, including external knowledge, such as the ICD-10 taxonomy, free-input that comes from the health records, and the code relationship. The technical aspects of the representation and feature are outside the scope of this discussion, however, the feature layers employ convolutional neural networks (CNNs) to extract various features from the data that are critical for output layer generation. Lastly, the output layer generates the ICD code as well as takes into account the loss function with subsequent back-propagation for further optimization. Teng et al. discusses various CNNs and their iterations including attention-convolution and dilated convolution that can be applied to ICD coding. Ultimately, these advances in AI applied to ICD coding may be merged with advances seen here in LLM technology to construct more accurate and efficient models.
Research in other aspects of the LLM optimization have been explored including being able to visualize/analyze images and engaging with the technology with voice. In September 2023, OpenAI announced the ability of ChatGPT to analyze voice and image input (18). This optimization of LLM input may further optimize ICD coding. Along with further validation in cybersecurity and privacy, this technique may have the potential to take in voice-based clinical encounter discussions to generate ICD codes. This would further optimize clinic workflow compared to the methods of the aforementioned study as no text input would be required. Additionally, clinicians may not document their final encounter note immediately after the clinical encounter, thus, ICD code generation with LLMs must be performed after this manual step. By employing voice-based LLMs, ICD code selections may be available immediately after the patient encounter has finished. Future research may be geared towards these optimizations in LLM technology in ICD coding as well as the cybersecurity research that must go behind these technologies to ensure safe clinical implementation.
Conclusions
Overall, in this study, we reviewed how ChatGPT could be used in the practice of generating ICD codes. As it stands, the widely available version of ChatGPT can provide accurate responses most of the time but still has several drawbacks, including hallucinating false codes or mistaking numbers despite understanding the diagnosis. This limits its use in current clinical practice but prompt tuning with code definitions, access to the internet, a more advanced language model like GPT-4, or models specifically trained on EHR records may improve the performance of LLMs such as ChatGPT in the task of ICD code generation. This could improve clinical efficiency and reduce inconsistencies in ICD coding.
Acknowledgments
Funding: None.
Footnote
Data Sharing Statement: Available at https://jmai.amegroups.com/article/view/10.21037/jmai-23-106/dss
Peer Review File: Available at https://jmai.amegroups.com/article/view/10.21037/jmai-23-106/prf
Conflicts of Interest: All authors have completed the ICMJE uniform disclosure form (available at https://jmai.amegroups.com/article/view/10.21037/jmai-23-106/coif). JC has received fees from Allergan, Salutaris, and Biogen. The other authors have no conflicts of interest to declare.
Ethical Statement: The authors are accountable for all aspects of the work in ensuring that questions related to the accuracy or integrity of any part of the work are appropriately investigated and resolved.
Open Access Statement: This is an Open Access article distributed in accordance with the Creative Commons Attribution-NonCommercial-NoDerivs 4.0 International License (CC BY-NC-ND 4.0), which permits the non-commercial replication and distribution of the article with the strict proviso that no changes or edits are made and the original work is properly cited (including links to both the formal publication through the relevant DOI and the license). See: https://creativecommons.org/licenses/by-nc-nd/4.0/.
References
- Antaki F, Touma S, Milad D, et al. Evaluating the Performance of ChatGPT in Ophthalmology: An Analysis of Its Successes and Shortcomings. Ophthalmol Sci 2023;3:100324. [Crossref] [PubMed]
- Potapenko I, Boberg-Ans LC, Stormly Hansen M, et al. Artificial intelligence-based chatbot patient information on common retinal diseases using ChatGPT. Acta Ophthalmol 2023;101:829-31. [Crossref] [PubMed]
- Raimondi R, Tzoumas N, Salisbury T, et al. Comparative analysis of large language models in the Royal College of Ophthalmologists fellowship exams. Eye (Lond) 2023; Epub ahead of print. [Crossref] [PubMed]
- Balas M, Ing EB. Conversational ai models for ophthalmic diagnosis: Comparison of chatgpt and the isabel pro differential diagnosis generator. JFO Open Ophthalmology 2023;1:100005. [Crossref]
- DiGiorgio AM, Ehrenfeld JM. Artificial Intelligence in Medicine & ChatGPT: De-Tether the Physician. J Med Syst 2023;47:32. [Crossref] [PubMed]
- World Health Organization. International Statistical Classification of Diseases and related health problems: Alphabetical index. Geneva: World Health Organization; 2004.
- Cai CX, Michalak SM, Stinnett SS, et al. Effect of ICD-9 to ICD-10 Transition on Accuracy of Codes for Stage of Diabetic Retinopathy and Related Complications: Results from the CODER Study. Ophthalmol Retina 2021;5:374-80. [Crossref] [PubMed]
- Dugan J, Shubrook J. International Classification of Diseases, 10th Revision, Coding for Diabetes. Clin Diabetes 2017;35:232-8. [Crossref] [PubMed]
- Kojima T, Gu SS, Reid M, et al. Large language models are zero-shot reasoners. Adv Neural Inf Process Syst 2022;35:22199-213.
- OpenAI. Introducing ChatGPT. 2022. Available online: https://openai.com/blog/chatgpt
- OpenAI. GPT-4 Technical Report. Available online: https://cdn.openai.com/papers/gpt-4.pdf
- OpenAI. ChatGPT Plugins. 2023. Updated March 23, 2023. Available online: https://openai.com/blog/chatgpt-plugins
- Lee P, Bubeck S, Petro J. Benefits, Limits, and Risks of GPT-4 as an AI Chatbot for Medicine. N Engl J Med 2023;388:1233-9. [Crossref] [PubMed]
- Johnson AE, Pollard TJ, Shen L, et al. MIMIC-III, a freely accessible critical care database. Sci Data 2016;3:160035. [Crossref] [PubMed]
- Huang K, Altosaar J, Ranganath R. Clinicalbert: Modeling clinical notes and predicting hospital readmission. arXiv:1904.05342. 2019. Available online: https://arxiv.org/abs/1904.05342
- Yang X, Chen A, PourNejatian N, et al. A large language model for electronic health records. NPJ Digit Med 2022;5:194. [Crossref] [PubMed]
- Teng F, Liu Y, Li T, et al. A review on deep neural networks for ICD coding. IEEE Trans Knowl Data Eng 2022;35:4357-75. [Crossref]
- OpenAI. ChatGPT can now see, hear, and speak. 2023. (Accessed 10/1/23). Available online: https://openai.com/blog/chatgpt-can-now-see-hear-and-speak
Cite this article as: Ong J, Kedia N, Harihar S, Vupparaboina SC, Singh SR, Venkatesh R, Vupparaboina K, Bollepalli SC, Chhablani J. Applying large language model artificial intelligence for retina International Classification of Diseases (ICD) coding. J Med Artif Intell 2023;6:21.