Exploring the capabilities and limitations of large language models in nuclear medicine knowledge with primary focus on GPT-3.5, GPT-4 and Google Bard
Introduction
A large language model (LLM) is a type of artificial intelligence that can generate human-like text. This technology has the potential to transform our approach to accessing and processing online information. Prior to the advent of LLMs, internet users predominantly relied on search engines, which were designed to retrieve pre-existing information through one-time queries. In contrast, LLMs possess the ability to generate novel textual content by summarizing information and engaging in ongoing dialogues with users, thereby providing a more intuitive user experience compared to conventional search engines (1).
Two prominent applications, ChatGPT and Bard, have emerged among LLMs. ChatGPT serves as a frontend interface of GPT-3.5 and GPT-4, both of which are LLMs developed by OpenAI Inc. (San Francisco, USA). In contrast, Bard, developed by Google LLC (Mountain View, USA), is built upon the LLMs called Pathways Language Models (PaLM) and Gemini. Both of these applications have witnessed widespread adoption, appealing to both casual users and technology enthusiasts alike (2).
LLMs have demonstrated vast usefulness across various medical specialties (3), such as their ability to answer questions in ophthalmology (4) and address queries related to lung cancer (5). In the field of nuclear medicine, ChatGPT was shown to be able to provide adequate advice and satisfactory explanation of fluorine-18 fluorodeoxyglucose ([18F]FDG) positron emission tomography/computed tomography (PET/CT) reports (6). However, another study suggested that the GPT-3.5-based ChatGPT could not respond correctly enough to pass the national nuclear medicine specialty examination (7).
Newer LLMs have been shown to be superior in its accuracy compared to their older counterparts (8). The evaluation of the accuracy of the newer GPT-4-based ChatGPT and the freely available Bard in the field of nuclear medicine remains underexplored. Therefore, we conducted this pilot study to assess the accuracy of the responses from GPT-3.5, GPT-4, and Bard to medical questions related to nuclear medicine.
Methods
One author (S.V.), who was a nuclear medicine physician with 3 years of teaching experience in a medical school, curated a set of 20 questions related to nuclear medicine at the level necessary for medical students and general practitioners. The questions were in the four-choice single best answer format to ensure quantifiable measurement of the accuracy of LLMs’ responses and were then verified by the other investigator (K.K.), who was a nuclear medicine physician in a medical school with over 20 years of teaching experience. The questions covered foundational knowledge in nuclear medicine and varied in complexity according to the Bloom’s cognitive taxonomy (9) (Figure 1). The abbreviations were presented in these questions without their corresponding full spellings to emulate how health care professionals are expected to interact with LLMs (Table 1).
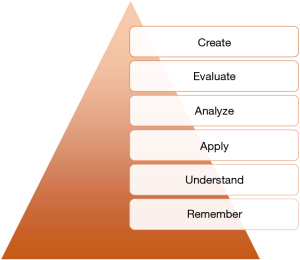
Table 1
Abbreviation | Full spellings |
---|---|
BMD | Bone mineral density |
CSF | Cerebrospinal fluid |
CXR | Chest X-ray |
DTPA | Diethylenetriaminepentaacetic acid |
DISIDA | Diisopropyl-iminodiacetic acid |
FDG | Fluorodeoxyglucose |
F-18 | Flurorine-18 |
GI | Gastrointestinal |
GFR | Glomerular filtration rate |
I-131 | Iodine-131 |
LVEF | Left ventricular ejection fraction |
MIBG | Meta-iodobenzylguanidine |
MIBI | Methoxyisobutylisonitrile |
MUGA | Multigated acquisition |
PET/CT | Positron emission tomography/computed tomography |
RBC | Red blood cell |
NaI | Sodium iodide |
Tc-99m | Technetium-99m |
TSH | Thyroid stimulating hormone |
WBC | White blood cell |
WHO | World Health Organization |
The versions of LLMs we tested in this experiment were GPT-3.5 (Aug 3, 2023 version), GPT-4 (Oct 29, 2023 version), and Bard (Aug 28, 2023 version). The questions were posted into the chatbot interface of each LLM once, and their responses were recorded. To avoid interference from previous prompts, each question was presented to the LLMs in separate chats.
The accuracy of the LLMs were examined using correct response rates and were expressed in as counts and percentages. Formal comparisons between paired proportions were conducted using McNemar’s test on the Jupyter software interface with the R Stats package. A P value of <0.05 was considered statistically significant. This was a non-human subject experiment and our institute’s ethics committee certified this study for an exemption (COE No. 062/2023).
Results
The 20 questions used in our experiment included questions in the remember level, the understand level, and the apply level. Their contents covered multiple topics in nuclear medicine (Table 2).
Table 2
Question number | Input queries | Bloom’s modified taxonomy levels | Content areas | Expected answers | GPT-3.5 answer | GPT-4 answers | Bard answer |
---|---|---|---|---|---|---|---|
1. | Which procedure is NOT performed in the nuclear medicine department? | Remember | Introduction to nuclear medicine | D | D | D | D |
A PET | |||||||
B SPECT | |||||||
C Thyroid uptake | |||||||
D External beam radiotherapy | |||||||
2. | What is dual energy DXA used for? | − | Bone mineral density | B | B | B | B |
A Diagnosis of bone tumor | |||||||
B Diagnosis of osteoporosis | |||||||
C Diagnosis of bone infection | |||||||
D Diagnosis of bone metastasis | |||||||
3. | According to the WHO, the T-score of which area is NOT used for diagnostic classification of osteoporosis? | Remember | Bone mineral density | D | D | D | D |
A Total hip | |||||||
B Femoral neck | |||||||
C Lumbar spine | |||||||
D Ward’s triangle | |||||||
4. | Which of the following is NOT is the advantage of renal function assessment with renal scintigraphy over creatinine clearance? | Understand | Nephrology | D | D | D | D |
A Can assess split function between right and left kidney | |||||||
B Can visualize rough morphology of the kidneys | |||||||
C Can assess outflow obstructions | |||||||
D More accurate GFR | |||||||
5. | Which radiotracer is used in parathyroid scintigraphy? | Remember | Oncology | B | B | B | B |
A I-131 MIBG | |||||||
B Tc-99m MIBI | |||||||
C Tc-99m DTPA | |||||||
D I-131 NaI | |||||||
6. | Which tumor is most likely to cause false negative on [18F]FDG PET/CT? | Remember | Oncology | D | B* | D | D |
A Hodgkin lymphoma | |||||||
B Metastatic lung cancer | |||||||
C Metastatic cervical cancer | |||||||
D Metastatic prostate cancer | |||||||
7. | Which pattern on ventilation/perfusion (V/Q) scan is most suggestive of pulmonary embolism? | Apply | Vascular and pulmonology | A | A | A | A |
A Mismatched perfusion defect | |||||||
B Mismatched ventilation defect | |||||||
C Matched ventilation/perfusion defect | |||||||
D Perfusion defect with air-space consolidation on CXR | |||||||
8. | Which pattern on of lymphoscintigraphy (lymphatic scan) is suggestive of lymphatic obstruction? | Apply | Vascular and pulmonology | A | A | A | A |
A Dermal backflow | |||||||
B Visible blood pool activity | |||||||
C Visible deep lymphatic vessels | |||||||
D Tracer leakage at injection site | |||||||
9. | Which thyroid tumor is NOT treated with radioiodine? | Remember | Thyroidology | B | B | B | B |
A Follicular thyroid carcinoma | |||||||
B Medullary thyroid carcinoma | |||||||
C Follicular variant papillary thyroid carcinoma | |||||||
D Conventional variant papillary thyroid carcinoma | |||||||
10. | Which thyrotoxicosis CANNOT be treated with radioiodine? | Remember | Thyroidology | A | A | A | A |
A Hashitoxicosis | |||||||
B Graves’ disease | |||||||
C Toxic adenoma | |||||||
D Toxic multinodular goiter | |||||||
11. | How should levothyroxine (LT4) be adjusted in patient with differentiated thyroid carcinoma after radioiodine treatment? | Apply | Thyroidology | C | C | C | C |
A To keep serum TSH within the normal range | |||||||
B To keep serum TSH above the normal range | |||||||
C To keep serum TSH within specific ranges recommended by ATA, which may be below the ranges for normal population | |||||||
D To keep serum TSH within specific ranges recommended by ATA, which may be above the ranges for normal population | |||||||
12. | Which of the following is NOT an advantage of Tc-99m MIBI myocardial perfusion scan? | Understand | Cardiology | D | D | D | D |
A Not nephrotoxic | |||||||
B Compatible to exercise stress | |||||||
C Compatible to pharmacologic stress | |||||||
D Ability to assess valvular complications | |||||||
13. | In which setting does MUGA scan is preferred for LVEF calculation over echocardiography? | Apply | Cardiology | C | D* | C | C |
A Valvular heart disease | |||||||
B Congenital heart disease | |||||||
C Follow up after cardiotoxic drug | |||||||
D Immediate assessment after myocardial infarction | |||||||
14. | Tc-99m RBC GI bleeding scan is the preferred modality in: | Apply | Gastroenterology | D | D | A* | D |
A Slow bleeding | |||||||
B Variceal bleeding | |||||||
C Hypovolemic shock | |||||||
D Intermittent bleeding | |||||||
15. | Which tracer is used for Meckel scan? | Remember | Gastroenterology | D | D | D | C* |
A Tc-99m RBC | |||||||
B Tc-99m WBC | |||||||
C Tc-99m sulfur colloid | |||||||
D Tc-99m pertechnetate | |||||||
16. | What is the interpretation of Tc-99m DISIDA scan when tracer can be excreted into bowel of patients with neonatal hepatitis? | Apply | Hepatology | C | C | C | A* |
A Impaired liver function is likely | |||||||
B Impaired cardiac function is likely | |||||||
C Biliary atresia can be totally excluded | |||||||
D Neonatal hepatitis can be totally excluded | |||||||
17. | [18F]FDG PET brain is used in: | Remember | Neurology | D | D | D | D |
A Alzheimer’s disease | |||||||
B Atypical parkinsonism | |||||||
C Epilepsy | |||||||
D All of the above | |||||||
18. | Tc-99m DTPA CSF scan is used in: | Remember | Neurology | D | D | D | D |
A Brain death | |||||||
B CSF leakage | |||||||
C CSF shunt dysfunction | |||||||
D All of the above | |||||||
19. | Bone scan is mostly used for which indication: | Understand | Osteology | B | B | B | B |
A Measurement of BMD | |||||||
B Diagnosis of bone metastasis | |||||||
C Diagnosis of compression fracture | |||||||
D Differentiate type of bone tumors | |||||||
20. | Which tumor is most likely associated with false negative bone metastasis on bone scan? | Understand | Osteology | D | B* | D | D |
A Lung cancer | |||||||
B Breast cancer | |||||||
C Prostate cancer | |||||||
D Renal cell carcinoma |
Since all three LLMs examined in this study only support plain text input, the input queries in the table are displayed as plain text without any formatting. The asterisks in the table indicates the answer that deviated from the expected answers that the investigators considered to be the correct best answer. LLM, large language model; PET, positron emission tomography; SPECT, single-photon emission computed tomography; DXA, X-ray absorptiometry; WHO, World Health Organization; GFR, glomerular filtration rate; I-131, iodine-131; MIBG, meta-iodobenzylguanidine; MIBI, methoxyisobutylisonitrile; DTPA, diethylenetriaminepentaacetic acid; NaI, sodium iodide; [18F]FDG, fluorine-18 fluorodeoxyglucose; CT, computed tomography; CXR, chest X-ray; ATA, American Thyroid Association; TSH, thyroid stimulating hormone; MUGA, multigated acquisition; LVEF, left ventricular ejection fraction; RBC, red blood cell; GI, gastrointestinal; WBC, white blood cell; DISIDA, diisopropyl-iminodiacetic acid; CSF, cerebrospinal fluid; BMD, bone mineral density.
All three LLMs responded to all questions without declining. Out of 20 questions, there were 15 questions that all three LLMs responded to correctly. GPT-3.5, GPT-4, and Bard provided correct responses to 17 [correct response rate 85.0%, 95% confidence interval (CI): 62.1–96.8%], 19 (correct response rate 95.0%, 95% CI: 75.1–100%), and 18 questions (correct response rate 90.0%, 95% CI: 68.3–98.8%), respectively (Figure 2).
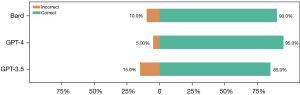
The statistical analyses did not show significantly different correct response rates between GPT-3.5 and GPT-4 [McNemar’s χ2(1)=1, P=0.317], between GPT-3.5 and Bard [McNemar’s χ2(1)=0.2, P=0.655], and between GPT-4 and Bard [McNemar’s χ2(1)=0.333, P=0.564].
GPT-3.5 answered three questions incorrectly: one at the remember level, one at the understand’ level, and one at the apply level. First, the question, “Which tumor is most likely to cause false negative on [18F]FDG PET/CT?” was incorrectly responded to by GPT-3.5. In its response, GPT-3.5 stated that metastatic prostate cancer generally showed high FDG uptake and was unlikely to cause a false negative on [18F]FDG PET/CT scans. However, this statement was incorrect because most prostate cancer does not show high FDG uptake. Another question incorrectly responded to by GPT-3.5 was the question “In which setting does MUGA scan is preferred for LVEF calculation over echocardiography?”. The investigators expected the answer “Follow up after cardiotoxic drug”, owing to the low inter-observer variability of the multigated acquisition (MUGA) scan, but GPT-3.5 chose the incorrect “Immediate assessment after myocardial infarction” without providing supporting reasons. The question, “Which tumor is most likely associated with false negative bone metastasis on bone scan?”, was the last question incorrectly responded to by GPT-3.5. In response to this question, GPT-3.5 generated a highly incorrect statement. It claimed that osteoblastic metastasis caused by breast cancer leads to false negative bone scans, even though osteoblastic metastasis is typically not associated with false negative bone scans.
GPT-4 answered one apply level question incorrectly. It opted for “Slow bleeding” as the answer for the question “Tc-99m RBC GI bleeding scan is the preferred modality in:” contrary to the investigators’ expected answer of “Intermittent bleeding”. While the technetium-99m red blood cell gastrointestinal (Tc-99m RBC GI) bleeding scan can detect slow GI bleeding with a rate as low as 0.5 milliliters per minute, CT angiography can also detect similarly slow bleeding rates with the advantage of better anatomical localization. However, the Tc-99m GI bleed scan can be performed over a longer period of time, making it beneficial for patients with intermittent bleeding (10). In this question, GPT-4 did not provide reasons why it did not choose “Intermittent bleeding” as the correct answer.
Bard answered one remember level question and one apply level question incorrectly. It chose “Tc-99m sulfur colloid” instead of the correct “Tc-99m pertechnetate” as the answer to the question, “Which tracer is used for Meckel scan?”. Bard incorrectly stated that Tc-99m sulfur colloid was used to detect small bowel cells present in the Meckel diverticulum. In reality, Meckel scan utilizes Tc-99m pertechnetate to detect the stomach cells that are present in the Meckel diverticulum. In response to the question “What is the interpretation of Tc-99m DISIDA scan when tracer can be excreted into bowel of patients with neonatal hepatitis?”, Bard correctly stated that it could imply biliary atresia can be excluded, which was also the correct answer choice. However, Bard self-contradictorily opted for the choice “Impaired liver function is likely”.
Discussion
Our experiment found that LLMs could select the correct choices to nuclear medicine-related single best answer questions with correct response rates of 85.0–95.0%. Although the correct response rate of GPT-4 was higher than that of Bard and the correct response rate of Bard was higher than that of GPT-3.5, these differences were not statistically significant.
The correct response rates of the LLMs in our experiment were higher than many of the previous studies. GPT-3.5, GPT-4, and Bard could choose the correct answer choices to the questions from the official board examination of the Japan Radiology Society with correct response rate of 40.8%, 65.0%, and 38.8%, respectively. The subgroup analysis from the same study showed the correct response rates of 40.0%, 93.3%, and 26.7% for GPT-3.5, GPT-4, and Bard in questions related to nuclear medicine (11). Similarly, the correct response rates of GPT-3.5 to the National Polish Nuclear Medicine Specialty Exam was 56% (7). Our correct response rates were also higher than the correct responses rates for open-end questions related to lung cancer, in which GPT-3.5 and Bard achieved correct response rates of 70.8% and 51.7%, respectively (5).
There could be many reasons that might explain the higher correct response rates achieved by our experiment. Firstly, because most LLMs have been in constant development, the accuracy of the responses generated by LLMs may have improved during the time between the experiments conducted by previous investigators and ours, with the more recent version a more accurate response (8). Secondly, the effect of different difficulties cannot be totally excluded as our experiments focused on questions relevant to medical students and general practitioners, in contrast to the questions focusing on radiology and nuclear medicine specialist investigated in some other studies (7,11). However, this hypothesis was not supported by the fact that the questions answered to incorrectly by LLMs varied in the levels of the Bloom’s cognitive taxonomy.
Although being among the first experiments focusing on the accuracy of LLMs in responding to questions related to nuclear medicine, particularly regarding to the knowledge level of medical students and general practitioners, there are a few limitations to our investigation. Firstly, being a pilot study, the number of questions were few and arbitrary, which could limit the statistical power of this study. Secondly, the use of multiple-choice questions may not reflect the real interactions between users and LLMs. Therefore, we encourage further investigation into the accuracy of LLMs on open-ended questions related to nuclear medicine, as well as the comprehensive assessment of potential risk and safeguard measures of LLM usages. Thirdly, we did not systematically modify or paraphrase the prompts to assess the consistency of the responses generated by LLMs. Lastly, due to the continuously evolving nature of LLMs, we recommend regularly reassessing their accuracy to stay up-to-date with the latest iterations, including both the ones we have studied and those that will be released in the future.
Conclusions
In conclusion, although the investigated LLMs were able to achieve high response rates, they could not answer all questions correctly. This highlights that LLMs should not be used as the sole resource for medical information, particularly in the field of nuclear medicine. Sufficient caution should be exercised when using LLMs as tools for information gathering to ensure the accuracy of the information retrieved.
Acknowledgments
Funding: None.
Footnote
Peer Review File: Available at https://jmai.amegroups.com/article/view/10.21037/jmai-23-180/prf
Conflicts of Interest: Both authors have completed the ICMJE uniform disclosure form (available at https://jmai.amegroups.com/article/view/10.21037/jmai-23-180/coif). Both authors were supported by the Learning Innovation Center of Chulalongkorn University. The authors have no other conflicts of interest to declare.
Ethical Statement: The authors are accountable for all aspects of the work in ensuring that questions related to the accuracy or integrity of any part of the work are appropriately investigated and resolved. This was a non-human subject experiment and our institute’s ethics committee certified this study for an exemption (COE No. 062/2023).
Open Access Statement: This is an Open Access article distributed in accordance with the Creative Commons Attribution-NonCommercial-NoDerivs 4.0 International License (CC BY-NC-ND 4.0), which permits the non-commercial replication and distribution of the article with the strict proviso that no changes or edits are made and the original work is properly cited (including links to both the formal publication through the relevant DOI and the license). See: https://creativecommons.org/licenses/by-nc-nd/4.0/.
References
- Currie GM. Academic integrity and artificial intelligence: is ChatGPT hype, hero or heresy? Semin Nucl Med 2023;53:719-30. [Crossref] [PubMed]
- Meskó B, Topol EJ. The imperative for regulatory oversight of large language models (or generative AI) in healthcare. NPJ Digit Med 2023;6:120. [Crossref] [PubMed]
- Thirunavukarasu AJ, Ting DSJ, Elangovan K, et al. Large language models in medicine. Nat Med 2023;29:1930-40. [Crossref] [PubMed]
- Biswas S, Logan NS, Davies LN, et al. Assessing the utility of ChatGPT as an artificial intelligence-based large language model for information to answer questions on myopia. Ophthalmic Physiol Opt 2023;43:1562-70. [Crossref] [PubMed]
- Rahsepar AA, Tavakoli N, Kim GHJ, et al. How AI Responds to Common Lung Cancer Questions: ChatGPT vs Google Bard. Radiology 2023;307:e230922. [Crossref] [PubMed]
- Rogasch JMM, Metzger G, Preisler M, et al. ChatGPT: Can You Prepare My Patients for [18F]FDG PET/CT and Explain My Reports? J Nucl Med 2023;64:1876-9. [Crossref] [PubMed]
- Kufel J, Bielówka M, Rojek M, et al. Assessing ChatGPT’s performance in national nuclear medicine specialty examination: An evaluative analysis. Iranian Journal of Nuclear Medicine 2024;32:60-5.
- Rizzo MG, Cai N, Constantinescu D. The performance of ChatGPT on orthopaedic in-service training exams: A comparative study of the GPT-3.5 turbo and GPT-4 models in orthopaedic education. J Orthop 2024;50:70-5. [Crossref] [PubMed]
- Mirbahai L, Adie J. Applying the utility index to review single best answer questions in medical education assessment. Arch Epid Pub Health 2020. doi:
10.15761/AEPH.1000113 .10.15761/AEPH.1000113 - Wortman JR, Landman W, Fulwadhva UP, et al. CT angiography for acute gastrointestinal bleeding: what the radiologist needs to know. Br J Radiol 2017;90:20170076. [Crossref] [PubMed]
- Toyama Y, Harigai A, Abe M, et al. Performance evaluation of ChatGPT, GPT-4, and Bard on the official board examination of the Japan Radiology Society. Jpn J Radiol 2024;42:201-7. [Crossref] [PubMed]
Cite this article as: Vachatimanont S, Kingpetch K. Exploring the capabilities and limitations of large language models in nuclear medicine knowledge with primary focus on GPT-3.5, GPT-4 and Google Bard. J Med Artif Intell 2024;7:5.