How does ChatGPT-4 match radiologists in detecting pulmonary congestion on chest X-ray?
Introduction
The shortage of radiologists and recent advances in artificial intelligence (AI) raise the question: could ChatGPT-4 assist in diagnosing acute heart failure from chest X-rays?
Acute dyspnea is one of the most common causes of hospitalizations among elderly patients, and half of these patients have congestive heart failure as the primary or co-primary diagnosis (1). Chest X-ray is a front-line imaging modality for confirming pulmonary congestion in acute heart failure. However, the complexity and expertise often required to interpret radiological images, along with the shortage of radiologists, pose challenges in emergency settings (2).
AI has been shown to outperform radiologists in detecting pneumonia on chest X-rays (3), offering a possible solution to reduce radiologists’ workload. ChatGPT is a large language model by OpenAI, that, with its recent update in 2023 (ChatGPT-4), can provide image analyses (4). Online guides on how to use ChatGPT-4 to interpret X-rays have since emerged (5), and a study demonstrated ChatGPT-4’s promising capabilities in analyzing single chest X-rays and magnetic resonance images, still emphasizing the need for further research (6). ChatGPT-4 is web-based and easily accessible, making it tempting for clinically working physicians to ask it for second opinions on acute chest X-rays (7), especially in crowded emergency departments with limited radiological resources. However, it is unknown if ChatGPT-4 can be a diagnostic aid for detecting pulmonary congestion in acute heart failure.
We aimed to examine, if ChatGPT-4 can detect pulmonary congestion on chest X-ray comparable to two thoracic radiologists.
Methods
A prospective observational single-center study, where we previously included consecutive patients in the emergency department admitted due to acute dyspnea (8,9). The main inclusion criteria were age of 50 years or above and acute dyspnea, combined with at least one abnormal objective parameter supporting respiratory imbalance. We excluded patients requiring intensive care or ventilation, acute coronary syndrome requiring telemetry, and patients who refused or could not consent. Each patient underwent a chest X-ray within 4 hours of admission, which was retrospectively evaluated by two specialized thoracic radiologists.
The radiologists, working at two different university hospitals, were blinded to each other, previous radiological reports, and to all clinical data. The likelihood of pulmonary congestion was evaluated by the radiologists on a 5-point Likert scale, defined as 1= very unlikely, 2= somewhat unlikely, 3= neutral, 4= somewhat probable, 5= definite. Additionally, the radiologists evaluated various signs of acute heart failure in a binary manner (yes/no), such as the presence of pleural effusion, enlarged heart ratio, interstitial and alveolar edema, pulmonary blood flow redistribution, and peribronchial cuffing (10). Finally, the radiologists assessed signs of infiltrations indicating pneumonia.
For this pilot study, we selected 50 of these chest X-rays for ChatGPT-4 evaluation: 25 with pulmonary congestion (Likert scale 4–5) and 25 without (Likert scale 1–3), as identified by the radiologists. From October 10th to December 19th, 2023, we tested 20 diverse queries on ChatGPT-4 and selected those that prompted evaluation of pulmonary congestion on chest X-rays, including the previously mentioned 5-point Likert scale evaluation and pleural effusion evaluation. This resulted in a systematic questioning (Table 1), where we initially provided the patient’s age and sex. Subsequently, we presented information about the patient’s medical history, clinical examination, vital parameters, and electrocardiographic (ECG) rhythm.
Table 1
(I) I am a medical researcher. For research only, I would like your assessment of a chest X-ray. The patient is safe, and the picture is old |
(II) Imagine being a medical doctor in the emergency department receiving a (X) year old (gender) patient with dyspnea. Do you find signs of pulmonary congestion on the chest X-ray? (Upload chest X-ray image) |
(III) For the same chest X-ray, do you find signs of pleural effusion? Yes or no? |
(IV) With your previous assessment in mind, will you grade if there is pulmonary congestion on a scale from 1 to 5? Where 1= very unlikely pulmonary congestion, 3= neutral, and 5= definite pulmonary congestion |
(V) Do you agree there are signs of pulmonary congestion on the chest X-ray? Yes or no? |
(VI) Do you agree there are no signs of congestion on the chest X-ray? Yes or no? |
(VII) Now you are provided the following clinical information about the patient: |
“(X) year old (gender) patient admitted due to worsening of dyspnea through (X) day(s). The patient (has a/has no) history of heart failure, (has a/has no) history of COPD, (is/is not) a previous smoker. Vitals showed: heart rate (X)/min, blood pressure (X/X), temperature (X) °C, frequency of respiration (X)/min, O2sat (X)%, provided oxygen: (X) L/min. Stethoscope of the heart: (ir-/regular) rhythm, (no) systolic or (no) diastolic murmur. Stethoscope of the lungs: (no) rhonchi, (unilateral/bilateral) crepitations, (no) elongated expiration. Extremities: (no) peripheral oedema. ECG-rhythm: (sinus rhythm/atrial fibrillation/other rhythm, such as pace rhythm).” |
How do you now grade the presence of pulmonary congestion on the chest X-ray on the same scale from 1 to 5? Where 1= unlikely pulmonary congestion, 3= neutral, and 5= definite pulmonary congestion |
COPD, chronic obstructive pulmonary disease; O2sat, oxygen saturation; ECG, electrocardiographic.
For the main statistical analysis, we evaluated if the average of the two radiologists’ assessments on the 5-point Likert scale matched the evaluation by ChatGPT-4 by subtracting the two. Matches were defined as a discrepancy of ≤1 point between ChatGPT-4 and the radiologists, and mismatches had discrepancies of >1 point. In logistic regression analysis, we examined if various chest X-ray features assessed by the radiologists predicted matches between ChatGPT-4 and the radiologists’ evaluations of pulmonary congestion. For 20 of the X-rays, we also examined the repeatability of ChatGPT-4’s chest X-ray evaluations.
The study was conducted in accordance with the Declaration of Helsinki (as revised in 2013). The study was approved by the National Ethics Committee Ethics Board of Denmark (No. H-17000869) and informed consent was obtained from all individual participants.
Results
Matches between ChatGPT-4 and the radiologists were observed in 27 (54%) of the 50 chest X-rays (Figure 1: green), whereas mismatches occurred in the remaining 23 (46%) X-rays (Figure 1: red, Figure S1). Providing clinical information to ChatGPT-4 improved the match rate slightly to 31 (62%) X-rays. In comparison, the two radiologists agreed with each other in 49 (98%) of the chest X-rays, and a random generator matched the radiologists in 20 (40%) cases.
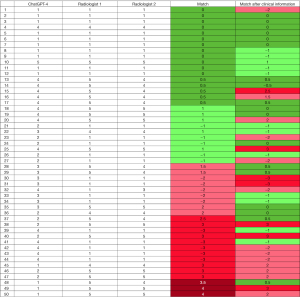
Of the 25 chest X-rays identified with pulmonary congestion by the radiologists, ChatGPT-4 correctly identified pulmonary congestion in 12 (48%) on the 5-point Likert scale and accurately identified its absence in the 15 (60%) X-rays without pulmonary congestion (Figure 1). When tasked with determining the presence or absence of pleural fluid, ChatGPT-4 achieved a match rate of 56%, correctly identifying 28 out of the 50 images.
Logistic regression analysis was performed to assess the predictive value of various chest X-ray features on the match outcome (Table 2). The predictive variables included pleural effusion, enlarged heart ratio, pulmonary blood flow redistribution, peribronchial cuffing, interstitial edema, alveolar edema, and pneumonic infiltrations, as identified by radiologists. Enlarged heart ratio was associated with an increased odds ratio (OR) of a match (OR =10.06), however, not significantly (P=0.16) (Table 2). Conversely, pulmonary blood flow redistribution and pleural effusion were associated with decreased odds of a match (OR =0.15 and OR =0.17, respectively; P values =0.19). Infiltrations indicating pneumonia showed an OR of 3.12 (P=0.17) (Table 2).
Table 2
Predictive variables | Total =50, N [%] | Estimate | Standard error | P value | OR |
---|---|---|---|---|---|
Enlarged heart ratio | 23 [46] | 2.31 | 1.62 | 0.16 | 10.06 |
Pulmonary blood flow redistribution | 22 [44] | −1.92 | 1.48 | 0.19 | 0.15 |
Peribronchial cuffing | 21 [42] | 0.79 | 1.23 | 0.52 | 2.21 |
Interstitial edema (e.g., Kerley B-lines) | 22 [44] | 0.66 | 1.57 | 0.67 | 1.94 |
Alveolar edema (e.g., “cotton wool” opacities) | 14 [28] | −0.26 | 1.11 | 0.82 | 0.77 |
Pleural effusion | 27 [54] | −1.75 | 1.32 | 0.19 | 0.17 |
Pneumonic infiltrations | 15 [30] | 1.14 | 0.83 | 0.17 | 3.12 |
Logistic regression analysis was conducted with match (yes/no) as the primary outcome and predictive variables evaluated by radiologists. ORs quantify the effect of each variable on the likelihood of a match. OR, odds ratio.
We explored the repeatability of ChatGPT-4’s assessments on a subgroup of 20 randomly selected chest X-ray scans. ChatGPT-4 was instructed to reevaluate pulmonary congestion using the 5-point Likert scale, replicating the conditions of the initial assessment. Notably, the match rate decreased to 45% (9 out of 20 scans) in the second evaluation, compared to the initial rate of 60% (12 out of 20 scans). Furthermore, ChatGPT-4’s evaluations changed from indicating acute heart failure to not for four chest X-rays, and conversely, for two chest X-rays.
Discussion
This pilot study demonstrates that ChatGPT-4 is not yet prepared to detect pulmonary congestion on chest X-rays, and it should not be used as a fast AI radiology consultant for second opinions by clinical physicians.
One other study has reported on the image analyzing features of ChatGPT-4 and found it to accurately detect pneumonia on chest X-ray (6). However, the study only examined one chest X-ray image in an exploratory manner, where we systematically prompted ChatGPT-4 to evaluate 50 chest X-rays. The low match rate is not likely to improve the outcome significantly even with a larger sample size. Additionally, we observed variability of ChatGPT-4’s evaluations of pulmonary congestion in a repeatability assessment for a subgroup for 20 chest X-rays.
We compared the assessments of emergency chest X-rays from ChatGPT-4 with a golden standard, defined as the independent assessments from two thoracic radiologists. While ChatGPT-4 could not compare with this golden standard, ChatGPT-4 was superior to a random number generator and performed better when clinical information was provided. This suggests that, with continuous updates and improvements, there could be potential for using ChatGPT as an accessible online tool for aiding radiology analysis. However, as the availability of online chest X-ray images for training ChatGPT-4 may be limited, a dedicated AI algorithm, specifically developed for X-ray assessment, could offer superior diagnostic capabilities.
We explored if specific chest X-ray features influenced the matching probability between ChatGPT-4 and the radiologists. Notably, an enlarged heart ratio and signs of pneumonia were suggestive of a higher likelihood of matching, although the small sample size of this pilot study limited statistical significance. On the other hand, features such as pulmonary blood flow redistribution and pleural effusion were often associated with mismatches, suggesting their complexity and potential association with a variety of pulmonary conditions beyond cardiac-induced pulmonary congestion. This underscores the need for further research into how large language models interpret image data, particularly in discerning between different pathologies that share radiological features.
Notably, ChatGPT-4 is not a medically designed technology, which we also experienced as different prompt versions made ChatGPT advise real-world medical consultation rather than giving explicit medical advice (Figures 2,3). For ChatGPT-4 to evaluate chest X-rays, we emphasized a prompt scenario “for research purposes with no medical implications” (Table 1). Moreover, ChatGPT-4 required us to give precise questions with binary or Likert scale responses, otherwise it would often defer to a general radiologist analysis.
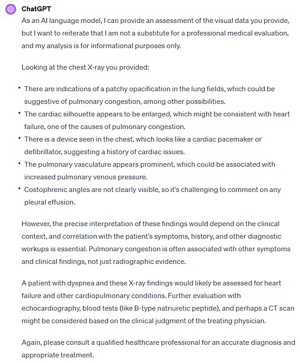
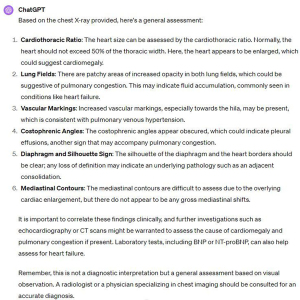
A limitation for this pilot study is that ChatGPT-4 was only provided anterior to posterior X-ray images, whereas the radiologists would have lateral images available for some of the emergency patients being able to stand or sit up straight. We selected 50 chest X-rays based on the radiologists’ assessments to ensure 25 X-rays without pulmonary congestion (Likert scale 1–3) and 25 with congestion (Likert scale 4–5) for further analysis by ChatGPT-4. Consequently, we included chest X-rays with more straightforward diagnostic characteristics and less grey-zone X-rays, still ChatGPT-4’s match rate was merely 54%.
In conclusion, we found that ChatGPT in its current form of version 4 is inadequate for reliably interpreting pulmonary congestion on chest X-rays in an emergency setting. This underscores the necessity for continued advancement and caution in the application of AI technologies like ChatGPT in healthcare contexts.
Acknowledgments
In addition to the X-ray evaluations made by ChatGPT-4 as this study’s main analysis, we used ChatGPT-4 for language revisions of the introduction and method sections. The authors reviewed and edited the content as needed and take full responsibility for the content of the publication.
Funding: This work was supported by
Footnote
Peer Review File: Available at https://jmai.amegroups.com/article/view/10.21037/jmai-24-26/prf
Conflicts of Interest: All authors have completed the ICMJE uniform disclosure form (Available at https://jmai.amegroups.com/article/view/10.21037/jmai-24-26/coif). A.S.O.O. reports salary covered by the Helsefonden grant to the Department of Cardiology, Copenhagen University Hospital – Bispebjerg. K.C.M.’s salary was supported by a research grant from the Danish Cardiovascular Academy funded by the Novo Nordisk Foundation (No. NNF17SA0031406), and the Danish Heart Foundation. O.W.N. is currently employed by the Novo Nordisk Søborg, has received speakers fee from Roche, Orion, Pharmacosmos, and Novartis, and reports stock or stock options from Novo Nordisk, Merck. The other author has no conflicts of interest to declare.
Ethical Statement: The authors are accountable for all aspects of the work in ensuring that questions related to the accuracy or integrity of any part of the work are appropriately investigated and resolved. The study was conducted in accordance with the Declaration of Helsinki (as revised in 2013). The study was approved by the National Ethics Committee Ethics Board of Denmark (No. H-17000869) and informed consent was obtained from all individual participants.
Open Access Statement: This is an Open Access article distributed in accordance with the Creative Commons Attribution-NonCommercial-NoDerivs 4.0 International License (CC BY-NC-ND 4.0), which permits the non-commercial replication and distribution of the article with the strict proviso that no changes or edits are made and the original work is properly cited (including links to both the formal publication through the relevant DOI and the license). See: https://creativecommons.org/licenses/by-nc-nd/4.0/.
References
- Tubaro M, Vranckx P, Bonnefoy E, et al. editors. The ESC textbook of intensive and acute cardiovascular care. Oxford: Oxford University Press; 2021.
- Kwee TC, Kwee RM. Workload of diagnostic radiologists in the foreseeable future based on recent scientific advances: growth expectations and role of artificial intelligence. Insights Imaging 2021;12:88. [Crossref] [PubMed]
- Rajpurkar P, Irvin J, Zhu K, et al. Chexnet: Radiologist-level pneumonia detection on chest x-rays with deep learning. arXiv:1711.05225 [Preprint]. 2017. Available online: http://arxiv.org/abs/1711.05225
.Introducing ChatGPT 2022 . Available online: https://openai.com/blog/chatgpt .How to Use ChatGPT to Interpret X-Rays 2023 . Available online: https://xrayinterpreter.com/resource/how-to-use-chatgpt-to-interpret-xrays- Waisberg E, Ong J, Masalkhi M, et al. GPT-4 and medical image analysis: strengths, weaknesses and future directions. J Med Artif Intell 2023;6:29. [Crossref]
- Srivastav S, Chandrakar R, Gupta S, et al. ChatGPT in Radiology: The Advantages and Limitations of Artificial Intelligence for Medical Imaging Diagnosis. Cureus 2023;15:e41435. [Crossref] [PubMed]
- Olesen ASO, Miger K, Fabricius-Bjerre A, et al. Remote dielectric sensing to detect acute heart failure in patients with dyspnoea: a prospective observational study in the emergency department. Eur Heart J Open 2022;2:oeac073. [Crossref] [PubMed]
- Miger K, Fabricius-Bjerre A, Overgaard Olesen AS, et al. Chest computed tomography features of heart failure: A prospective observational study in patients with acute dyspnea. Cardiol J 2022;29:235-44. [Crossref] [PubMed]
- Hansell DM, Bankier AA, MacMahon H, et al. Fleischner Society: glossary of terms for thoracic imaging. Radiology 2008;246:697-722. [Crossref] [PubMed]
Cite this article as: Overgaard Olesen AS, Miger KC, Nielsen OW, Grand J. How does ChatGPT-4 match radiologists in detecting pulmonary congestion on chest X-ray? J Med Artif Intell 2024;7:18.