Analysis of factors influencing maternal mortality and newborn health—a machine learning approach
Highlight box
Key findings
• Blocks like Umsning, Khliehriat, Mairang, Umling, and Laskein have high maternal mortality rate (MMR) due to intrauterine contraceptive device (IUCD) procedures.
• Nongstoin block has the maximum MMR due to pregnancy complications, and the highest IMR due to IUCD insertion complications.
• The high IMR found in the Umling, Umsning, and Mairang blocks correlated to pregnancy complications, hypertension, and hemoglobin level <7 g/dL.
What is known and what is new?
• To the best of the author’s knowledge, no systematic effort on MMR and infant mortality rate (IMR) was reported utilizing the advanced geospatial-artificial intelligence (Geo-AI) and machine learning approach in Meghalaya state.
• Data-driven insights are deduced using the advanced Geo-AI approach identifies the trends, gaps, and factors contributing to IMR and MMR to suggest interventions, prediction, and preventive measures.
What is the implication, and what should change now?
• More digital records for a better understanding of the changing patterns with time are required to be maintained.
Introduction
Maternal mortality rate (MMR) and infant mortality rate (IMR) serve as a benchmark of general community health while addressing social, economic, and developmental challenges. However, maternal and infant deaths are highly responsive to environmental and healthcare conditions, unlike old-age deaths. World Health Organization (WHO) reported that 810 women die every day worldwide due to childbirth and pregnancy-related complications, with the majority (94 %) of maternal deaths occurring in low and lower-income countries (1). Artificial intelligence (AI) and machine learning (ML) technology are emerging as promising tools for revolutionizing the healthcare system (2,3) and have great potential to reduce maternal deaths (4,5). Moreover, pregnancy-related risks can be reduced by predicting the complications and further preventive measures to save millions of mothers’ and infants’ lives (6). Therefore, the power of AI/ML is not only helping in overcoming the health challenges by identifying significant gaps in health infrastructure and targeting maternal and child health to attain global sustainable development goals (SDGs).
Further, AI has the advantage of analyzing large health datasets, especially when statistical methods are ineffective. The k-means algorithm is the most extensively studied clustering algorithm and is generally effective in producing good results. The k-means grouping algorithm was initially proposed by MacQueen in 1967 (7) and later enhanced by Hartigan and Wong (8). By using the k-means algorithm the researcher has segmented a large-scale heterogeneous rehabilitation client population into more homogeneous subgroups thereby improving the understanding of client characteristics and enabling appropriate targeting of rehabilitation services for home care patients (9). Some of the applications of clustering algorithms in the medical domain include medical diagnosis (10,11), biological data analysis (12,13), medical image segmentation (14,15), patient database management (16,17), and hospital resource management (18). Besides k-means, Khan et al. (19) reviewed AI methods for encouraging maternal and neonatal health in middle and low-income nations. AI integration with digital technologies emerged as a practical assistive tool in various healthcare facilities, including maternal and neonatal health. Another report (20) investigated a clustering algorithm k-means on the data set, applied factor analysis on the original data, and then the same clustering algorithm was applied to obtain the factor scores. A comparison of the effectiveness of clustering on the original data with that on the factor scores was found to be successful.
India is observant of the most significant number of maternal deaths worldwide, accounting for more than 20% of maternal mortality globally (21). The MMR and IMR are alarmingly high in the state of Meghalaya, with 197 deaths per 100,000 deliveries and 34 deaths per 1,000 live births (22), and require immediate healthcare intervention. Increasing maternal mortality in the state is one of the significant health concerns for the state government.
Furthermore, the State Health Policy [2021] outlines essential public health services aligned with the objectives of the WHO’s SDGs. Despite these efforts, there has been a lack of systematic research on MMR and IMR analysis utilizing geospatial-AI (Geo-AI) modeling within the state of Meghalaya India. Thus, to bridge this research gap, AI/ML techniques are employed to assess the availability and accessibility of human resources, identify gaps in the healthcare infrastructure, and scrutinize factors influencing IMR and MMR across Meghalaya’s 11 districts. The study employs applied research and geospatial analysis to derive data-driven insights and identify trends. Through an examination of gaps and factors contributing to maternal and infant mortality, the research proposes targeted interventions and preventive measures. The findings underscore the importance of enhancing healthcare facility accessibility, ultimately reducing maternal and infant deaths. These outcomes not only inform immediate interventions but also provide a roadmap for future research, emphasizing the need for policymakers to prioritize improvements in healthcare access.
Methods
Study area
Meghalaya meaning the “abode of clouds”, is one of the North Eastern states of India and is predominantly a hilly terrain. Meghalaya state has a geographical area of 22,429 km2 divided into 11 districts and further into 39 blocks (Figure 1). The state has about 170 health centers, mainly community health centers (CHCs), primary health centers (PHCs), and sub-health centers (SHCs).
Data
Meghalaya’s State Health Policy [2021] has identified that MMR and IMR are alarmingly high, with 197 deaths per 100,000 deliveries and 34 deaths per 1,000 live births which requires healthcare intervention (22). The parameters for each block considering total maternal deaths, total infant deaths (1–12 months), total infant deaths up to 4 weeks, infant deaths within 24 hours of birth, and stillbirths are studied.
Methodology
The methodology involves a systematic approach encompassing data aggregation, cleaning, and assimilation, followed by block-level clustering in Meghalaya based on raw data to delineate regions with varying risk levels. Subsequent data analysis aims to unveil underlying factors contributing to maternal and infant deaths and identify regions where these critical factors exert a dominant influence. These regions are then analyzed for overlap with previously determined risk clusters, offering a comprehensive understanding of the spatial distribution of maternal and infant mortality factors in Meghalaya. Techniques include data preprocessing, basic statistical analysis (multicollinearity removal, and dimensionality reduction), k-means clustering, feature engineering with a random forest machine learning model (RFMLM), exploratory factor analysis (EFA), and geospatial AI fusion for valuable insights. Figure 2 visually depicts the sequential steps to elucidate critical factors impacting MMR and IMR. The following section provides an in-depth explanation of the methodology.
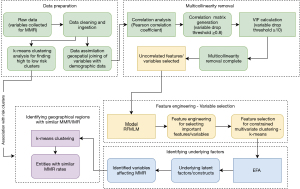
Data pre-processing
This step entails the collection of raw data pertaining to MMR and IMR, followed by manual data cleaning and ingestion processes. The data are then assimilated by geospatially joining variables with demographic data within a 10 km radius of the reported factors. The major demographic variables considered the socioeconomic status, which includes 32 factors, including the working and nonworking status of females, availability of shelter, drinking water quality, and literacy. The assimilated data are then ready for analysis.
Risk clusters
Leveraging information from the raw dataset that highlights maximum maternal and infant deaths, a k-means clustering analysis categorizes Meghalaya into five distinct risk clusters at the block level, ranging from high to low risk. Following this clustering, a detailed data analysis is conducted to uncover the underlying factors contributing to these deaths. In the process of deducing high-risk clusters, key variables such as total maternal deaths, total infant deaths (1–12 months), total infant deaths up to 4 weeks, still births, infant deaths within 24 hours (1 to 23 hours) of birth are considered. The risk clusters are shown in Figure 3. The risk clusters have been directly generated from the raw data, ensuring that all clusters exhibit comparable rates of maternal and infant deaths. This implies that high-risk clusters feature both maximum infant and maternal deaths, and this pattern extends to the remaining clusters. The final clusters, derived through a combination of statistical analysis and AI/ML techniques, have been distinctly formulated for IMR and MMR. Subsequently, these previously identified risk clusters are systematically compared with the ones determined through the analytical process.
Multicollinearity removal
To understand the interdependencies within the dataset and to use the data for subsequent analyses, like feature selection and modeling, the Pearson correlation analysis is applied to examine the pairwise relationships between various factors related to maternal health and infant health. This method enables the identification of variables that may exhibit significant correlations with the dependent variables, offering insights into potential determinants or contributing factors. A threshold of 0.8 is considered and variables with multicollinearity more than 0.8 are dropped from the analysis.
To ascertain that none of the independent variables are correlated among themselves, additionally, a variance inflation factor (VIF) check with a threshold of 10 is performed to assess the extent of multicollinearity among predictors. Variables with VIF values exceeding the threshold of 10 are flagged for further consideration in multicollinearity removal. After removing multicollinearity, uncorrelated features are selected to form a refined dataset.
RFMLM
In the application of the RFMLM to the pre-processed dataset, the following steps are systematically followed for feature engineering, focusing on identifying crucial features contributing to maternal and infant mortality:
- Data splitting: the dataset is partitioned into training and testing sets, with a split ratio of 70% to 30% (70% for training and 30% for the testing set). This allows the model to be trained on one subset and evaluated for performance on another.
- Model training: the RFMLM is trained using the training dataset, wherein the model constructs multiple decision trees and amalgamates their predictions, ensuring robustness and accuracy.
- Variable importance assessment: to gauge the importance of each variable in predicting MMR and IMR, the model calculates the Gini impurity or mean decrease in Gini for each feature. This process reveals their contribution to the model’s predictive performance.
- Model evaluation: the RFMLM’s performance on the testing dataset is assessed using metrics such as accuracy, precision, and recall, ensuring the model generalizes well to unseen data.
The model is fine-tuned through hyperparameter optimization, conducted via grid-search to optimize performance. After extracting the most important variables using the RFMLM, EFA is applied to identify underlying latent factors and constructs within the dataset. This step unveils hidden patterns and relationships among variables, enhancing the understanding of complex factors influencing maternal mortality.
Factor analysis
EFA is performed using variables obtained from RFMLM feature engineering. Factor analysis, a multivariate statistical tool, describes variability among observed, correlated variables in terms of a potentially lower number of unobserved variables called factors. The EFA process involves three main steps:
- Assessment of data suitability: to determine the strength of relationships among items, a coefficient of correlation >0.7 in the correlation matrix is sought. Two statistical measures assess the factorability of the data: Kaiser-Meyer-Olkin (KMO) measure of sampling adequacy and Bartlett’s test of sphericity (23-25).
- KMO measure: KMO evaluates the suitability of data for factor analysis. It is performed on 65 variables out of 452 in the study, assessing the variance proportion among all variables. The following formula is used for KMO estimation (23): where R is [rij] is correlation matrix; U is [uij] is the partial covariance matrix; and Σ is summation. The variance proportion can be interpreted as per Table 1.
- Bartlett’s test of sphericity: this test examines the null hypothesis that the original correlation matrix is an identity matrix, indicating variables are unrelated. A significant value (<0.05) suggests that factor analysis is worthwhile. To measure the overall relationship between the variables, the determinant of the correlation matrix |R| is calculated. Under H0, |R|=1; if the variables are highly correlated, then |R|≈0. The Bartlett’s test of sphericity is given by: where p is number of variables; n is total sample size; and R is correlation matrix.
- Orthogonal rotations: constrain factors to be uncorrelated, often favored but may be unrealistic in certain cases.
- Oblique rotations: permit factors to be correlated, often producing solutions with a simpler structure.
Table 1
KMO value | Interpretation |
---|---|
>0.9 & ≤1 | Excellent |
>0.8 & ≤0.9 | Good |
>0.7 & ≤0.8 | Moderate |
>0.6 & ≤0.7 | Reasonable |
>0.5 & ≤0.6 | Acceptable |
≤0.5 | Unacceptable |
KMO, Kaiser-Meyer-Olkin.
These steps collectively refine the dataset and uncover latent factors, enhancing the understanding of complex relationships among variables influencing maternal mortality.
Geo-AI and clustering data analysis
Following the extraction of pertinent variables through EFA, the subsequent step involves leveraging these factors to create factor clusters. This process begins by spatially joining the factors with the respective blocks in Meghalaya. This spatial association links the identified factors to specific geographic regions, allowing for a localized understanding of the factors influencing maternal and infant mortality.
To further delineate and visualize these factor clusters, k-means spatial clustering is applied. This spatial clustering method considers both the geographical proximity and factor similarities among different blocks. By utilizing this technique, the study aims to generate clusters that highlight spatial concentrations of common factors associated with maternal mortality.
Once the factor clusters are established through k-means spatial clustering, mapping techniques are employed to visually represent these clusters across Meghalaya. This spatial representation enhances the interpretability of the factor clusters and provides valuable insights into the geographical distribution of factors contributing to maternal mortality.
In the final phase of the analysis, the outcomes from k-means spatial clustering are systematically compared with the previously identified high to low-risk clusters. This comparative assessment aims to ascertain if there are any overlaps between the factor clusters and the risk clusters in specific blocks. The identification of overlaps or discrepancies informs the understanding of how the spatial distribution of factors aligns with the pre-determined risk levels.
This comprehensive approach, integrating EFA-derived factors with spatial clustering techniques, contributes to a nuanced understanding of the geographic patterns and underlying factors influencing maternal mortality in Meghalaya. The analysis not only identifies critical factors but also provides a spatial context, facilitating targeted interventions and policy decisions tailored to the specific needs of different regions.
Results
In our focused analysis of health data in Meghalaya, we begin with geo-tagging, data ingestion, and cleaning, followed by spatially joining health variables with demographic data. Using information on maximum maternal and infant deaths, k-means clustering categorizes Meghalaya into five block-level risk clusters. This section presents the results obtained from the clustering analysis.
Further, the results obtained from the analysis that identifies factors contributing to these deaths, employing a multicollinearity removal procedure with Pearson correlation and VIF calculations are presented (Tables 2,3). The results from feature engineering with the RFMLM reveal critical variables impacting MMR and IMR. EFA extracts hidden factors, and a multivariate spatial clustering algorithm identifies regions with similar MMR and IMR, offering a precise spatial understanding of maternal and infant mortality factors in 39 blocks of Meghalaya.
Table 2
Factors | Correlation with maternal death | Remarks | Max. no. of factors in blocks |
---|---|---|---|
Factor 1: birth control measures | Negative | This may result in a reduction in MMR | Umsning, Khliehriat, Mairang, Umling, Laskein |
Factor 2: pregnancy complications management | Negative | This may result in a reduction in MMR | Nongstoin, Mawkyrwat, Khliehriat, Mairang |
Factor 3: pregnant women medication | Negative | This may result in a reduction in MMR | Mawshynrut, Resubelpara, Selsella, Umsning, Dambo |
Factor 4: IUCD complications | Positive | This may increase MMR | Umling |
Factor 5: post-immunization complications | Positive | This may increase MMR | Khliehriat, Resubelpara, Dambo, Mylliem |
MMR, maternal mortality rate; IUCD, intrauterine contraceptive device.
Table 3
Factors | Correlation with infant death | Remarks | Max. no. of factors in blocks |
---|---|---|---|
Factor 1: pregnancy complications and institutional delivery | Negative | May result in reduction of IMR | Umling, Umsning, Mairang |
Factor 2: pregnant women medications | Negative | May result in reduction of IMR | Mawshynrut, Resubelpara, Selsella, Umsning |
Factor 3: IUCD complications and tests | Positive | May contribute to increase in IMR | Nongstoin |
IMR, infant mortality rate; IUCD, intrauterine contraceptive device.
Clustering analysis and risk groups
Based on the k-means clustering analyses, the blocks are divided into five risk groups. These risk groups with blocks are discussed in Table 4 (high-risk clusters) and Table 5 (medium and low clusters). The blocks affected by these clusters are shown in Figures 4-8.
- High-risk Cluster 2 identifies three blocks: Mawkyrwat, Resubelpera, and Thadlaskein;
- High-risk Cluster 4 identifies three blocks: Mairang, Selsella, and Umsning;
- High-risk Cluster 5 identifies one block: Nongstoin;
- Medium-risk Cluster 3 identifies 12 blocks: Amlarem, Betasing, Dalu, Khliehriat, Mawphlang, Mawryngkneng, Mawshynrut, Mawsynram, Mylliem, Pynursla, Rongara, and Zikzak;
- Low-risk Cluster 1 identifies 19 blocks: Baghmara, Chokpot, Dadenggiri, Dambo Rongjeng, Gambegre, Gasuapara, Jirang, Kharkutta, Laskein, Mawkynrew, Mawthadraishan, Ranikor, Rongram, Saipung, Samanda, Shella Bholaganj, Songsak, Tikrikilla, and Umling.
Table 4
Cluster # | Blocks in this cluster | Risk level |
---|---|---|
Cluster 2 | Mawkyrwat, Resubelpara, Thadlaskein | High risk |
Cluster 4 | Mairang, Selsella, Umsning | High risk |
Cluster 5 | Nongstoin | High risk |
Table 5
Cluster # | Blocks in this cluster | Risk level |
---|---|---|
Cluster 3 | Amlarem, Betasing, Dalu, Khliehriat Block, Mawphlang, Mawryngkneng, Mawshynrut, Mawsynram, Mylliem, Pynursla, Rongara, Zikzak | Medium risk |
Cluster 1 | Baghmara, Chokpot, Dadenggiri, Dambo Rongjeng, Gambegre, Gasuapara, Jirang, Kharkutta, Laskein, Mawkynrew, Mawthadraishan, Ranikor, Rongram, Saipung, Samanda, Shella Bholaganj, Songsak, Tikrikilla, Umling | Low risk |
Multicollinearity removal
A grayscale correlation heatmap using 47 health variables is generated as shown in Figure 9. The light colors represent a high correlation while the dark colors show a lesser correlation. After correlation analysis and using VIF to drop variables with values greater than 10, only 22 variables for IMR and 15 variables for MMR are considered critical.
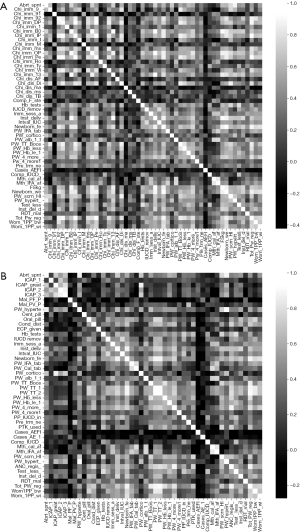
RFMLM
The results for feature engineering used for the MMR and IMR data are shown in Figure 10A,10B.
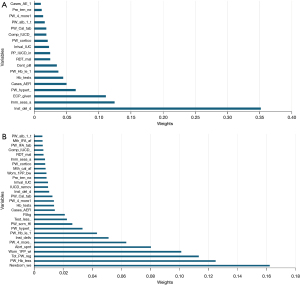
Factor analysis and Geo-AI clustering
The figures illustrating the blocks influenced by the variables identified in factor analysis are displayed in Figures 11-15 for MMR and Figure 16-18 for IMR. The factor list and its associated variables are also presented in Tables 6,7, respectively.
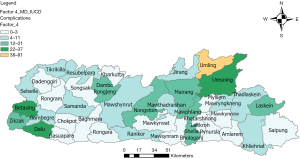
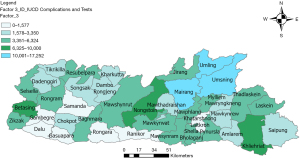
Table 6
Variables | Factor 1 loading | Factor 2 loading | Factor 3 loading | Factor 4 loading | Factor 5 loading | Maximum number of factors in blocks |
---|---|---|---|---|---|---|
Factor 1: birth control measures | Umsning, Khliehriat, Mairang, Umling, Laskein | |||||
Number of IUCD removals | 0.709892 | −0.02248 | 0.382489 | 0.236342 | −0.25317 | |
Number of interval IUCD insertions (excluding PPIUCD and PAIUCD) | 0.807591 | 0.090084 | 0.333343 | 0.07982 | −0.09212 | |
Number of PTKs used | 0.73131 | 0.176941 | −0.04164 | 0.058143 | 0.256302 | |
Number of post-partum (within 48 hours of delivery) IUCD insertions | 0.62747 | 0.207689 | 0.182982 | 0.409731 | −0.14948 | |
Factor 2: pregnancy complications | Nongstoin, Mawkyrwat, Khliehriat, Mairang | |||||
Out of the new cases of PW with hypertension detected, issues were managed at the institution | 0.202142 | 0.808275 | 0.016919 | 0.047005 | 0.353141 | |
Number of PW given ANC in preterm labour | −0.01615 | 0.876252 | 0.042867 | −0.05362 | −0.11695 | |
Factor 3: pregnant women medication and tests | Mawshynrut, Resubelpara, Selsella, Umsning, Dambo | |||||
Number of PW given one albendazole tablet after 1st trimester | 0.093967 | 0.136145 | 0.957651 | −0.04206 | 0.033984 | |
RDT conducted for Malaria | 0.138174 | 0.05344 | 0.591389 | 0.212093 | −0.03787 | |
Number of PW given 360 calcium tablets | 0.143528 | 0.017516 | 0.591309 | 0.088593 | 0.108195 | |
Factor 4: IUCD complications | Umling | |||||
Number of complications following IUCD insertion | 0.197137 | −0.04762 | 0.13651 | 0.977832 | −0.04277 | |
Factor 5: post-immunization complications | Khliehriat, Resubelpara, Dambo, Mylliem | |||||
Number of cases of AEFI—others | −0.12945 | 0.02649 | 0.019265 | −0.0569 | 0.59812 |
IUCD, intrauterine contraceptive device; PPIUCD, post-partum IUCD; PAIUCD, post-abortion IUCD; PTK, pregnancy test kit; PW, pregnant women; ANC, antenatal corticosteroid; RDT, rapid diagnostic test; AEFI, adverse event following immunization.
Table 7
Cases | Variables | Factor 1 loading | Factor 2 loading | Factor 3 loading | Maximum number of factors in blocks |
---|---|---|---|---|---|
Factor 1: pregnancy complications and institutional delivery | Umling, Umsning, Mairang | ||||
Out of the new cases of PW with hypertension detected, cases managed at the institution | PW_hypert_ | 0.877449 | 0.075792 | −0.0229 | |
Number of PW given ANC in preterm labor | PW_cortico | 0.772027 | −0.00388 | −0.13355 | |
Number of PW having Hb level <7 g/dL (tested cases) | PW_Hb_le_1 | 0.609993 | 0.134303 | 0.361387 | |
Out of total institutional deliveries number of women discharged within 48 hours of delivery | Inst_del_d | 0.605226 | 0.163821 | 0.288005 | |
Factor 2: medications | Mawshynrut, Resubelpara, Selsella, Umsning | ||||
Number of PW given one albendazole tablet after 1st trimester | PW_alb_1_t | 0.16348 | 0.82392 | 0.119643 | |
RDT conducted for Malaria | RDT_mal | 0.068757 | 0.735475 | 0.206234 | |
Number of PW given 360 calcium tablets | PW_Cal_tab | 0.058979 | 0.521607 | 0.202004 | |
Factor 3: IUCD complications and tests | Nongstoin | ||||
Number of IUCD removals | IUCD_remov | 0.097354 | 0.282324 | 0.827954 | |
Number of interval IUCD insertions (excluding PPIUCD and PAIUCD) | Intval_IUC | 0.246919 | 0.313996 | 0.640061 | |
Number of complications following IUCD insertion | Comp_IUCD_ | 0.048351 | 0.148302 | 0.559373 | |
Number of Hb tests conducted | Hb_tests | 0.394967 | 0.272348 | 0.545639 |
PW, pregnant women; ANC, antenatal corticosteroid; Hb, hemoglobin; RDT, rapid diagnostic test; IUCD, intrauterine contraceptive device; PPIUCD, post-partum IUCD; PAIUCD, post-abortion IUCD.
Discussion
Underlying factors responsible for maternal deaths
The core suggestions to reduce maternal mortality include (I) birth control measures or family planning with related reproductive health services; (II) pregnancy complications management or skilled care during pregnancy and childbirth; (III) medication of pregnant women with timely emergency obstetric care; and (IV) intrauterine contraceptive device (IUCD) and post-immunization complications focus on reducing maternal mortality shown in Table 6.
Factor 1: birth control [number of IUCD removals, number of intervals IUCD insertions, number of pregnancy test kits (PTKs) used, and number of post-partum (within 48 hours of delivery) IUCD insertions]. Geospatial and statistical analyses reveal that all these MMR due to Factor 1 are high in Umsning, Khliehriat, Mairang, Umling, and Laskein blocks of Meghalaya, out of which Umnsning and Mairang are also a part of the list of high-risk cluster and positively linked with a high number of maternal deaths in respective blocks and spatial representation of the impact by Factor 1 is shown in Figure 11. It is suggested that proper family planning measures in these blocks may result in a reduction in MMR. Contraceptive use is advised as it also lowers the risk of maternal mortality per birth, as measured by the MMR, by preventing high-risk births, that is, births to women who are “too young” or “too old”, birth intervals that are “too close”, and high-parity births (i.e., “too many”).
Factor 2: pregnancy complication management [pregnant women with hypertension detected, managed at the institution, and number of pregnant women given antenatal corticosteroid (ANC) in preterm labor]. Maternal deaths related to the management of pregnancy complications are high in the Nongstoin, Mawkyrwat, Khliehriat, and Mairang blocks, as shown in Figure 12. Nongstoin block records the highest number of maternal deaths due to pregnancy complications.
Factor 3: pregnant women medication [number of pregnant women given one albendazole tablet after 1st trimester, rapid diagnostic test (RDT) conducted for Malaria, and number of pregnant women given 360 calcium tablets]. Lack of medications and tests administered to pregnant women also contribute to high MMR as seen in Mawshynrut, Resubelpara, Selsella, Umsning, and Dambo blocks shown in Figure 13.
Factors 4 and 5: IUCD and post-immunization complications (high number of IUCD and post-immunization complications). Higher MMR in Umling block due to IUCD complications and Khliehriat, Resubelpara, Dambo, and Mylliem blocks due to post-immunization complications are observed as shown in Figures 14,15.
Maternal deaths intervention
Table 2 discusses the factors correlated with the high MMR in the various blocks in Meghalaya and based on the above-mentioned underlying factors priority-wise interventions are required to reduce MMR. The maximum number of factors affect the mentioned blocks and hence priority intervention is required in Khlieriat (MMR affected by Factors 1, 2, and 5), Umsning (MMR affected by Factors 1 and 3), Mairang, Umling (MMR affected by Factors 1 and 4), and Resubelpara blocks (MMR affected by Factors 3 and 5), where more than one factor is contributing to high MMR.
Underlying factors responsible for infant deaths
The core suggestions to reduce infant mortality include (I) pregnancy complications and institutional delivery; (II) medications; and (III) IUCD complications and tests focused on reducing infant mortality shown in Table 7.
Factor 1: pregnancy complications and institutional delivery [variables are pregnant women with hypertension detected, cases managed at the institution, number of pregnant women having hemoglobin (Hb) level <7 g/dL (tested case), and out of total institutional deliveries number of women discharged within 48 hours of delivery]. As shown, Umling, Umsning, and Mairang blocks are the areas with high infant deaths contributed by these factors, as shown in Figure 16.
Factor 2: medications (variables are the number of pregnant women given one albendazole tablet after 1st trimester, RDT conducted for Malaria, and the number of pregnant women given 360 calcium tablets). Mawshynrut, Resubelpara, Selsella, and Umsning blocks, are the areas with high infant deaths contributed by factors (Figure 17).
Factor 3: IUCD complications and tests (variables are the numbers of IUCD removals, number of intervals IUCD insertions, number of complications following IUCD insertions, and number of Hb tests conducted). Nongstoin block has high infant deaths due to these contributing factors (Figure 18).
Infant deaths (within 24 hours) intervention
Table 3 discusses the blocks and the correlation with the above-mentioned factors which contribute to high IMR in the state and it is suggested that priority-based interventions are required in the listed blocks based on underlying factors. The maximum number of factors affect the mentioned blocks and hence priority intervention is required in Umsning (IMR affected by Factors 1 and 2), where more than 1 factor is contributing to high IMR.
Hence, the Umsning block needs an immediate intervention for more than one factor contributing to both high MMR and IMR.
Blocks commonly identified in cluster analysis and factor analysis
The blocks with high MMR and IMR determined from factor analysis are subsequently examined for any overlap with the blocks previously determined from risk cluster analysis, providing a comprehensive understanding of the spatial distribution of maternal and infant mortality factors in Meghalaya. This leads to the priority-wise intervention required in the blocks starting from high risk to low risk and addressing of the factors leading to the MMR and IMR in the respective blocks.
Blocks identified for factor analysis in factors correlated to MMR and risk cluster overlap:
- Nongstoin, Mairang, Selsella, Umsning, Mawkyrwat, and Resubelpera blocks identified during factor analysis lie in high-risk cluster groups (Clusters 1, 2, and 3);
- Khliehriat, Mawshynrut, and Mylliem blocks identified during factor analysis lie in medium-risk cluster groups (Cluster 4);
- Laskein, Mawkynrew, Dambo, and Umling blocks identified during factor analysis lie in low-risk cluster groups (Cluster 5).
Blocks identified for factor analysis in factors correlated to IMR and risk cluster overlap:
- Mairang, Selsella, Umsning, Resubelpera, and Nongstoin blocks identified during factor analysis lie in high-risk cluster groups (Clusters 1, 2, and 3);
- Mawshynrut blocks identified during factor analysis lie in medium-risk cluster groups (Cluster 4);
- Umling blocks identified during factor analysis lie in low-risk cluster groups (Cluster 5).
Conclusions
In conclusion, this study systematically investigated the IMR and MMR in Meghalaya, India, focusing on 11 districts. Historical datasets were analyzed using advanced statistical techniques and AI/ML models. The method involved geotagging and preprocessing of raw health datasets for streamlined ingestion. Health variables were spatially joined with demographic data, offering a comprehensive view of the region’s health landscape and its relation to demographic factors. By applying k-means clustering analysis to reported Infant and Maternal deaths, five distinct risk clusters were categorized. A detailed analysis identified critical factors influencing IMR and MMR, leading to the creation of clusters based on these determinants. The dataset underwent cleaning to eliminate collinearity, and the RFMLM was applied for feature engineering, providing a deeper exploration of variables impacting IMR and MMR. EFA identified key factors responsible for IMR and MMR, which were then used to prepare cluster maps illustrating the dominance of these critical factors in various blocks of Meghalaya. Comparative analysis of these regions with risk clusters provided insights into potential reasons for observed patterns. This exercise enhances understanding of the health conditions contributing to maternal and infant deaths in Meghalaya. Observations highlight specific regions, such as Umsning, Khliehriat, Mairang, Umling, and Laskein, with elevated MMR due to Birth control complications, pregnant women with hypertension, and pregnant women given ANC in preterm labor, IUCD procedures and lack of proper medications. Notably, the Nongstoin block exhibited the highest maternal deaths due to pregnancy complications and the highest IMR associated with pregnant women with hypertension, pregnant women having Hb level <7 g/dL, and IUCD insertion complications. Maternal mortality, preventable with timely medication, underscores the importance of addressing medication-related issues in these blocks. Implementing frequent Accredited Social Health Activist (ASHA) visits for health advice and regular health camps in challenging accessibility areas can reduce maternal mortality. For high IMR in Umling, Umsning, and Mairang blocks linked to pregnancy complications, hypertension, and low Hb cases, immediate interventions are imperative. Particularly in hilly terrains with travel restrictions, such as Nongstoin, Umling, and Umsning blocks, tailored strategies must be devised to address unique challenges. This study aims to provide valuable insights for policymakers and healthcare practitioners to formulate effective strategies for reducing IMR and MMR in Meghalaya.
Acknowledgments
The authors are highly thankful to the Department of Health and Family Welfare, Government of Meghalaya (India) for the support from time to time for smooth completion of work.
Funding: None.
Footnote
Data Sharing Statement: Available at https://jmai.amegroups.com/article/view/10.21037/jmai-23-107/dss
Peer Review File: Available at https://jmai.amegroups.com/article/view/10.21037/jmai-23-107/prf
Conflicts of Interest: All authors have completed the ICMJE uniform disclosure form (available at https://jmai.amegroups.com/article/view/10.21037/jmai-23-107/coif). All authors are employees of Deepspatial Inc. The authors have no other conflicts of interest to declare.
Ethical Statement: The authors are accountable for all aspects of the work in ensuring that questions related to the accuracy or integrity of any part of the work are appropriately investigated and resolved. Institutional Review Board (IRB) approval and informed consent are not suitable for this study as it does not involve any human experiment.
Open Access Statement: This is an Open Access article distributed in accordance with the Creative Commons Attribution-NonCommercial-NoDerivs 4.0 International License (CC BY-NC-ND 4.0), which permits the non-commercial replication and distribution of the article with the strict proviso that no changes or edits are made and the original work is properly cited (including links to both the formal publication through the relevant DOI and the license). See: https://creativecommons.org/licenses/by-nc-nd/4.0/.
References
- UNFPA, World Health Organization, UNICEF, World Bank Group, the United Nations Population Division. Trends in maternal mortality 2000 to 2017: Estimates by WHO, UNICEF, UNFPA, World Bank Group and the United Nations Population Division. 2019. Accessed 10 Jan 2021. Available online: https://www.unfpa.org/featured-publication/trends-maternal-mortality-2000-2017
- Topol EJ. High-performance medicine: the convergence of human and artificial intelligence. Nat Med 2019;25:44-56. [Crossref] [PubMed]
- VoPham T. Emerging trends in geospatial artificial intelligence (geoAI): potential applications for environmental epidemiology. Environ Health 2018;17:40. [Crossref] [PubMed]
- World Health Organization. Maternal deaths decline slowly with vast inequalities worldwide. 2019. Accessed 10 Jan 2021. Available online: https://www.who.int/news/item/19-09-2019-maternal-deaths-decline-slowly-with-vast-inequalities-worldwide
- World Health Organization. Maternal mortality. 2023. Accessed 10 July 2023. Available online: https://www.who.int/news-room/fact-sheets/detail/maternal-mortality
- Islam MN, Mustafina SN, Mahmud T, et al. Machine learning to predict pregnancy outcomes: a systematic review, synthesizing framework and future research agenda. BMC Pregnancy Childbirth 2022;22:348. [Crossref] [PubMed]
- MacQueen J. Some methods for classification and analysis of multivariate observations. In: Le Cam Lm, Neyman J, editors. Proceedings of the Fifth Berkeley Symposium on Mathematical Statistics and Probability. Berkeley and Los Angeles: University of California Press; 1967;1:281-97.
- Hartigan JA, Wong MA. Algorithm AS 136: A k-means clustering algorithm. J R Stat Soc Ser C Appl Stat 1979;28:100-8.
- Armstrong JJ, Zhu M, Hirdes JP, et al. K-means cluster analysis of rehabilitation service users in the Home Health Care System of Ontario: examining the heterogeneity of a complex geriatric population. Arch Phys Med Rehabil 2012;93:2198-205. [Crossref] [PubMed]
- Nithya N, Duraiswamy K, Gomathy P. A survey on clustering techniques in medical diagnosis. Int J Comput Sci Trends Technol 2013;1:17-23.
- Paul R, Hoque ASML. Clustering medical data to predict the likelihood of diseases. 2010 Fifth International Conference on Digital Information Management (ICDIM). IEEE; 2010:44-9.
- Li X, Zhu F. On clustering algorithms for biological data. Engineering 2013;5:549-52. [Crossref]
- Wiwie C, Baumbach J, Röttger R. Comparing the performance of biomedical clustering methods. Nat Methods 2015;12:1033-8. [Crossref] [PubMed]
- Ma Z, Tavares JMRS, Jorge RMN. A review on the current segmentation algorithms for medical images. International Conference on Imaging Theory and Applications. SciTePress; 2009:135-40.
- Dhanachandra N, Manglem K, Chanu YJ. Image segmentation using K-means clustering algorithm and subtractive clustering algorithm. Procedia Comput Sci 2015;54:764-71. [Crossref]
- Narmadha D. Survey of clustering algorithms for categorization of patient records in healthcare. Indian Journal of Science and Technology 2016; [Crossref]
- da Veiga FA. Structure discovery in medical databases: a conceptual clustering approach. Artif Intell Med 1996;8:473-91. [Crossref] [PubMed]
- Dilts D, Khamalah J, Plotkin A. Using cluster analysis for medical resource decision making. Med Decis Making 1995;15:333-47. [Crossref] [PubMed]
- Khan M, Khurshid M, Vatsa M, et al. On AI Approaches for Promoting Maternal and Neonatal Health in Low Resource Settings: A Review. Front Public Health 2022;10:880034. [Crossref] [PubMed]
- Anand S, Padmanabham P, Govardhan A. Application of factor analysis to k-means clustering algorithm on transportation data. Int J Comput Appl 2014;95:40-6. [Crossref]
- Mavalankar D, Vora K, Prakasamma M. Achieving Millennium Development Goal 5: is India serious? Bull World Health Organ 2008;86:243-243A. [Crossref] [PubMed]
.Meghalaya Health Policy 2021 . Available online: https://meghealth.gov.in/docs/Meghalaya%20Health%20Policy%202021.pdf- Shrestha N. Factor analysis as a tool for survey analysis. Am J Appl Math Stat 2021;9:4-11. [Crossref]
- Arora V, Kharb M, Sinha LN, et al. Service quality dimensions regarding maternal and child health-care provided to the beneficiaries attending a Nagrik Hospital Rohtak Haryana. Int J Med Sci Public Health 2019;8:1039-45. [Crossref]
- Coakes SJ, Ong C. SPSS version 18.0 for Windows. Version 18.0. Melbourne: John Wiley & Sons Australia Ltd.; 2011.
Cite this article as: Zaman B, Sharma A, Garg J, Ram C, Kushwah R, Muradia R. Analysis of factors influencing maternal mortality and newborn health—a machine learning approach. J Med Artif Intell 2024;7:1.