Racial/ethnic reporting differences in cancer literature regarding machine learning vs. a radiologist: a systematic review and meta-analysis
Highlight box
Key findings
• This study found a lack of inclusion of racial/ethnic and other important demographic information in cancer literature comparing artificial intelligence (AI) to radiologists.
What is known and what is new?
• Machine learning (ML) holds promise in aiding diagnosis of patient conditions. However, studies are increasingly highlighting racial/ethnic biases in ML models despite evidence showing variation in condition status amongst different population groups.
• This study evaluates if AI algorithms targeted at cancer prediction account for ethnoracial biases in their model training.
What is the implication, and what should change now?
• Cancer outcomes and diagnoses show significant disparities across races. ML algorithms need to consider demographic information when training on a patient population. AI built on datasets that ignore race may lack generalizability to other patient populations. It is important to not only report this information for AI for cancer imaging, but to also consider it when training a model.
Introduction
Background
Machine learning (ML) has emerged as a promising tool to assist physicians in diagnosis and classification of patient conditions from medical imaging data. ML algorithms have been used to diagnose many conditions including breast cancer (1), diabetes (2), heart disease (3), Parkinson’s (4), and Coronavirus Disease 2019 (COVID-19) (5). However, as ML has become more common, so has more attention been directed to the impact of racial biases due to imbalanced datasets (6). A recent study found that despite the evident differences in how diabetes affects different populations, artificial intelligence (AI) algorithms often did not take racial/ethnic differences into account or even report what percentages persisted in their training sets (7). This is important as it has been well documented that health disparities and complications within diabetes exist for minority subgroups (8). A recent study found that diabetes occurs earlier and at lower body mass index (BMI) in Asian, Hispanic, and Black/African American populations than in White populations, indicating the need for increased screening (9). Results from ML studies are increasingly emphasizing the need for proper racial evaluation of models and datasets, as many studies including different racial/ethnic groups into their models are showing significant variation in performance by race, increasing the likeliness of potential biases and disparities in care (10-12).
Rationale and knowledge gap
It has long been known that cancer outcomes vary for different racial/ethnic groups (13). For example, there are known disparities in cancer outcomes for Black/African American and Hispanic minorities suffering from breast cancer (14-16), prostate cancer (17), lung cancer (18), colorectal cancer (19), and pancreatic cancer (20). Beyond racial/ethnic status, there are known disparities by gender for cancers such as liver cancer, where men die at a much higher rate than women (21). It has been found that health disparities amongst racial/ethnic subgroups can be further exacerbated due to socioeconomic status (SES) (22), English proficiency (23), environmental pollution based on community locations (24), cultural diets and habits (25), and genetic factors (26). Lack of inclusion of minority subgroups in ML models for cancer diagnoses can have an even more direct impact on decreased performance and further health disparities. For example, ML provides opportunities for early detection of skin cancers such as melanoma, which allows for optimal efficacy of treatment (27). However, recent studies have found an underrepresentation of certain demographic groups such as Black/African American patients in dermatological predictive models which can lead to models unable to accurately predict cancers for darker skin types (28,29).
Objective
We previously conducted a review of 61 articles that compared the performance of radiologists to ML algorithms in regard to cancer diagnoses and prediction (30). It is important to also consider if algorithms targeted at cancer prediction account for different racial/ethnic groups present in their training samples and the adequacy of those samples. We surveyed literature regarding ML prediction of cancer and its performance compared to a radiologist’s prediction to see if these articles also reported any racial/ethnic information. Cancer outcomes can vary greatly based on different racial/ethnic groups; as such, it is important to draw attention to the need for more diversity and better reporting within ML datasets. The study is presented in accordance with the PRISMA reporting checklist (available at https://jmai.amegroups.com/article/view/10.21037/jmai-23-31/rc) (31).
Methods
Literature review
Online literature databases (PubMed, MEDLINE, and Google Scholar) were searched for studies that reported results of ML algorithms compared to radiologists for cancer prediction. Literature search was conducted by two independent researchers and any variations were subsequently resolved. All studies published before May 2022 were considered. Papers were excluded if an inappropriate imaging type was used to train a model [such as dual energy X-ray absorptiometry (DEXA) to identify breast cancer], if they did not report results on prediction of cancer (benign vs. malignant), if they did not compare algorithm to a radiologist, if they did not use a board-certified radiologist, or if they were not in English. Key terms used to identify literature are summarized in Table 1. Papers were independently reviewed by the same two researchers for demographic and other reported information.
Table 1
Subject | Key terms |
---|---|
Article type | Clinical study, clinical trial, controlled clinical trial, journal article, randomized controlled trial |
Machine learning keywords | Machine learning, artificial intelligence, neural networks (NN), support vector machine (SVM), naïve Bayes, logistic regression, convolutional neural network (CNN), deep learning, random forest, decision tree |
Clinical keywords | Cancer, radiologist, physician, clinician |
Exclusion criteria | Does not contain prediction of benign vs. malignant histology, not in English, does not use board certified radiologist, does not compare algorithm to a radiologist |
Additional filters | Radiologist deemed imaging technology inappropriate for type of cancer diagnosis (e.g., DEXA for breast cancer) |
DEXA, dual energy X-ray absorptiometry.
Quality assessment of literature
Alongside the quality assessment of reported patient information in this study, manuscripts were assessed for completeness and overall quality through a modified CLAIM checklist pertaining to ML within radiology (30,32). Manuscripts were reviewed for quality of ML algorithm, results, reported data, and risk of bias through an evaluation of reported metrics, use of correct validation datasets and methods, and reproducibility. Manuscripts were specifically searched for reported metrics [area under the receiver operating characteristic curve (AUC), accuracy, positive predictive value (PPV) negative predictive value (NPV), etc.], inclusion of a separate training and testing set and cross validation or hold-out sample, features included, and whether sufficient information was provided for replication. Manuscripts were assessed for image quality analysis, including whether it was conducted, mentioned, or if images were excluded or included based on quality assessment. Information regarding the quality assessment of literature is reported in Table S1.
Meta analysis
Studies included in this meta-analysis were evaluated for inclusion of demographic information in addition to information of algorithm and radiologist performance. Total papers identified, imaging type, and cancer site were summarized. Studies included in this review are cited in Table S2.
This study considered demographic information to be age, racial/ethnic status, and gender. This study also looked to see if additional history or patient information (such as smoking status or BMI) was reported by the original publication. SES was not considered for this meta-analysis due to the overall lack of reporting in literature. Papers were evaluated first for inclusion of any demographic information regarding the patient population in the study overall. Papers were then evaluated for incorporation and inclusion of demographic information in the algorithm. A study was considered to have included demographic information into a model if an analysis was performed that looked at the statistical impact of at least one demographic variable on the algorithm performance (for instance, if the study included a table of P values for demographic information). For demographic information to be considered included in the model, the study had to include demographic information as a variable input, had to report a P value regarding performance of the demographic subgroup on the model, or had to evaluate model performance by demographic subgroup.
Literature that reported racial/ethnic information was evaluated for total makeup of patient population and inclusion of which categories of reported information.
Statistical analysis
Statistical analysis was performed to determine the prevalence and distribution of studies categorized by various reported demographic information. For studies presenting racial/ethnic information, we conducted further analysis to examine the frequency and distribution of each racial group. Manuscripts that incorporated racial/ethnic information into the algorithms were then reviewed for presence of statistical analyses regarding demographic variables and appropriate use of chosen statistical tests.
Results
Literature search results
The literature search resulted in 115 studies (Figure 1) (31). After screening for full text eligibility based on the inclusion criteria, 31 studies were excluded. The remaining 84 studies were identified for inclusion in this meta-analysis. Information regarding total studies, cancer sites, and imaging type are reported in Table 2. The most common cancer site identified in the review was the breast (26 studies), and the most common imaging method utilized was magnetic resonance imaging (MRI) (35 studies).
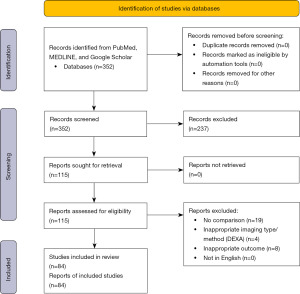
Table 2
Cancer site | Total (n=84) | Imaging method | |||||
---|---|---|---|---|---|---|---|
CT | Mammogram | MRI | Shear wave elasticity images | Ultrasound | X-ray | ||
Breast | 26 | 1 | 8 | 6 | 0 | 9 | 2 |
Central nervous system (brain, spine) | 8 | 0 | 0 | 8 | 0 | 0 | 0 |
Gastrointestinal | 9 | 4 | 0 | 4 | 1 | 0 | 0 |
Genitourinary (prostate, bladder, kidney, adrenal) | 11 | 5 | 0 | 6 | 0 | 0 | 0 |
Gynecology (ovaries, uterus) | 9 | 0 | 0 | 9 | 0 | 0 | 0 |
Head and neck (lymph thyroid) | 7 | 1 | 0 | 0 | 0 | 5 | 1 |
Sarcoma (soft tissue, fatty tissue) | 4 | 1 | 1 | 2 | 0 | 0 | 0 |
Thorax (lungs, chest) | 10 | 7 | 0 | 0 | 0 | 0 | 3 |
CT, computed tomography; MRI, magnetic resonance imaging.
Breakdown of total demographic information reported and type of demographic information reported is summarized in Figure 2. A total of 73 studies reported demographic information, and 32 evaluated the effect of demographic information on model performance.
Only nine reported racial/ethnic groups, and only three of those studies incorporated racial/ethnic groups into their models. Of the nine studies that reported this information, the specified racial/ethnic minorities that were the most included were White/Caucasian (n=9/9) and Black/African American (n=8/9). Asian (n=4/9), American Indian (n=3/9), and Hispanic (n=2/9) were reported in less than half of the studies. Percentage of racial/ethnic minorities included in each patient population and which racial/ethnic minorities were reported are summarized in Figure 3.
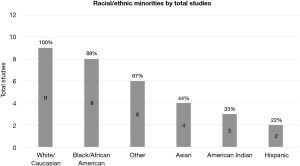
Two of the three studies that incorporated racial/ethnic status into the studies’ respective analyses reported statistical significance of race on the algorithm performance. Yala et al. demonstrated that inclusion of race/ethnicity caused significant diagnostic improvements for breast cancer of a hybrid deep learning model built on mammogram imaging and traditional risk factors (33). Performance of this deep learning model was improved compared to the current clinical standard for breast cancer risk prediction: the Tyrer-Cusick model. Yala et al. also evaluated the differences in the model improvements for different racial subtype groups. Inclusion of race/ethnicity caused statistically significant increases in the AUC for White/Caucasian (P<0.001) and Black/African American (P<0.001), which resulted in a model that performed well for both ethnicities.
In contrast, Beig et al. found that race was not a statistically significant factor in algorithm performance (P=0.97) (34). Incorporated demographic variables and reported P values for Yala et al. and Beig et al. are summarized in Table 3.
Table 3
Studies | Incorporated demographic variables | Reported P value |
---|---|---|
Yala et al. | Patient history | |
Premenopausal women | 0.40 | |
Postmenopausal women | <0.001 | |
Race | ||
White | <0.001 | |
Black/African American | <0.01 | |
Beig et al. | Gender | 0.38 |
Age | <0.01 | |
Patient history (smoking status) | <0.01 | |
Race | 0.97 |
Additionally, Schaffter et al. did not report any statistical testing of race on algorithm performance (35). Schaffter et al. instead used a stratified sample for training the model that ensured racial groups were evenly distributed amongst training and testing groups.
Discussion
Key findings
The majority (73/84) of the manuscripts reviewed in this meta-analysis included some form of demographic information. However, only nine of these attempted to describe racial/ethnic information for the reported patient population. The lack of inclusion of information of not only race, but also demographic information such as age, gender, BMI, or patient history is indicative of a larger problem that exists within AI for cancer imaging. There are significant disparities in cancer outcomes and diagnoses for different races. Additionally, it has been shown that patient race can be identified from medical imaging (36). Many algorithms do not consider information such as race within consideration of prediction of clinically significant cancer. However, race is often correlated with different physical attributes such as BMI, which makes evaluation of race as a unique demographic variable important for downstream treatment of a patient as well. It is important to report and consider demographic information when considering not only AI for cancer, but overall care of a cancer patient. ML algorithms need to consider demographic information when evaluating a patient population for training the algorithm. AI built on datasets that do not consider race may be built entirely on homogeneous patient populations that do not generalize well to other datasets.
Implications
It is vital to account for racial/ethnic groups in ML models as carefully addressing these disparities can lead to improved treatment outcomes (chemotherapy, radiation therapy, etc.), reduction of inequalities present in the lack of technology access of certain minorities, prevention of biases, and further integration of relevant social determinants of health. Race has been shown to affect outcomes of cancer treatment such as chemotherapy (37) and radiation therapy (38,39); further, patient attributes that matter to treatment such as BMI are often strongly correlated with race/ethnicity status. By not including multiple races/ethnicities into the modeling process, the lack of heterogenous genetic information in open source datasets can further exacerbate a lack of access of certain races/ethnicities to personalized medicine and treatments. Improper training of models on non-representative datasets can cause racial biases that skew performance (40). Algorithms try to maximize overall prediction accuracy by optimizing for those individuals which appear frequently in the training data. This can cause variable performance for different racial/ethnic groups. Additionally, the performance of the predictors used in the models could substantially vary across different populations. There is a need to establish a diversity standard and prioritization of racial and social determinants of health data collection as well as the need for thorough evaluation of ML algorithms in race subgroups before clinical deployment to reduce bias. ML algorithms should be carefully designed to be ethical and reliable so that all demographic populations obtain equal benefit, with equal performance amongst groups and proper allocation of resources during clinical usage.
Limitations
This study was limited in that it only evaluated manuscripts that compared an AI to a radiologist. Many of these were feasibility studies that also did not report more information regarding the algorithm itself. Additionally, this study only evaluated demographic information for manuscripts evaluating AI for cancer. A broader review might reveal even more problems with demographic reporting present in the wider AI for medicine community.
Conclusions
An evaluation of 84 manuscripts regarding AI in cancer found that only 9 reported racial/ethnic information for the patient population involved in the study. Only 3 of these studies incorporated racial/ethnic information into the final model. It is important to not only report this information for AI for cancer imaging, but to also consider it when training a model.
Acknowledgments
Funding: None.
Footnote
Reporting Checklist: The authors have completed the PRISMA reporting checklist. Available at https://jmai.amegroups.com/article/view/10.21037/jmai-23-31/rc
Peer Review File: Available at https://jmai.amegroups.com/article/view/10.21037/jmai-23-31/prf
Conflicts of Interest: All authors have completed the ICMJE uniform disclosure form (available at https://jmai.amegroups.com/article/view/10.21037/jmai-23-31/coif). The authors have no conflicts of interest to declare.
Ethical Statement: The authors are accountable for all aspects of the work in ensuring that questions related to the accuracy or integrity of any part of the work are appropriately investigated and resolved.
Open Access Statement: This is an Open Access article distributed in accordance with the Creative Commons Attribution-NonCommercial-NoDerivs 4.0 International License (CC BY-NC-ND 4.0), which permits the non-commercial replication and distribution of the article with the strict proviso that no changes or edits are made and the original work is properly cited (including links to both the formal publication through the relevant DOI and the license). See: https://creativecommons.org/licenses/by-nc-nd/4.0/.
References
- jmai-6-0undefined-25
- Aljuaid H, Alturki N, Alsubaie N, et al. Computer-aided diagnosis for breast cancer classification using deep neural networks and transfer learning. Comput Methods Programs Biomed 2022;223:106951. [Crossref] [PubMed]
- Naz H, Ahuja S. Deep learning approach for diabetes prediction using PIMA Indian dataset. J Diabetes Metab Disord 2020;19:391-403. [Crossref] [PubMed]
- Bemando C, Miranda E, Aryuni M. Machine-learning-based prediction models of coronary heart disease using naïve Bayes and Random Forest Algorithms. 2021 International Conference on Software Engineering & Computer Systems and 4th International Conference on Computational Science and Information Management (ICSECS-ICOCSIM). 2021:232-7.
- Sherly Puspha Annabel L, Sreenidhi S, Vishali N. A novel diagnosis system for parkinson’s disease using K-means clustering and decision tree. In: Sharma H, Gupta MK, Tomar GS, et al. editors. Communication and Intelligent Systems. Singapore: Springer; 2021:607-15.
- Narin A, Kaya C, Pamuk Z. Automatic detection of coronavirus disease (COVID-19) using X-ray images and deep convolutional neural networks. Pattern Anal Appl 2021;24:1207-20. [Crossref] [PubMed]
- The Lancet Digital Health. Can artificial intelligence help create racial equality in the USA? Lancet Digit Health 2021;3:e135. [Crossref] [PubMed]
- Pham Q, Gamble A, Hearn J, et al. The Need for Ethnoracial Equity in Artificial Intelligence for Diabetes Management: Review and Recommendations. J Med Internet Res 2021;23:e22320. [Crossref] [PubMed]
- Haw JS, Shah M, Turbow S, et al. Diabetes Complications in Racial and Ethnic Minority Populations in the USA. Curr Diab Rep 2021;21:2. [Crossref] [PubMed]
- Aggarwal R, Bibbins-Domingo K, Yeh RW, et al. Diabetes Screening by Race and Ethnicity in the United States: Equivalent Body Mass Index and Age Thresholds. Ann Intern Med 2022;175:765-73. [Crossref] [PubMed]
- Nayan M, Salari K, Bozzo A, et al. Predicting survival after radical prostatectomy: Variation of machine learning performance by race. Prostate 2021;81:1355-64. [Crossref] [PubMed]
- Chen IY, Szolovits P, Ghassemi M, Can AI. Help Reduce Disparities in General Medical and Mental Health Care? AMA J Ethics 2019;21:E167-179. [Crossref] [PubMed]
- Allen A, Mataraso S, Siefkas A, et al. A Racially Unbiased, Machine Learning Approach to Prediction of Mortality: Algorithm Development Study. JMIR Public Health Surveill 2020;6:e22400. [Crossref] [PubMed]
- Zavala VA, Bracci PM, Carethers JM, et al. Cancer health disparities in racial/ethnic minorities in the United States. Br J Cancer 2021;124:315-32. [Crossref] [PubMed]
- Iqbal J, Ginsburg O, Rochon PA, et al. Differences in breast cancer stage at diagnosis and cancer-specific survival by race and ethnicity in the United States. JAMA 2015;313:165-73. [Crossref] [PubMed]
- Hirko KA, Rocque G, Reasor E, et al. The impact of race and ethnicity in breast cancer-disparities and implications for precision oncology. BMC Med 2022;20:72. [Crossref] [PubMed]
- Petersen SS, Sarkissyan M, Wu Y, et al. Time to Clinical Follow-up after Abnormal Mammogram among African American and Hispanic Women. J Health Care Poor Underserved 2018;29:448-62. [Crossref] [PubMed]
- Yamoah K, Lee KM, Awasthi S, et al. Racial and Ethnic Disparities in Prostate Cancer Outcomes in the Veterans Affairs Health Care System. JAMA Netw Open 2022;5:e2144027. [Crossref] [PubMed]
- National Cancer Institute. Surveillance, Epidemiology, and End Results Program. Cancer stat facts: Lung and bronchus cancer. Cancer Stat 2018. Available online: https://seer.cancer.gov/statfacts/html/lungb.html
- Siegel RL, Miller KD, Jemal A. Cancer statistics, 2020. CA Cancer J Clin 2020;70:7-30. [Crossref] [PubMed]
- Scarton L, Yoon S, Oh S, et al. Pancreatic Cancer Related Health Disparities: A Commentary. Cancers (Basel) 2018;10:235. [Crossref] [PubMed]
- Rumgay H, Arnold M, Ferlay J, et al. Global burden of primary liver cancer in 2020 and predictions to 2040. J Hepatol 2022;77:1598-606. [Crossref] [PubMed]
- Wisniewski JM, Walker B. Association of Simulated Patient Race/Ethnicity With Scheduling of Primary Care Appointments. JAMA Netw Open 2020;3:e1920010. [Crossref] [PubMed]
- Cataneo JL, Meidl H, Ore AS, et al. The Impact of Limited Language Proficiency in Screening for Breast Cancer. Clin Breast Cancer 2023;23:181-8. [Crossref] [PubMed]
- Cheng I, Tseng C, Wu J, et al. Association between ambient air pollution and breast cancer risk: The multiethnic cohort study. Int J Cancer 2020;146:699-711. [Crossref] [PubMed]
- Pinheiro PS, Callahan KE, Stern MC, et al. Migration from Mexico to the United States: A high-speed cancer transition. Int J Cancer 2018;142:477-88. [Crossref] [PubMed]
- Charan M, Verma AK, Hussain S, et al. Molecular and Cellular Factors Associated with Racial Disparity in Breast Cancer. Int J Mol Sci 2020;21:5936. [Crossref] [PubMed]
- Diaz MJ, Mark I, Rodriguez D, et al. Melanoma Brain Metastases: A Systematic Review of Opportunities for Earlier Detection, Diagnosis, and Treatment. Life (Basel) 2023;13:828. [Crossref] [PubMed]
- Bhatt H, Shah V, Shah K, et al. State-of-the-art machine learning techniques for melanoma skin cancer detection and classification: A comprehensive review. Intell Med 2023;3:180-90.
- Kleinberg G, Diaz MJ, Batchu S, et al. Racial underrepresentation in dermatological datasets leads to biased machine learning models and inequitable healthcare. J Biomed Res (Middlet) 2022;3:42-7. [PubMed]
- Provenzano D, Rao YJ, Goyal S, et al. Radiologist vs. machine learning: A comparison of performance in cancer imaging. 2021 IEEE Applied Imagery Pattern Recognition Workshop (AIPR). 2021:1-10.
- Page MJ, McKenzie JE, Bossuyt PM, et al. The PRISMA 2020 statement: an updated guideline for reporting systematic reviews. BMJ 2021;372: [Crossref] [PubMed]
- Mongan J, Moy L, Kahn CE Jr. Checklist for Artificial Intelligence in Medical Imaging (CLAIM): A Guide for Authors and Reviewers. Radiol Artif Intell 2020;2:e200029. [Crossref] [PubMed]
- Yala A, Lehman C, Schuster T, et al. A Deep Learning Mammography-based Model for Improved Breast Cancer Risk Prediction. Radiology 2019;292:60-6. [Crossref] [PubMed]
- Beig N, Khorrami M, Alilou M, et al. Perinodular and Intranodular Radiomic Features on Lung CT Images Distinguish Adenocarcinomas from Granulomas. Radiology 2019;290:783-92. [Crossref] [PubMed]
- Schaffter T, Buist DSM, Lee CI, et al. Evaluation of Combined Artificial Intelligence and Radiologist Assessment to Interpret Screening Mammograms. JAMA Netw Open 2020;3:e200265. [Crossref] [PubMed]
- Gichoya JW, Banerjee I, Bhimireddy AR, et al. AI recognition of patient race in medical imaging: a modelling study. Lancet Digit Health 2022;4:e406-14. [Crossref] [PubMed]
- Hoeh B, Würnschimmel C, Flammia RS, et al. Effect of chemotherapy in metastatic prostate cancer according to race/ethnicity groups. Prostate 2022;82:676-86. [Crossref] [PubMed]
- Lee W, Nelson R, Akmal Y, et al. Racial and ethnic disparities in outcomes with radiation therapy for rectal adenocarcinoma. Int J Colorectal Dis 2012;27:737-49. [Crossref] [PubMed]
- Lee DJ, Zhao Z, Huang LC, et al. Racial variation in receipt of quality radiation therapy for prostate cancer. Cancer Causes Control 2018;29:895-9. [Crossref] [PubMed]
- Zou J, Schiebinger L. AI can be sexist and racist - it's time to make it fair. Nature 2018;559:324-6. [Crossref] [PubMed]
Cite this article as: Patel R, Provenzano D, Wallington SF, Loew M, Rao YJ, Goyal S. Racial/ethnic reporting differences in cancer literature regarding machine learning vs. a radiologist: a systematic review and meta-analysis. J Med Artif Intell 2023;6:25.