GPT-4 and medical image analysis: strengths, weaknesses and future directions
Introduction
ChatGPT (Generative Pre-trained Transformer) is an artificial intelligence (AI) language model developed by OpenAI (San Francisco, USA) (1). It is based on the Transformer architecture, which was introduced in 2017 by Vaswani et al. (2) which is a type of neural network that is particularly well-suited for processing sequential data, such as text. The usages for ChatGPT continue to grow, and new applications of the langue learning model continue to be found (3,4). During the training process, the network is fed huge amounts of text data and learns to identify these patterns and relationships in order to generate natural language (2). Following this process, a sophisticated fine-tuning process occurs including the use of human researchers to provide prompts and responses (5). Reinforcement learning from human feedback (RLHP) is also conducted, where human graders rank GPT responses to a set of queries (5).
GPT-4 is the newest version of ChatGPT released on March 14, 2023 and has been reported to have a broader knowledge base as well as improved problem-solving ability (Figure 1). GPT-4 has also been reported to be less easy to fool, less likely to reply to disallowed requests, and is capable of processing 8 times more words.
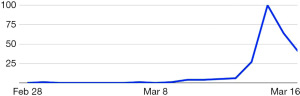
Out of the new features introduced in GPT-4, the most important feature may be its new ability to analyze images. However, the ability to browse the web has been suspended since 3rd July, 2023 and GPT-4 currently does not interpret images. This study was performed during the introductory phase when GPT-4 had browsing capabilities. If performed accurately, GPT-4 image analysis may be able to revolutionize patient care, by leading to more accurate diagnoses. This could help doctors to diagnose and treat patients quickly and accurately, especially in areas where access to medical professionals may be limited (3). To examine GPT-4’s image diagnostic ability, we provided it with a variety of common medical imaging modalities: from chest X-rays, magnetic resonance images (MRI), to optical coherence tomography (OCT) images.
We examined the performance of GPT-4 to interpret a chest X-ray (Figure 2). Chest X-ray interpretation is a common and important task that is crucial for patient care. GPT-4 was able to accurately identify that this was a community-acquired pneumonia, and described an opacity in the lower right lung field that is consistent with pneumonia. GPT-4 also picked up on a more subtle sign, that was not even described in the original manuscript the image was re-printed from, of right heart border blurring. However, without hearing the patient’s history, differentiating community-acquired vs. hospital-acquired pneumonia could not be made by GPT-4 with any degree of certainty.

We then chose an MRI image with an arrow to determine if having some minimal labelling would be useful for GPT-4’s image analysis (Figure 3). Impressively, GPT-4 was capable of identifying this image as an MRI scan in the sagittal plane, with T1 post-contrast showing a clival chondroma. GPT-4 also described that a chondroma would appear as a round, well-circumscribed and homogenously enhancing lesion on a T1-weighted image.
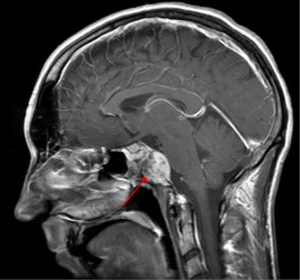
However, GPT-4, may be supplementing its image analysis with context from the publication that the image was featured in or by using underlying image meta-data. When we tried two different links for the same image, the results were vastly different (Figure 4). The ResearchGate link was likely richer in meta-data, and when this information was not provided, GPT-4 provided an extremely inaccurate response, responding that the provided brain MRI was a photomicrograph of a breast duct. This shows a major flaw in the image analysis ability of GPT-4, and the large language learning model may be overly reliant on text input.
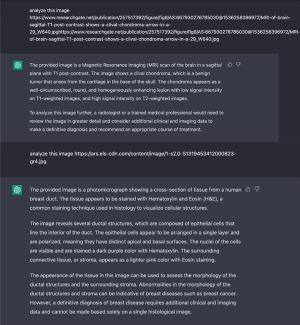
Finally, we examined GPT-4’s ability to analyze fundus photography and OCT images (Figure 5). GPT-4 incorrectly described the provided image as a close-up photograph of the eye, and stated that it showed the various layers of the cornea, including stroma, epithelium and endothelium. No reference to the ophthalmic condition (dominant optic atrophy) was made by GPT-4, meaning it went undetected.
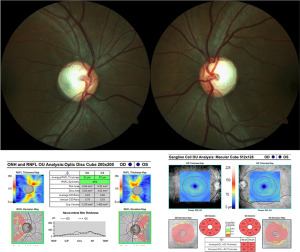
Future directions
The performance of GPT-4’s image analysis could be improved by further training on a dataset of medical images and corresponding diagnoses in order to learn to identify patterns and relationships between the images and their associated conditions. In addition to its many capabilities, GPT-4 also has a specific set of limitations (9). For instance, GPT-4 bases its predictions on the patterns it discovers in the training data. This means that the model might not perform well when provided with data that is fundamentally different from the data it was trained on or when faced with a brand-new challenge (10). An AI model can remain only as unbiased as the data that it is trained on, so it is essential that diverse datasets are used to minimize the potential of AI bias. Additionally, the cut off training date for ChatGPT is September 2021. As medical knowledge and clinical guidelines are constantly evolving, this can lead to the generation of outdated recommendations.
Moreover, GPT-4 could struggle to understand the context of a conversation, which could lead to misconceptions or wrong responses. Information provided by GPT-4 is not always reliable or accurate, especially when discussing complex or technical topics, therefore reference to other sources of information is necessary (11). Due to the black box nature of AI models, interpretation of GPT-4 outputs must be made with caution to ensure that no errors have been made (12). Particularly in healthcare delivery and medicine, where policies and procedures are frequently revised, GPT-4 algorithms comments can be out-of-date or incorrect. Lastly GPT-4 may collect and preserve user information including contact information and browsing history. As a result, GPT-4 users should be aware of its potential privacy concerns. It should also be noted that Google recently released an AI chatbot, Bard, that has image analysis capabilities. Meta is also developing its own large language model (LLM), LlaMa 2, which may soon also provide image analysis capabilities. Future work will be required to determine which LLM possesses the best image analysis capabilities. As LLMs become more prevalent, it is also important to implements mechanisms to ensure equitability and accountability. Open-source codes could allow for routine oversight, increased accountability for data generation standards, and facilitate LLM development (13). In conclusion, although significant advancements and further research is still required, the future of automated medical image analysis is highly promising.
Acknowledgments
Funding: None.
Footnote
Peer Review File: Available at https://jmai.amegroups.com/article/view/10.21037/jmai-23-94/prf
Conflicts of Interest: All authors have completed the ICMJE uniform disclosure form (available at https://jmai.amegroups.com/article/view/10.21037/jmai-23-94/coif). The authors have no conflicts of interest to declare.
Ethical Statement: The authors are accountable for all aspects of the work in ensuring that questions related to the accuracy or integrity of any part of the work are appropriately investigated and resolved. This article does not contain any studies with human participants performed by any of the authors. IRB approval and informed consent are waived as no patients were involved.
Open Access Statement: This is an Open Access article distributed in accordance with the Creative Commons Attribution-NonCommercial-NoDerivs 4.0 International License (CC BY-NC-ND 4.0), which permits the non-commercial replication and distribution of the article with the strict proviso that no changes or edits are made and the original work is properly cited (including links to both the formal publication through the relevant DOI and the license). See: https://creativecommons.org/licenses/by-nc-nd/4.0/.
References
- Introducing ChatGPT. Accessed March 15, 2023. Available online: https://openai.com/blog/chatgpt
- Vaswani A, Shazeer N, Parmar N, et al. Attention is All you Need. In: Advances in Neural Information Processing Systems. Vol 30. Curran Associates, Inc.; 2017. Accessed March 15, 2023. Available online: https://proceedings.neurips.cc/paper/2017/hash/3f5ee243547dee91fbd053c1c4a845aa-Abstract.html
- Waisberg E, Ong J, Masalkhi M, et al. GPT-4: a new era of artificial intelligence in medicine. Ir J Med Sci 2023;192:3197-200. [Crossref] [PubMed]
- Waisberg E, Ong J, Masalkhi M, et al. Text-to-image artificial intelligence to aid clinicians in perceiving unique neuro-ophthalmic visual phenomena. Ir J Med Sci 2023;192:3139-42. [Crossref] [PubMed]
- Thirunavukarasu AJ, Ting DSJ, Elangovan K, et al. Large language models in medicine. Nat Med 2023;29:1930-40. [Crossref] [PubMed]
- Larcher R, Pantel A, Arnaud E, et al. First report of cavitary pneumonia due to community-acquired Acinetobacter pittii, study of virulence and overview of pathogenesis and treatment. BMC Infect Dis 2017;17:477. [Crossref] [PubMed]
- Kim JD, Hashemi N, Gelman R, et al. Neuroimaging in ophthalmology. Saudi J Ophthalmol 2012;26:401-7. [Crossref] [PubMed]
- Waisberg E, Micieli JA. Neuro-Ophthalmological Optic Nerve Cupping: An Overview. Eye Brain 2021;13:255-68. [Crossref] [PubMed]
- Alser M, Waisberg E. Concerns with the usage of ChatGPT in Academia and Medicine: A viewpoint. Am J Med Open 2023;9:100036. [Crossref]
- Waisberg E, Ong J, Kamran SA, et al. Transfer learning as an AI-based solution to address limited datasets in space medicine. Life Sci Space Res (Amst) 2023;36:36-8. [Crossref] [PubMed]
- Waisberg E, Ong J, Masalkhi M, et al. Generative Pre-Trained Transformers (GPT) and Space Health: A Potential Frontier in Astronaut Health During Exploration Missions. Prehosp Disaster Med 2023;38:532-6. [Crossref] [PubMed]
- Waisberg E, Ong J, Paladugu P, et al. Challenges of Artificial Intelligence in Space Medicine. Space Science & Technology 2022;2022:9852872. [Crossref]
- Paladugu PS, Ong J, Nelson N, et al. Generative Adversarial Networks in Medicine: Important Considerations for this Emerging Innovation in Artificial Intelligence. Ann Biomed Eng 2023;51:2130-42. [Crossref] [PubMed]
Cite this article as: Waisberg E, Ong J, Masalkhi M, Zaman N, Sarker P, Lee AG, Tavakkoli A. GPT-4 and medical image analysis: strengths, weaknesses and future directions. J Med Artif Intell 2023;6:29.