Utilizing an apriori algorithm to examine attributes associated with hypertension and hypertension cardiovascular patients in Pakistan
Highlight box
Key findings
• Lifestyle attributes: the analysis revealed that attributes like daily meals, consumption of fruits and vegetables, legumes and meat, and following a high-fat diet were frequently observed among hypertension (HTN) and HTN-cardiovascular disease (CVD) patients.
• Symptom categories: various symptoms, such as neck and jaw pain/discomfort, headaches, and chest pain, were found to be associated with HTN.
• Health status: high systolic blood pressure (SBP) and diastolic blood pressure were commonly observed among the patients.
• Combinations of factors: strong associations were observed between combinations of lifestyle attributes, symptoms, and health status. For example, eating vegetables, high SBP, number of meals per day, chest pain, as well as lifestyle, symptoms, and job exhibited strong associations.
What is known and what is new?
• In our study, we have identified both known and new aspects related to HTN. The known factors include lifestyle attributes such as meals per day, consumption of fruits and vegetables, legumes and meat, and following a high-fat diet, as well as common symptoms like neck and jaw pain, headaches, and chest pain. These factors have been previously associated with HTN in existing research.
• The new aspect of our study is the utilization of the apriori machine learning algorithm to analyze the relationship between various factors and HTN. By applying this algorithm, we were able to uncover patterns and associations that may have been overlooked using traditional statistical methods. This approach adds a novel perspective to understanding the influence of lifestyle, symptoms, and health status on HTN patients.
What is the implication, and what should change now?
• These findings contribute to the understanding of the factors associated with HTN and HTN-CVDs. It highlights the importance of lifestyle modifications and symptom management in preventing and managing these conditions.
Introduction
Cardiovascular disease (CVD) continues to grow and will be the leading cause of death by 2030. At least 45% of deaths are due to heart attacks, and 51% are due to cerebrovascular incidents (1). Hypertension (HTN) is a medical condition with a chronic systemic increase in arterial pressure above the threshold value (140/90 mmHg, as per the European Society of Cardiology and European Society of Hypertension) (1). HTN is a modifiable risk factor in many serious health conditions, including kidney disease, peripheral vascular disease, myocardial infarction, stroke, and congestive heart failure. Uncontrolled HTN can cause kidney failure, stroke and even death (2).
The prevalence of HTN worldwide is estimated to be more than 7 million cases per year, with rates ranging between 20% and 50% of adults have HTN. According to global data, nearly 1 billion people were affected by HTN in 2000, and this number is projected to increase to around 1.56 billion by 2025 (3). Singh et al. conducted a study on HTN in Asian countries and found that the prevalence of HTN in adults living in urban areas ranges from 15% to 35%; notably, the prevalence of HTN in adults living in rural areas was found to be 2–3 times lower compared to urban areas. This highlights the potential impact of lifestyle and environmental factors on HTN rates in different areas (4).
HTN in Pakistan is the most common health disorder suffered by people over 40 years of age. Based on Pakistan’s National Health Survey Report, HTN affects 18% of all adults, rising to 33% for those aged over 45 years. The prevalence of HTN in Pakistan in those aged over 15 years is 18%, with a prevalence in urban residents of 21.6% and rural residents of 16.2%. Around 70% of patients suffering from HTN are unaware of their disease; almost 5.5 million men and 5.3 million women suffer from HTN, but less than 3% of the population is aware of having HTN (5).
Furthermore, HTN in Pakistan is considered a significant risk factor for heart, cerebrovascular, and kidney diseases, and it also plays a crucial role in brain hemorrhage, which can be life-threatening. The increase in the prevalence of HTN can be attributed to various factors, such as population growth and aging, changes in diet, lack of physical activity, increased body mass index (BMI), and excessive alcohol consumption. In urban areas, the prevalence of HTN is exacerbated by urbanization and lifestyle factors, including high alcohol consumption, excessive salt intake, the consumption of fatty foods, and reduced physical activity and sports participation (6).
Individuals who have financial problems or socio-economic problems have a higher risk of developing HTN compared to financially stable individuals. The lack of universal health insurance coverage is an obstacle in accessing health service facilities (7). Low socioeconomic status (SES) is one of the strongest predictors of morbidity and mortality due to HTN (8), and is instrumental in the cardiovascular health gap (8). Socioeconomic factors influence HTN control with respect to diagnosis, treatment, and patient access and adherence to recommended treatment regimens (9). They are also instrumental in diet and lifestyle factors that are instrumental in HTN etiology.
Furthermore, the genetic and environmental factors that make someone more likely to develop HTN are often shared among family members, and it is crucial to consider family history when assessing HTN risk; children of parents with HTN have a higher likelihood of experiencing it. In fact, a family history of HTN that develops before the age of 55 is considered the strongest risk factor for high blood pressure (BP) in offspring. Additionally, even grandparents having HTN can pose a 10% risk to their grandchildren (10). Previous research shows that there is a 30–50% variation in BP that may be inherited (11).
Pharmacological or medication status (MS) treatment of HTN comorbidity in patients with MS involves the same antihypertensive drugs as used in patients without MS. HTN patients require appropriate BP monitoring and timely intervention delivery and medication adherence (12). Some of the symptoms status (SS) of HTN may include headache, pain in the back of the neck, nausea, and sometimes vomiting, dizziness, drowsiness, and irregular heartbeat; long-term high BP can lead to eye damage (13). HTN can cause several subtle symptoms in young and middle-aged women that are often interpreted as “stress” or “menopause-related” (14).
The health status (HS) of HTN sufferers is unstable, which sometimes causes the patient to get sick (47.6%). This is due to the diverse factors instrumental in exacerbation, including poor medication adherence (particularly lack of regularity), high salt consumption, and inadequate physical activity (15). Increased BP is a major risk factor for chronic heart disease, stroke, and coronary heart disease (16), as well as heart failure, peripheral vascular disease, kidney problems, retinal hemorrhages, and vision problems (4).
Literature gap and study rationale
Based on the factors mentioned above that could influence people with HTN to develop CVD, such as health status, symptoms, genetics, socioeconomic, lifestyle, and medication, the authors did not come across any previous studies on HTN that used the apriori algorithm in combination with machine learning. This could be because this specific combination is relatively new and emerging. It is possible that not many researchers have explored it yet. However, this presents an exciting opportunity for the authors to contribute to the field by investigating the potential benefits and applications of incorporating apriori algorithm data mining into machine learning methodologies for this topic.
The aim of the study is to utilize an apriori algorithm to analyze various attributes associated with HTN and HTN-CVD patients in Pakistan. The study focuses on genetic factors, sociodemographic factors, lifestyle factors, medication, symptoms and health-related factors. The goal is to identify and understand the relationships between these factors and the occurrence of HTN and cardiovascular conditions in our dataset of patients from Pakistan.
Methods
Inclusion criteria
Participants comprised male and female CVD patients visiting Military Hospital (MH) and Fauji Foundation Hospital (FFH) Rawalpindi, Pakistan, with symptoms of high BP like headache, dizziness, blurred vision, chest pain, shortness of breath, nausea, and sleep apnea.
Exclusion criteria
Patients were excluded from this study if they had neurological diseases, chronic renal impairment, known psychological illnesses, asthma, pregnancy, alcoholism, advanced hepatic and renal insufficiency, or any other endocrinological disorder.
Ethical permission
The patients were briefed about the purpose and protocol of the study, and their written consent (in English/Urdu) was taken for inclusion in the study. The patients examined at MH Rawalpindi, Pakistan, and FFH, Rawalpindi, Pakistan were selected for the current investigation.
The study was conducted in accordance with the Declaration of Helsinki (as revised in 2013). The study was approved by the institutional board of Pir Mehr Ali Shah Arid Agriculture University Rawalpindi Ethics Committee (No. PMAS-AAUR/1406) for the use of human subjects, and informed consent was obtained from all individual participants.
Data preparation
The BP of hypertensive cardiovascular patients was measured by using a sphygmomanometer. Hypertensive patients were identified based on three measured values of systolic BP higher than 140 mmHg, three measured values of diastolic BP higher than 90 mmHg, or patients taking hypertensive medicines (who were ipso facto diagnosed with HTN).
The height of hypertensive cardiovascular patient subjects was measured using a Harpenden Stadiometer with a precision of 0.1 cm. The weight of males was determined through a digital weight scale as close as 0.1 kg. The determination of BMI was carried out through Cole et al.’s [1997] equation (17):
This retrospective study was conducted to develop a market basket analysis in the context of health data. In this analysis, we apply market basket analysis techniques to examine the patterns and relationships between different antecedents or attribute. Market basket analysis, or association rule mining, is a data mining technique to uncover associations and dependencies among items in a dataset (18).
Data were collected from patients who visited the hospital; participants were randomly selected using their medical records over a 30-day period. Respondents were randomly selected using a random number generator application that was adjusted to the specified inclusion criteria. Patients who met our criteria were invited to voluntarily participate in the survey. All 98 patients in this study were over 19 years old.
To ensure the accuracy of the variables, we took a practical approach to modeling. We systematically added or removed terms and backed them up with statistical calculations using a multinomial regression model and Sharpley additive explanations. This hands-on process involved actively exploring the data to uncover patterns and connections between various attributes associated with HTN and HTN-CVD. Tasks such as data preprocessing, identifying frequent itemsets, and generating association rules were part of the process. This step is displayed in Figure 1.
Market basket analysis
Originally, market basket analysis was one of the leading data mining techniques that focused on understanding customers’ “purchase patterns”. This analysis aims to identify exciting patterns by considering the products customers frequently buy (18). To identify these patterns, the researcher analyses the products that customers purchase together during their visits to the supermarket. A market basket analysis study provides valuable insights into customers’ preferred items, and helps better understand their purchasing behavior. When applied to health data, a market basket model can help identify relationships between health conditions, treatments, medications, or other variables (19-21). Researchers and healthcare professionals can gain insights into potential risk factors, treatment effectiveness, or co-occurrence of certain conditions by analyzing these associations. In our study, we use market basket analysis to understand the relationship between attributes such as lifestyle, economic factors, symptoms, genetic, medications and health status to the output of HTN and HTN-CVD.
We used association rule mining algorithms such as the apriori algorithm to implement a market basket model for our health data. This algorithm helps identify frequent “itemsets/attribute” and generate association rules based on support and confidence measures. In data mining, support measures the relative frequency of an item set in a dataset and confident is the association rule between the antecedent and consequent. For example, if an itemset appears in 12% of the transactions, its support is 12%. It serves as a threshold to identify frequent item sets, which are used to generate association rules. For instance, if we set the support threshold at 5%, any itemset occurring in more than 5% of the transactions would be considered frequent (18).
Analytical tools
The MBA analysis was developed using Python 3.7 (Python Software Foundation, Wilmington, DE, USA). We used the libraries “pandas”, “numpy”, and “scikit-learn”, and the specialized libraries “mlxtend” and “apyori” were employed to facilitate association rule mining and market basket analysis. These tools enabled the examination of relationships and patterns within the market basket data.
Statistical analysis
Demographic data were presented as count (percentages). We noticed that the age feature had two missing data points and two outliers. To handle the missing data, we used the participants’ median values. As for the outliers, we imputed them using the normal data range, with the lower limit calculated as Q1−1.5*IQR and the upper limit as Q3+1.5*IQR. We used Python version 3.7. for data gathering and statistical evaluation.
Results
In this study, we included a total of 98 participants, with 11 HTN and 87 HTN-CVD. The age range of HTN participants was 19–60 years, while for HTN-CVD participants, it was 28–60 years. Among all HTN participants, males accounted for 45%, and females accounted for 55%. For HTN-CVD participants, males made up 64% of the total, while females accounted for 36% (Table 1). Table 2 displays the input sample data for the studied variables. All symptoms are measured by absence or presence, and the diet was considered in terms of daily consumption.
Table 1
Diagnosis | Number of participants [%] | Age participant (years) | Sex | |
---|---|---|---|---|
Male, n [%] | Female, n [%] | |||
HTN | 11 [11] | 19–60 | 5 [45] | 6 [55] |
HTN-CVD | 87 [89] | 28–60 | 56 [64] | 31 [36] |
HTN, hypertension; CVD, cardiovascular disease.
Table 2
Item | Name | Information |
---|---|---|
Attributes variables | ||
1 | Diagnosis | 0 hypertension |
Hypertension is a blood pressure reading of 140/90 mmHg or higher on a medical record for several visits | 1 hypertension cardiovascular | |
Cardiovascular disease by assessing their medical history and examining their medical record | ||
HS | ||
2 | SBP | 0 normal |
Three measures value of SBP | 1 above 140 mmHg | |
3 | DBP | 0 normal |
Three measures value of DBP | 1 above 90 mmHg | |
4 | Health | 0 healthy |
Reviewing medical history, vital signs, symptoms and medical records | 1 non-healthy | |
SS | ||
5 | Headache | 0 no |
Do they often experience headache | 1 yes | |
6 | Dizziness | 0 no |
Do they often experience dizziness | 1 yes | |
7 | Blurred vision | 0 no |
Do they often experience blurred vision | 1 yes | |
8 | Nausea | 0 no |
Do they often experience feeling sickness or discomfort in their stomach | 1 yes | |
9 | Sleep apnea | 0 no |
Do they often experience loud snoring, daytime sleepiness, breathing repeatedly stops and starts during sleep | 1 yes | |
10 | Pain/discomfort (neck, jaw, back) | 0 no |
Do they often experience pain in the neck/jaw/back | 1 yes | |
11 | Feeling weak, lightheaded/faint | 0 no |
Do they often experience faint | 1 yes | |
12 | Chest pain | 0 no |
Do they often experience chest pain | 1 yes | |
13 | Breath | 0 no |
Do they often experience shortness of breath | 1 yes | |
14 | Indigestion | 0 no |
Do they often experience pain in your upper abdomen like bloating, heartburn etc. | 1 yes | |
15 | Palpitations | 0 no |
Do they often experience a pounding heartbeat | 1 yes | |
16 | Chest pain | 0 no |
Do they often experience a pounding heartbeat | 1 yes | |
GS | ||
17 | Family HTN | 0 no |
Do they have any relatives who have hypertension | 1 yes | |
18 | HTN family specify | 0 no direct family (father, mother, sister, brother) |
Please specify if this is a direct family or non-direct family | 1 yes | |
19 | Family CVD | 0 no |
Do they have any relatives who has CVD | 1 yes | |
20 | CVD family specify | 0 no direct family (father, mother, sister and brother) |
Please specify if this is a direct family or non-direct family | 1 yes | |
SES | ||
21 | Job | 0 nonphysical |
Does their job involve physical tasks, or is it more of a non-physical role | 1 manual | |
22 | Income | 0 middle to high income |
It is more than the average income or the lower income brackets, average is 82,100 PKR per month | 1 low income | |
LS | ||
23 | Physical activity | 0 no |
Do they doing physical activity for at least 30 minutes of moderate-intensity exercise | 1 yes | |
24 | Smoking/Naswar | 0 no |
Do they smoke or using Naswar | 1 yes | |
25 | Diet plan | 0 no |
Do they have diet plan | 1 yes | |
26 | Meals/day | 0 no |
Do they eat meals three times daily | 1 yes | |
27 | Fat diet | 0 no |
Do they are having a fat diet | 1 yes | |
28 | Having meat daily | 0 no |
Less than 50 g daily or more than 100 g | 1 yes | |
Less than 50–100 g (one to two servings) per day of unprocessed red meat or recommendation of zero to less than 50 g (one serving) per day of processed red meat to reduce the risk of HTN and CVD (Allen et al., 2022) | ||
29 | Chicken | 0 no |
Do they consume chicken daily | 1 yes | |
30 | Fish | 0 no |
Do they consume fish daily | 1 yes | |
31 | Pulses | 0 no |
Do they consume pulses daily | 1 yes | |
32 | Vegetable | 0 no |
Do they consume vegetables daily | 1 yes | |
33 | Fruits | 0 no |
Do they consume fruit daily | 1 yes | |
MS | ||
34 | Combination medicines and traditional | 0 no |
Do they primarily rely on medications prescribed by doctors or do you prefer traditional remedies | 1 yes | |
35 | Treatment for HTN/HTN-CVD | 0 no |
Do they receive treatment for their hypertension or hypertension cardiovascular treatment | 1 yes |
HTN, hypertension; CVD, cardiovascular disease; HS, health status; SBP, systolic blood pressure; DBP, diastolic blood pressure; SS, symptoms status; GS, genetic status; SES, socioeconomic status; LS, lifestyle status; MS, medication status.
Table 3 is a frequency table displaying attributes, apriori, and types. Each row represents a combination of attributes with their corresponding apriori and type. For instance, in the first row, the attribute {25} or “meals per day” has an apriori of 98%, and in the same row, the attribute {30} or “pulses” also has an apriori of 98%. These attributes are considered highly important, with a 98% apriori.
Table 3
Frequent item set size based on 60% most frequent | Apriority | Frequent set of attributes |
---|---|---|
1 | 0.98–0.73 | {25}, {30}, {31}, {33}, {27}, {2}, {1}, {26}, {10}, {3}, {13}, {11}, {15}, {20}, {12}, {5} … |
2 | 0.98–0.87 | {25, 30}, {31, 25}, {33, 25}, {31, 30}, {31, 33}, {27, 30}, {27, 31}, {27, 33}, {2, 25}, {2, 30} … {30, 1} … |
3 | 0.98–0.87 | {31, 25, 30}, {33, 25, 30}, {31, 33, 35}, {31, 33, 30}, {27, 25, 30}, {27, 25, 31}, {27, 33, 25}, {2, 33, 30}, {31, 2, 25} ... {25, 30, 1} … |
4 | 0.98–0.87 | {31, 33, 25, 30}, {27, 25, 30, 31}, {27, 33, 25, 30}, {27, 33, 30, 31}, {31, 2, 25, 30}, {2, 25, 33, 30}, {31, 2, 25, 33}, {31, 2, 33, 30} … {31, 25, 30, 1} … |
5 | 0.96–0.87 | {27, 25, 31, 30, 33}, {2, 25, 31, 30, 33}, {27, 2, 25, 31, 30}, {27, 2, 31, 30, 33}, {27, 2, 31, 30, 33}, {25, 31, 30, 33, 1}, {27, 25, 31, 30, 1}, {27, 25, 30, 33, 1} … |
6 | 0.88–0.74 | {27, 2, 25, 31, 30, 33}, {27, 25, 31, 30, 33, 1}, {2, 25, 31, 30, 33, 1}, {27, 2, 25, 30, 33, 1}, {27, 2, 25, 31, 33, 1}, {27, 2, 31, 30, 33, 26}, {2, 25, 31, 30, 33, 3} … |
7 | 0.78–0.70 | {27, 2, 25, 31, 30, 33, 1}, {27, 2, 25, 31, 30, 33, 3}, {27, 2, 25, 31, 30, 33, 26}, {27, 25, 31, 30, 33, 1, 26}, {27, 2, 25, 31, 20, 30, 33}, {13, 27, 25, 31, 30, 33, 15} … |
8 | 0.60–0.61 | {2, 25, 31, 30, 33, 3, 1, 26}, {27, 2, 25, 31, 30, 33, 3, 1}, {27, 2, 25, 10, 31, 30, 33, 1}, {27, 2, 25, 12, 31, 30, 33, 1}, {13, 27, 2, 25, 31, 30, 15, 1}, {13, 27, 2, 25, 30, 33, 15, 1} |
9 | 0.61 | {13, 27, 2, 25, 31, 30, 33, 15, 1} |
There are specific attributes that hold high importance in the dataset, as indicated by their 98% apriori. For example, attributes from the lifestyle group, such as {25} or “meals per day”, {30} or “eating pulses”, {31} or “eating vegetables”, {27} or “eating meat”, {26} or “fat diet”, and are considered significant, and play a crucial role in the dataset. Similarly, attributes from the symptoms group, like {33} or “chest pain”, have a high apriori of 98%, while {11} or “feeling weak/lightheaded/faint”, {15} or “palpitations”, {10} or “pain/discomfort (neck, jaw, back)” and {5} or “headaches” have a lower apriori range, from 74–77%. When it comes to health factors, {2} or “systolic blood pressure (SBP)” has a higher apriori of 91% compared to {3} or “diastolic blood pressure (DBP)”, which has apriori of 77%, lastly from socioeconomic group attribute {20} or “job” also have high apriori 78%.
When looking at the attributes that appear together in a diagnosis, the second row of the table shows two attributes that have a 98% apriori. For example, the attribute combination {25, 30} represents “eating vegetable and meals per day”, while {31, 33} represents “eating vegetable and chest pain”. The table also highlights the three attributes with the highest apriori, namely {31, 25, 30} which represents “eating vegetable, meals per day, and eating pulses”. Table 3 displays more comprehensive information.
Figure 2 displays the frequent attributes that appear in both HTN and HTN-CVD patients, it presents a scatter plot showing the relationship between the attribute and its frequent occurrence or apriori score, which is also explained in Table 3. In Table 4, each row represents a specific attribute combination and its associated information, including the antecedent and consequent support. The antecedent support refers to the proportion of instances wherein the antecedent attributes (i.e., those on the left-hand side) are present in the dataset. In this case, it indicates the frequency of occurrences of attributes like eating pulses, blurred vision, chest pain, eating vegetables, meals/day, etc. The consequent support represents the proportion of instances where the consequent attribute (the attribute on the right-hand side) is present in the dataset. In this case, it indicates the frequency of occurrences of attributes like diagnosis, blurred vision, chest pain, meals/day, etc.
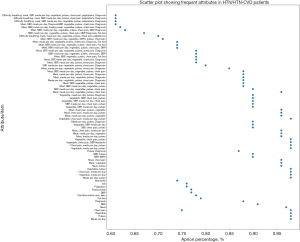
Table 4
Frequency attribute | Apriority | Types |
---|---|---|
1 | 0.98 | Meals per day |
0.98 | Pulses | |
0.98 | Vegetable | |
0.98 | Chest pain | |
0.97 | Meat | |
0.91 | SBP | |
0.88 | Diagnosis | |
0.79 | Fat diet | |
0.78 | Pain/discomfort neck, jaws | |
0.77 | DBP | |
0.77 | Feeling weak | |
0.76 | Palpitation | |
0.76 | Job | |
0.74 | Headache | |
2 | 0.98 | Meals per day, pulses |
0.98 | Vegetable, meals per day | |
0.98 | Chest pain, meals per day | |
0.98 | Vegetable, pulses | |
0.96 | Meat, pulses | |
0.96 | Meat, vegetable | |
0.96 | Meat, chest pain | |
0.9 | SBP, meals per day | |
0.9 | SBP, pulses | |
0.87 | Pulses, diagnosis | |
3 | 0.98 | Vegetable, meals per day, pulses |
0.98 | Chest pain, meals per day, pulses | |
0.98 | Vegetable, chest pain, treatment | |
0.98 | Vegetable, chest pain, pulses | |
0.96 | Meat, meals per day, pulses | |
0.96 | Meat, meals per day, vegetable | |
0.96 | Meat, chest pain, meals per day | |
0.9 | SBP, chest pain, pulses | |
0.9 | Vegetable, SBP, meals per day | |
0.9 | Meals per day, pulses, diagnosis | |
4 | 0.98 | Vegetable, chest pain, meals per day, pulses |
0.96 | Meat, meals per day, pulses, vegetable | |
0.96 | Meat, chest pain, meals per day, pulses | |
0.96 | Meat, chest pain, pulses, vegetable | |
0.9 | Vegetable, SBP, meals per day, pulses | |
0.9 | SBP, meals per day, chest pain, pulses | |
0.9 | Vegetable, SBP, meals per day, chest pain | |
0.9 | Vegetable, SBP, chest pain, pulses | |
0.9 | Vegetable, meals per day, pulses, diagnosis | |
5 | 0.96 | Meat, meals per day, vegetable, pulses, chest pain |
0.9 | SBP, meals per day, vegetable, pulses, chest pain | |
0.88 | Meat, SBP, meals per day, vegetable, pulses | |
0.88 | Meat, SBP, vegetable, pulses, chest pain | |
0.88 | Meals per day, vegetable, pulses, chest pain, diagnosis | |
0.88 | Meat, meals per day, vegetable, pulses, diagnosis | |
0.88 | Meat, meals per day, pulses, chest pain, diagnosis | |
6 | 0.88 | Meat, SBP, meals per day, vegetable, pulses, chest pain |
0.85 | Meat, meals per day, vegetable, pulses, chest pain, diagnosis | |
0.8 | SBP, meals per day, vegetable, pulses, chest pain, diagnosis | |
0.78 | Meat, SBP, meals per day, pulses, chest pain, diagnosis | |
0.78 | Meat, SBP, meals per day, vegetable, chest pain, diagnosis | |
0.78 | Meat, SBP, vegetable, pulses, chest pain, fat diet | |
0.78 | SBP, meals per day, vegetable, pulses, chest pain, DBP | |
7 | 0.78 | Meat, SBP, meals per day, vegetable, pulses, chest pain, diagnosis |
0.74 | Meat, SBP, meals per day, vegetable, pulses, chest pain, DBP | |
0.74 | Meat, SBP, meals per day, vegetable, pulses, chest pain, fat diet | |
0.74 | Meat, meals per day, vegetable, pulses, chest pain, diagnosis, fat diet | |
0.71 | Meat, SBP, meals per day, vegetable, pulses, chest pain | |
0.7 | Difficulty breathing, meat, meals per day, vegetable, pulses, chest pain, palpitations | |
8 | 0.67 | SBP, meals per day, vegetable, pulses, chest pain, DBP, diagnosis, fat diet |
0.63 | Meat, SBP, meals per day, vegetable, pulses, chest pain, DBP, diagnosis | |
0.62 | Meat, SBP, meals per day, feeling weak, vegetable, pulses, chest pain, diagnosis | |
0.61 | Meat, SBP, meals per day, diagnosis, SBP, vegetable, pulses, chest pain, diagnosis | |
0.61 | Difficulty breathing, meat, SBP, meals per day, vegetable, pulses, palpitations, diagnosis | |
0.61 | Difficulty breathing, meat, SBP, meals per day, pulses, chest pain, palpitations, diagnosis | |
9 | 0.61 | Difficulty breathing, meat, SBP, meals per day, vegetable, pulses, chest pain, palpitations, diagnosis |
SBP, systolic blood pressure; DBP, diastolic blood pressure.
In our study, the itemset {30, 7, 33} pulses, blurred vision, chest pain has antecedent support 0.88, consequent support 0.73 and confidence of 0.75, meaning that there is a strong association between the antecedent (pulses, blurred vision, chest pain) and the consequent. The high support values indicate that these symptoms are frequently observed together, while the confidence of the association rule between the antecedent and consequent is 0.75, indicating that there is a 75% likelihood that the consequent will occur when the antecedent is present.
The associations between different attributes and diagnoses shown in Table 5 demonstrate that attributes such as {30, 7, 33} or “eating pulses”, “symptom blurred vision”, and “chest pain” have an apriori of 88% to the diagnosis. Additionally, the table reveals that the attributes {31, 7, 33, 1} or “eating vegetables”, “symptoms of blurred vision” and “chest pain”, and “diagnosis” are interrelated by 88%. Moreover, the table highlights the relationship between attributes {31, 7, 33, 30, 25} or “eating vegetable”, “blurred vision”, “chest pain”, “meals per day” accounted for 88%. Lastly, the table showcases the connection between diagnosis and symptom of blurred vision, diagnosis and symptoms of chest pain, and lifestyle “eating meat”, as well as “diagnosis” and lifestyle “eating vegetable”, “meals/days”, and symptoms of “blurred vision”.
Table 5
Attribute diagnosis | Attribute | Information | Antecedent support | Consequent support | Confidence |
---|---|---|---|---|---|
1 | {30, 7, 33} | Pulses, blurred vision, chest pain | 0.88 | 0.73 | 0.75 |
{31, 7, 33} | Vegetable, blurred vision, chest pain | 0.88 | 0.73 | 0.75 | |
{31, 30 7} | Vegetable, pulses, blurred vision | 0.88 | 0.73 | 0.97 | |
{31, 7, 33, 30, 25} | Vegetable, blurred vision, chest pain, meals/day | 0.88 | 0.73 | 0.75 | |
{7, 25} | Blurred vision, meals/day | 0.88 | 0.73 | 0.75 | |
{7} | Blurred vision | 0.88 | 0.73 | 0.75 | |
{7, 33, 25} | Blurred vision, chest pain, meals/day | 0.88 | 0.73 | 0.97 | |
{12, 27} | Chest pain, having meat | 0.88 | 0.73 | 0.97 | |
{31, 7, 25} | Vegetable, blurred vision, meals/day | 0.88 | 0.73 | 0.97 |
The table’s values of antecedent support and consequent support are both 0.88, indicating a high frequency of occurrence for these attribute combinations. Overall, Table 5 provides insights into the relationships between different attributes and their association with the diagnosis, as well as the frequency of occurrence of these attribute combinations in the dataset.
Discussion
After applying the apriori algorithm to the dataset we collected, we discovered frequent sets of attributes in connection with HTN and HTN-CVD patients. These sets include 1-item, 2-item, 3-item, and so on, which are generated from the candidate item sets. These frequent attribute item sets are helpful in implementing preventive measures to address common attributes in the dataset of lifestyle status (LS), HS, SS, SES, genetic status (GS), and MS. Table 2 displays the sample input dataset, for this experiment, we considered a minimum support count of 60%.
If we break down the frequent item sets from the results. In the 1-item set, we have LS, SS, HS, which occur often (refer to the first row of Table 3). Moving on to the 2-item set, we have {25, 30}, where 25 represents “meals per day”, and 30 represents “eating pulses daily”. These attributes fall under the common attribute group of lifestyles. Similarly, in the 3-item set {31, 25, 30}, we have “eating vegetables”, “meals per day”, and “eating pulses daily”, all falling under the common group of lifestyles. We also have another 3-item set {33, 25, 30}, where 33 represents “chest pain”, and 25 and 30 refer to the attributes mentioned earlier. This set combines symptoms and lifestyle attributes. Moving on to the 4-item set {31, 33, 25, 30}, we have “eating vegetables”, “chest pain”, “meals per day”, and “eating pulses”, all representing symptoms and lifestyle attributes. As we move to the 6-item set {27, 2, 25, 31, 30, 33}, we introduce other attributes like “SBP” from the health status group, and {27, 2, 25, 31, 20, 30, 33} we introduce another attribute SES “job”. These frequent item sets with a minimum support count of 60% or above share common attributes related to lifestyle, symptoms, general health conditions and SES. In our study, we found that certain factors appeared most frequently in the data set for HTN and HTN-CVD, including lifestyle attributes like regularly eating vegetables, having three meals a day, and consuming a moderate amount of meat. It is also important to note that regularly consuming a high-fat diet and pulses were common attributes.
The current research has identified other attributes that frequently occur together, as shown in Table 4. This information can help health professionals and stakeholders plan the necessary approach to ensure the health of patients with HTN and HTN-related CVDs. Health workers can raise awareness among the general public about the attributes that commonly appear in HTN patients, enabling them to assist their patients in preventing further complications.
Similarly, in the symptoms group, we found that patients who regularly experienced chest pain, feelings of weakness/lightheadedness/faintness, palpitations, and pain/discomfort in the neck, jaw, or back, as well as headaches, difficulty breathing were quite common.
When it comes to health factors, having higher systolic BP appeared in 91% of the data, and higher diastolic BP was also a common attribute. According to the 2019 American College of Cardiology/American Heart Association (ACC/AHA) Guideline on the Primary Prevention of Cardiovascular Disease, individuals with high BP are advised to start with lifestyle changes as the first step of treatment. These changes include a healthy diet for heart health (22).
LS are an important factor in increasing BP. The combination of an exercise program and weight loss can have an effect on BP, and the intensity of physical activity also affects BP (23-25). Available evidence suggests a direct relationship between sodium intake and BP; increased salt consumption can trigger water retention, causing elevated volumes of blood flow in the vessels (26). The study showed that lifestyle factors influence essential HTN. These factors encompass obesity, excessive fat consumption, inadequate intake of fruits, vegetables, whole-grain foods, and pulses (27-29).
The relationship between SS and HTN showed significant results. A number of cardiopulmonary and neurological symptoms are thought to be associated with HTN. Al Anas and Nuralita showed that there is a relationship between anxiety symptoms and the incidence of HTN, expressed by a P value of 0.001 (P<0.05) (30). Zhang and Zhang examined the relationship between psychological impacts and the incidence of HTN and showed that there was a relationship between anxiety symptoms and HTN: the higher the psychological symptoms, the higher the incidence of HTN, HTN can cause fatigue, headaches, a heavy feeling in the neck, dizziness, blurred vision, palpitations, ringing in the ears, and nosebleeds (31). Patients with HTN who have poor lifestyle habits and coping skills are more likely to experience emotional disturbances, leading to feelings of tension, anxious thoughts, and physical changes such as increased BP (32,33).
HS is also related to HTN. HTN experienced significant decreases in measures of general Health status associated with increases in the number of antihypertensives, HTN and its treatment with medication have been found to significantly impact reported health status. HTN is defined as having a SBP (≥140/90 or ≥130/80 mmHg); such elevated SBP has significant impacts, and both systolic and diastolic HTN independently influence the risk of adverse cardiovascular events, regardless of the HTN definition (34,35). HTN and HTN-CVD are inversely correlated with general health, and can lead to physical problems and social dysfunction, depression, anxiety, and insomnia (36). Thus, effective management of HTN is an absolute requirement to improve the health status of HTN and HTN-CVD patients. HTN can be treated with medication therapy and LS.
The study found a strong connection between symptoms like blurred vision, chest pain, and lifestyle factors such as consuming pulses and vegetables, with a diagnosis of HTN or HTN-related cardiovascular issues. The high antecedent support value indicates that the symptoms are frequently observed in patients with this diagnosis.
In terms of implementation, these results can help disease management and complications: inform medical professionals about potential indicators of HTN or cardiovascular issues. By recognizing the presence of these symptoms, healthcare providers can consider conducting further tests or evaluations to confirm diagnosis and provide appropriate treatment, this result will be helpful to advocate healthy lifestyle and management of complications.
Advocating a healthy lifestyle is the best policy for this in Pakistan. It would be beneficial to focus on promoting healthy lifestyle habits, such as encouraging individuals to consume a balanced diet with fruits, vegetables, and legumes and to follow a low-fat diet.
Additionally, disease diagnosis by raising awareness about the symptoms of HTN and the importance of regular BP monitoring can help in early detection and timely intervention. Implementing strategies to improve access to healthcare services and medication for patients with HTN can also be beneficial.
Conclusions
Based on the market basket data analysis undertaken in this study, it is clear that LF, SS, HS and SES are frequently associated among HTN and HTN-CVD patients. Lifestyle attributes like meals per day, eating fruits and vegetables, eating legumes and meat, and following eating a high-fat diet are the most commonly observed items in the analysis. Other associated attributes fall under the categories of symptoms such as pain/discomfort in the neck and jaws, headache, and chest pain. In terms of health status, high BP (SBP and DBP) are also common. Combinations of lifestyle attributes, symptoms, and health status, such as eating vegetables, high BP (SBP), meals per day, and chest pain, and also lifestyle, symptoms and job show a very high apriori. These findings enhance our understanding of the relationship between risk groups and the presence of HTN in patients, providing valuable insights into patterns and associations among lifestyle, symptoms, medication, and health status.
Limitations
It was impossible in this study to encompass the vast expanse of tabulated results, to discuss each association separately. Instead, we summarized a few of the most significant findings, to emphasize the overall patterns and trends observed in the market basket analysis. We also explained how to interpret the tables, to encourage readers and researchers to explore the data and its implications further.
Acknowledgments
Funding: None.
Footnote
Data Sharing Statement: Available at https://jmai.amegroups.com/article/view/10.21037/jmai-24-15/dss
Peer Review File: Available at https://jmai.amegroups.com/article/view/10.21037/jmai-24-15/prf
Conflicts of Interest: All authors have completed the ICMJE uniform disclosure form (available at https://jmai.amegroups.com/article/view/10.21037/jmai-24-15/coif). The authors have no conflicts of interest to declare.
Ethical Statement: The authors are accountable for all aspects of the work and ensure that questions related to the accuracy or integrity of any part of the work are appropriately investigated and resolved. The study was conducted in accordance with the Declaration of Helsinki (as revised in 2013). The study was approved by the institutional board of Pir Mehr Ali Shah Arid Agriculture University Rawalpindi Ethics Committee (No. PMAS-AAUR/1406) for the use of human subjects, and informed consent was obtained from all individual participants.
Open Access Statement: This is an Open Access article distributed in accordance with the Creative Commons Attribution-NonCommercial-NoDerivs 4.0 International License (CC BY-NC-ND 4.0), which permits the non-commercial replication and distribution of the article with the strict proviso that no changes or edits are made and the original work is properly cited (including links to both the formal publication through the relevant DOI and the license). See: https://creativecommons.org/licenses/by-nc-nd/4.0/.
References
- Elahi A, Ali AA, Khan AH, et al. Challenges of managing hypertension in Pakistan - a review. Clin Hypertens 2023;29:17. [Crossref] [PubMed]
- Riaz M, Shah G, Asif M, et al. Factors associated with hypertension in Pakistan: A systematic review and meta-analysis. PLoS One 2021;16:e0246085. [Crossref] [PubMed]
- Shafi ST, Shafi T. A survey of hypertension prevalence, awareness, treatment, and control in health screening camps of rural central Punjab, Pakistan. J Epidemiol Glob Health 2017;7:135-40. [Crossref] [PubMed]
- Singh S, Shankar R, Singh GP. Prevalence and Associated Risk Factors of Hypertension: A Cross-Sectional Study in Urban Varanasi. Int J Hypertens 2017;2017:5491838. [Crossref] [PubMed]
- Fuchs FD, Whelton PK. High Blood Pressure and Cardiovascular Disease. Hypertension 2020;75:285-92. [Crossref] [PubMed]
- Modey Amoah E, Esinam Okai D, Manu A, et al. The Role of Lifestyle Factors in Controlling Blood Pressure among Hypertensive Patients in Two Health Facilities in Urban Ghana: A Cross-Sectional Study. Int J Hypertens 2020;2020:9379128. [Crossref] [PubMed]
- Nakagomi A, Yasufuku Y, Ueno T, et al. Social determinants of hypertension in high-income countries: A narrative literature review and future directions. Hypertens Res 2022;45:1575-81. [Crossref] [PubMed]
- Lam CS. The socioeconomics of hypertension: how $50 000 may buy a drop in blood pressure. Hypertension 2011;58:140-1. [Crossref] [PubMed]
- Madu E, Madu K, Jacobowitz W. The Relationship between Socioeconomic Status and Adherence to Antihypertensive Treatment Regimen in a Metropolitan Community Sample of Hypertensive African Americans in New York. Public Health Open J 2019;4:44-51. [Crossref]
- Louca P, Menni C, Padmanabhan S. Genomic Determinants of Hypertension With a Focus on Metabolomics and the Gut Microbiome. Am J Hypertens 2020;33:473-81. [Crossref] [PubMed]
- Patel RS, Masi S, Taddei S. Understanding the role of genetics in hypertension. Eur Heart J 2017;38:2309-12. [Crossref] [PubMed]
- Mark M, Duff E. Primary Care Management of Hypertension in Patients With Multiple Sclerosis. The Journal for Nurse Practitioners 2023;19:104652. [Crossref]
- Oparil S, Acelajado MC, Bakris GL, et al. Hypertension. Nat Rev Dis Primers 2018;4:18014. [Crossref] [PubMed]
- Maas AHEM. Hypertension in women: no “silent” lady-killer. E-Journal of Cardiology Practice 2019;17(21).
- Achjar KAH, Kusumawardani LH, Parashita SAP. Health Status of Older Adults with Hypertension after Family and Cadre Empowerment through Comprehensive Care. Media Karya Kesehatan 2022;5:79-94. [Crossref]
- Sabapathy K, Mwita FC, Dauya E, et al. Prevalence of hypertension and high-normal blood pressure among young adults in Zimbabwe: findings from a large, cross-sectional population-based survey. Lancet Child Adolesc Health 2024;8:101-11. [Crossref] [PubMed]
- Cole TJ, Flegal KM, Nicholls D, et al. Body mass index cut offs to define thinness in children and adolescents: international survey. BMJ 2007;335:194. [Crossref] [PubMed]
- Loshin D. Chapter 17 - Knowledge Discovery and Data Mining for Predictive Analytics. In: Loshin D. editor. Business Intelligence. 2nd edition. Morgan Kaufmann; 2013:271-86.
- Jayawardene WP. YoussefAgha AH. Multiple and substitute addictions involving prescription drugs misuse among 12th graders: gateway theory revisited with Market Basket Analysis. J Addict Med 2014;8:102-10. [Crossref] [PubMed]
- Ali Y, Farooq A, Alam TM, et al. Detection of Schistosomiasis Factors Using Association Rule Mining. IEEE Access 2019;7:186108-14.
- Rao AB, Kiran JS, Poornalatha G. Application of market–basket analysis on healthcare. International Journal of System Assurance Engineering and Management 2021;14:924-9.
- Khalil H, Zeltser R. Antihypertensive Medications. Treasure Island, FL, USA: StatPearls Publishing; 2023.
- Beilin LJ, Puddey IB, Burke V. Lifestyle and hypertension. Am J Hypertens 1999;12:934-45. [Crossref] [PubMed]
- Diaz KM, Shimbo D. Physical activity and the prevention of hypertension. Curr Hypertens Rep 2013;15:659-68. [Crossref] [PubMed]
- Mills KT, Stefanescu A, He J. The global epidemiology of hypertension. Nat Rev Nephrol 2020;16:223-37. [Crossref] [PubMed]
- Grillo A, Salvi L, Coruzzi P, et al. Sodium Intake and Hypertension. Nutrients 2019;11:1970. [Crossref] [PubMed]
- Samadian F, Dalili N, Jamalian A. Lifestyle Modifications to Prevent and Control Hypertension. Iran J Kidney Dis 2016;10:237-63. [PubMed]
- Jayalath VH, de Souza RJ, Sievenpiper JL, et al. Effect of dietary pulses on blood pressure: a systematic review and meta-analysis of controlled feeding trials. Am J Hypertens 2014;27:56-64. [Crossref] [PubMed]
- Li B, Li F, Wang L, et al. Fruit and Vegetables Consumption and Risk of Hypertension: A Meta-Analysis. J Clin Hypertens (Greenwich) 2016;18:468-76. [Crossref] [PubMed]
- Al Anas M, Nuralita NS. Association Between Anxiety Symptoms and Degree of Hypertension in Rural Indonesia. International Journal of Innovations in Engineering Research and Technology 2021;8:34-8.
- Zhang Y, Zhang DZ. Red meat, poultry, and egg consumption with the risk of hypertension: a meta-analysis of prospective cohort studies. J Hum Hypertens 2018;32:507-17. [Crossref] [PubMed]
- Radojicic A, Vukovic-Cvetkovic V, Pekmezovic T, et al. Predictive role of presenting symptoms and clinical findings in idiopathic intracranial hypertension. J Neurol Sci 2019;399:89-93. [Crossref] [PubMed]
- Stacey AW, Sozener CB, Besirli CG. Hypertensive emergency presenting as blurry vision in a patient with hypertensive chorioretinopathy. Int J Emerg Med 2015;8:13. [Crossref] [PubMed]
- Lawrence WF, Fryback DG, Martin PA, et al. Health status and hypertension: a population-based study. J Clin Epidemiol 1996;49:1239-45. [Crossref] [PubMed]
- Flint AC, Conell C, Ren X, et al. Effect of Systolic and Diastolic Blood Pressure on Cardiovascular Outcomes. N Engl J Med 2019;381:243-51. [Crossref] [PubMed]
- Moodi M, Sharifzadeh G, Saadatjoo SS. General Health Status and its Relationship With Health-Promoting Lifestyle Among Patients With Hypertension. Modern Care Journal 2015;12:e8674. [Crossref]
Cite this article as: Nuryunarsih D, Wahyuningsih HP, Rauf S, Zaidan M, Herawati L. Utilizing an apriori algorithm to examine attributes associated with hypertension and hypertension cardiovascular patients in Pakistan. J Med Artif Intell 2024;7:34.