Assessment of accuracy of an early artificial intelligence large language model at summarizing medical literature: ChatGPT 3.5 vs. ChatGPT 4.0
Highlight box
Key findings
• ChatGPT 4.0 significantly outperforms its predecessor, ChatGPT 3.5, in summarizing medical literature, with fewer errors and displays better comprehension.
What is known and what is new?
• It is known that ChatGPT 4.0 has produced fewer hallucinations than ChatGPT 3.5. The degree of accuracy, specifically within the domain of medical decision support, has been incompletely quantified. This study quantifies that accuracy.
What is the implication, and what should change now?
• The study explores the accuracy of a popular, advanced large language model at medical knowledge summarization. This paper highlights the shortcomings of that system in that domain. Benchmarking medical decision support and summarization of medical literature should continue to be studied and should be standardized.
Introduction
One of the applications of artificial intelligence (AI) proposes performing tasks by computers that humans have traditionally performed. Machine learning is an advanced version of AI in which the performance of such tasks is improved without explicit programming but through training on relevant data (1). Neural networks are machine learning models involving neuron layers loosely based on the structure of neuronal connections seen in physiology (2). Deep learning models are a type of neural network that involves multiple, often numerous, layers of digital neurons. Deep learning has succeeded at solving problems in computing that were once unreachable, and these systems now often outperform humans in various fields, such as facial recognition and analytic strategy games (3). Transformers are a type of deep learning architecture that simultaneously maintains the meaning and context of a string of letters. Generative pre-trained transformers (GPTs) are an application of a transformer model that is trained on a large corpus of text with the purpose of generating novel human-like content (4).
ChatGPT is a conversational version of a GPT produced by the company OpenAI that, in its most recent iterations, has produced impressive conversational performance that can often compete with that of humans. Specifically, ChatGPT now rivals medical students in USMLE scores and various specialized board examinations (5). It has also outperformed physicians in providing high-quality, empathetic responses to written questions on online medical forums, with a panel of licensed healthcare professionals preferring ChatGPT responses 79% of the time (6). Notably, however, in its current version, ChatGPT can produce factually incorrect responses and may invent or alter existing sources of information termed in large language model (LLM) research as “artificial hallucinations” (7). This tendency has decreased in newer versions of ChatGPT. However, its persistence offers a challenge to users who may consider it a source of credible information (8-10).
Current investigations into the usefulness of ChatGPT for clinical decision support and medical literature summarization include the perspective piece by Jin et al. that concluded that both ChatGPT 3.5 and 4.0 produced plausible sounding medical responses. However, ChatGPT 3.5 provided fabricated citations whereas ChatGPT 4.0 refused to cite articles altogether. They also showed that summaries generated by these LLMs included inaccuracies, missing citations, and excluded contradictory evidence relevant to the prompts (11). Specialized LLMs for summarizing medical literature have been produced and benchmarked. Lozano et al. described production and testing of Clinfo.ai, which is an open-source Retrieval-Augmented Large Language Model system for answering medical questions using Scientific Literature. They built a benchmark question set titled PubMedRS-200 by which to evaluate the effectiveness of that model. Their model outperformed ChatGPT 3.5 and 4.0 on a number of automated metrics on PubMEDRS-200, expected to have moderate to high correlation with human preferences (12). Fleming et al. described a clinician-generated question answering dataset for benchmarking LLMs at tasks involving the electronic health record (EHR), named MedAlign. They tested that dataset on six general domain LLMs using physician evaluation, noting a significant rate of errors from 35% (ChatGPT 4.0) to 68% (MPT-7B-Instruct). They also evaluated the correlation between clinician ranking and automated natural language generation metrics with the aim to rank LLMs without human review (13). In a study by Tang et al., researchers evaluated medical evidence summarization by ChatGPT 3.5 and ChatGPT via both automated and human evaluators with domain specific expertise. Consistent with other literature, they found that LLMs were susceptible to factual errors and were more error-prone when summarizing over longer textual contexts (14). Our paper addresses a human based evaluation of ChatGPT 3.5 and 4.0 medical literature summarization using multiple physician evaluators in a simple grading scheme that is easily reproducible with a focus on clinical applicability of summarization conclusions.
Methods
ChatGPT versions 3.5 and 4.0 summarized the top 25 most cited publications using the keyword “trauma” from the New England Journal of Medicine. Two physicians trained in trauma and critical care surgery assessed these summaries to accurately establish the paper’s purpose, methodology, conclusions, and primary clinical conclusion. Quantitative and qualitative assessment of errors was performed. The summaries were produced by asking ChatGPT 3.5 and 4.0 via the web chat platform with the same prompt, only changing the name of the paper to be evaluated. The following prompt language was used on every summary: Please summarize “NAME OF PAPER”. Each summarization was produced in a separate window. Summaries were produced over a <72-hour period to ensure that updates to the GPT model would be unlikely to affect the summary. Specifically, ChatGPT 3.5 summaries were produced using the May 3rd version accessed on May 11, 2023, between 8 am and 3 pm Mountain Standard Time (MST). ChatGPT 4.0 summaries were produced by accessing ChatGPT 4.0 starting October 22, 2023, at 8 am until October 24, 2023, at 5 pm. Over that period of time, no significant updates to the models were noted on the OpenAI website for each GPT version. Both versions of ChatGPT were utilized without plugins for access to query the internet. Therefore, their summaries reflected only their understanding of the literature during their training. Physician evaluators then reviewed these summaries on the following criteria: number of errors, types of errors, whether the summary explained why the paper was written, how the study was performed, the conclusions of the study, and whether the primary clinical conclusion of the paper was accurately reflected in the summary. Types of errors were categorized as ‘numeric inaccuracy’, ‘misrepresents study design’, and ‘misrepresents study conclusion’. Specifically, numeric inaccuracy was defined as an error in which the summary misrepresented a number, such as the sample size, that was in the original paper. A ‘misrepresentation of study design’ error was one that inaccurately reflected the study design, for example, defining the study as a prospective study when it was a retrospective or misrepresenting the study medications or primary outcomes to be measured. A ‘misrepresentation of study conclusion’ was defined as inaccurately representing the study findings, such as rejecting the study’s hypothesis when, in fact, the hypothesis was accepted or drawing an additional conclusion not addressed in the paper.
Results
Error analysis was performed by evaluating the total number of errors and the types of errors made. We also analyzed the study comprehension of each summary and a descriptive analysis of the content produced by both ChatGPT 3.5 and 4.0 models. The relevant exclusions to the study are detailed below.
Exclusion
Of the 25 articles that returned through our search of “Trauma” in the New England Journal of Medicine, two articles were deemed not of a clinical nature, and were therefore excluded from analysis (articles 1 and 4). One article contained a prompt error on secondary review of the summary, and therefore its analysis was excluded (article 6). For four of the articles, ChatGPT 4.0 claimed no knowledge of the paper and refused to summarize, and therefore we excluded these articles from the analysis (articles 18, 20, 22, and 23). A full display of the article titles and exclusions may be found in Table S1. The complete results of each reviewer’s analysis of ChatGPT 3.5 and 4.0 may be found in Tables S2,S3.
Error analysis
Total number of errors
Reviewer 1 noted 27 errors across 18 articles for ChatGPT 3.5 and 13 errors across 18 articles for ChatGPT 4.0. This equates to an error rate of 1.5 and 0.72 per article for ChatGPT 3.5 and 4.0, respectively. Reviewer 2 noted 22 errors across 18 articles for ChatGPT 3.5 and 2 errors across 18 articles for ChatGPT 4.0. This equates to an error rate of 1.2 and 0.11 for ChatGPT 3.5 and 4.0, respectively. Both reviewers noted an improvement in rate of error for ChatGPT 4.0 as compared with ChatGPT 3.5.
Types of errors made
Reviewer 1 found that the most common error that ChatGPT 3.5 produced was a misrepresentation of study design (noted for 9 of 18 articles), whereas the most common error that ChatGPT 4.0 produced was a misrepresentation of study conclusions (noted for 7 of 18 articles). Reviewer 1 found a significant improvement from ChatGPT 3.5 to 4.0 summaries on all categories of errors, notably finding that ChatGPT 3.5 only produced 3 of 18 summaries completely devoid of error as compared with 7 of 18 for ChatGPT 4.0. The results of reviewer 1’s tally of errors are displayed in Figure 1 and Table S4.

Reviewer 2 found that the most common error that ChatGPT 3.5 produced was a numeric inaccuracy (noted for 10 of 18 articles), whereas the most common error that ChatGPT 4.0 produced was also numeric inaccuracy (noted for 1 of 18 articles). Reviewer 2 found a significant improvement from ChatGPT 3.5 to 4.0 summaries on most categories of errors, notably finding that ChatGPT 3.5 only produced 8 of 18 summaries completely devoid of error as compared with 17 of 18 for ChatGPT 4.0. The results of reviewer 2’s tally of errors are displayed in Figure 2 and Table S5.
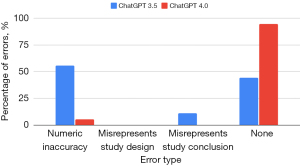
Comprehension analysis
Reviewer 1 found that ChatGPT 3.5 summaries displayed a failure of comprehension somewhat evenly across categories at a proportion of 7 of 18 articles, except for the category of “why” where ChatGPT 3.5 succeeded in representing the background of the articles in all summaries. Reviewer 1 found that ChatGPT 4.0 summaries most commonly displayed a failure of comprehension of the conclusions of the study with a proportion of 3 of 18 articles. Reviewer 1 found ChatGPT 3.5 displayed a perfect comprehension in 6 of 18 articles whereas ChatGPT 4.0 displayed a perfect comprehension in 14 of 18 articles. The results of reviewer 1’s tally of summary comprehension are displayed in Figure 3 and Table S6.
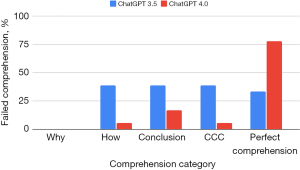
Reviewer 2 found that ChatGPT 3.5 summaries most commonly displayed a failure of comprehension of the conclusions of the article with a proportion of 11 out of 18 articles. Reviewer 2 found that ChatGPT 4.0 summaries most commonly displayed a failure of comprehension of the study design with a proportion of 4 of 18 articles. Reviewer 2 found ChatGPT 3.5 displayed a perfect comprehension in 5 of 18 articles whereas ChatGPT 4.0 displayed a perfect comprehension in 12 of 18 articles. The results of reviewer 2’s tally of summary comprehension are displayed in Figure 4 and Table S7.

An average of both reviewers’ evaluations of ChatGPT summary comprehension of articles showed that ChatGPT 4.0 outperformed ChatGPT 3.5 in all categories. Interobserver agreement between evaluators as measured by the Cohen kappa coefficient was strongest for the category of correct clinical conclusion (CCC), with a score of 0.3 for ChatGPT 3.5 and 0.64 for ChatGPT 4.0, connoting a fair and substantial agreement between evaluators. For that category, on average, the reviewers found that ChatGPT 3.5 summaries failed to display comprehension of the CCC 44% of the time, whereas ChatGPT 4.0 failed in this category 8% of the time. The results of the averaged review of comprehension failure across evaluators are displayed in Figure 5 and Table S8.
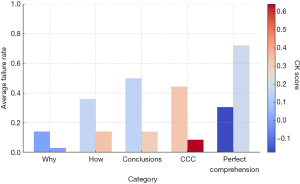
Descriptive analysis
Human evaluators with clinical experience evaluated the nature of the errors and quality of the summaries through a grading system. Though ChatGPT 4.0 less frequently made numeric errors than ChatGPT 3.5, it primarily did so by leaving out specific numbers altogether. Of note, when ChatGPT 3.5 and 4.0 provided numeric inaccuracies, they were in the appropriate range compared with the actual study’s true values. Otherwise, the cited numbers seem to be derived from other sources altogether. For example, when asked to summarize the findings in the “Saline or albumin for fluid resuscitation in patients with traumatic brain injury”, ChatGPT 3.5 confused and combined the results from that study with that of the original SAFE Study, claiming that “the study included 6,997 patients with TBI who were randomly assigned to receive either saline or albumin for fluid resuscitation” (15,16). The original SAFE Study did, in fact, include 6,997 participants, but they were ICU patients and not specifically traumatic brain injury (TBI) patients. Significantly, ChatGPT 3.5 then incorrectly synthesized the conclusions of the two studies, leading to an inaccurate clinical conclusion: “[t]he authors concluded that there was no significant difference in mortality between saline and albumin for fluid resuscitation in patients with TBI”. The relevant study did in fact find a higher mortality in TBI patients resuscitated with albumin. In the same study, ChatGPT 4.0 left out the sample size and misrepresented the results but correctly reported a higher mortality in the albumin group than the saline group [33% and 25% with risk ratio (RR) 1.32 vs. actual study values 33.2% and 20.4% with RR 1.63].
ChatGPT 3.5 and 4.0 generated inaccuracies in study conclusions in clinically reasonable ways, seemingly superimposing a general clinical understanding into its recollection of a particular study. For example, ChatGPT 3.5 and 4.0 similarly misrepresented the criteria for requiring a head computed tomography (CT) in patients with a minor head injury as put forth in “Indications for computed tomography in patients with minor head injury” (17). The actual criteria included the following seven clinical findings as indications for head CT in minor head injury: headache, vomiting, age over 60 years, drug or alcohol intoxication, deficits in short-term memory, physical evidence of trauma above the clavicles, and seizure. ChatGPT 3.5 correctly identified these seven criteria but added “loss of consciousness” and “coagulopathy” as criteria. ChatGPT 4.0 correctly identified seven clinical criteria. Still, it replaced “headache” and “drug or alcohol intoxication” with “focal neurologic deficit” and “high-impact mechanism of injury”. In both summaries, ChatGPT correctly concluded that the paper’s clinical criteria safely avoided unnecessary CT scans.
Overall, ChatGPT 4.0 responses were better structured. All ChatGPT 4.0 summaries were bulleted with well-defined subtopics, whereas no ChatGPT 3.5 summaries contained this structure.
Discussion
ChatGPT 3.5 and 4.0 have an impressive knowledge base. However, both made errors at a high rate. ChatGPT 4.0 significantly exceeded the capabilities of ChatGPT 3.5 in both quality of content and minimization of errors. The nature of the errors in ChatGPT 4.0 summaries was more in line with the overall clinical consensus on a given topic than the errors of ChatGPT 3.5, which often made statements that sounded convincing but were factually incorrect.
It is unclear what error rate is clinically significant when evaluating AI systems. Certainly, ChatGPT 4.0 already displays a breadth of understanding of available literature. However, it also confidently gives wrong answers. Compared with ChatGPT 4.0’s success rate, if requested to recite the design and results of 25 assorted medical papers across all fields of medicine, physicians would not be expected to succeed in perfectly summarizing those papers. But further, few would confidently confabulate the results of papers with which they are not familiar. We define “understanding” of available literature from a functional standpoint, conceding that LLMs have not proven to have a subjective experience of understanding but rather reply to prompts in a manner consistent with human understanding.
Though the most recent version of ChatGPT 4.0 now directly accesses online resources for information, it is important to consider the accuracy of the knowledge base that is the engine for this and future systems. It is likely that errors in clinical reasoning will be poorly tolerated by regulating bodies, and similar to autonomous vehicle regulation, a near-zero error rate may be the expectation before these systems can be put into practice. However, the regulatory agencies guiding safety in healthcare and the automotive industry are different, as is the risk profile for these two domains. By comparison, a buyer’s AI-guided car may harm other drivers for the sake of the buyer’s convenience. In contrast, a patient may choose AI diagnostics if it offers a marginal benefit to their survival without conferring risk to other parties. How healthcare systems may choose to integrate these resources is ethically complex. Applying AI models with systemic errors to clinical use for cost reduction and distributing access to potentially life-saving but costly AI models unequally offer opposing ethical choices to healthcare systems. This complexity is compounded further by the tendency of AI systems to promulgate entrenched social biases into their algorithms. For example, Kelly et al. highlighted the underperformance of AI algorithms at detecting benign and malignant moles in dark-skinned individuals due to the under-representation of these groups in the historical training data (18). Similar concerns for exacerbating healthcare disparity with use of LLMs in clinical decision support exist and are being investigated (19).
This constitutes one of the first papers directly considering the accuracy of LLMs in their understanding of medical literature. Another paper considered clinical accuracy when answering medical questions online (6). However, that paper spoke more about the ability of LLMs to communicate with patients about their care. Therefore, it did not require the same degree of accuracy as expected for making clinical decisions. In that paper, three licensed physicians graded ChatGPT and physician responses to online medical questions in a blinded comparison, defining response quality and empathy on a subjective scale. That paper found that evaluators preferred ChatGPT 3.5 to physician responses 78.6% of the time, and AI outperformed human responses in empathy and quality. As a system designed to be an effective chatbot, it is not surprising that ChatGPT gave highly empathetic responses. Chatbots have already proven effective in other industries in customer support; that paper showed that ChatGPT had enough medical knowledge to extend that capability to a medical domain. The paper showed hope that medical chatbots may remove some of the burden of patient correspondence worsening physician burnout.
Another recent paper currently in preprint tested ChatGPT 3.5 on a variety of medical questions from various medical fields, answers of which were evaluated for correctness and completeness. They reported that AI answers were graded as “correct” or “nearly all correct” over 50% of the time but that there were some instances in which the chatbot was “spectacularly and surprisingly wrong” (20). These findings also match our experience, with ChatGPT 3.5 and 4.0 usually getting the majority of the appropriate clinical conclusions correct but with instances of critically wrong answers.
This study has a number of limitations. Only two physician evaluators were used to judge the summaries. Where one could expect differences in interpretation of the ChatGPT summaries by additional evaluators, it would be unexpected that the data regarding the CCCs should change significantly. There is not much room for interpretative error when ChatGPT 3.5 claims “there was no significant difference in mortality between saline and albumin for fluid resuscitation in patients with TBI” when the literature, in fact, stated that for “patients with traumatic brain injury, fluid resuscitation with albumin was associated with higher mortality rates than was resuscitation with saline”. As these systems become further refined it is possible that a full panel of experts will be needed to grade the accuracy of these systems more rigorously. Additionally, blinding the evaluator to the source of the summary, whether ChatGPT 3.5 or 4.0, could have removed bias in comparing the two LLMs. Should future researchers wish to pursue this design, prompt engineering to require the output from the two versions to appear similar will be necessary. As ChatGPT 4.0 responses differed in format and style from ChatGPT 3.5, blinding may be ineffective. Further, each summarization prompt was only attempted once. Given that a given LLM may respond variably to the same prompt, this design may underestimate the degree of error to which ChatGPT responds to a medical summarization prompt. Our hope was that such variability would reveal itself across publication summaries. Future studies may wish to repeat the same prompt multiple times on a given LLM to assess stability. Another limitation of this study is that we confined the analysis to medical literature under the domain of “trauma”, which may not evaluate the performance of ChatGPT across all medical topics. Although the keyword chosen was “trauma”, this search resulted in papers from a wide array of medical topics, including trauma, critical care, orthopedic surgery, preventative medicine and post-injury psychiatric support. We chose the general topic of trauma because the physician evaluators’ expertise was in trauma. See Table S1 for a complete listing of the papers evaluated. Though we chose to test ChatGPT due to its gaining popularity and competitive level of performance, future studies may wish to compare its accuracy with other available LLMs, specifically those optimized for research and medical decision support. An additional source of bias in this paper is our choice to exclude the four papers that ChatGPT 4.0 declined to summarize. At first sight, declining to confabulate a summary it was not trained on implies credibility as a source of information. It is possible, however, that ChatGPT 4.0 may have hallucinated a lack of training on these papers. Alternatively, including these four papers and giving ChatGPT a score of 0 on metrics of comprehension could have skewed the bias in the opposite direction, favoring ChatGPT 3.5 hallucinations that correctly extrapolated the details of these studies from their titles.
Previous works have considered the tendency of LLMs to memorize text from their datasets (21) and the inherent biases in which texts they tend to memorize (22). Those studies found that texts were more frequently memorized the more they were found in the LLM dataset and that literature in the public domain was more frequently memorized, respectively. Our study compares inaccuracies between ChatGPT 3.5 and 4.0; however, misrepresenting a number from a study, for example, more accurately reflects two independent errors: a tendency to hallucinate information and a failure to memorize information. Our hope was to minimize the latter component of this error by choosing medical literature in a prominent journal with a high citation rate. This implies a bias in our study towards better memorization performance, and therefore, when generalized to less prominent journals, the clinician may expect a greater number of inaccuracies.
Clinicians must judge the validity of sources of information before applying them to practice. However, LLMs offer new challenges: first, the process of acquiring clinical information and understanding is neither peer-reviewed nor externally tested. Second, the process of forming clinical recommendations for specific scenarios is opaque. Though ChatGPT may explain how it arrived at a clinical decision, its responses are simply designed to be evaluated as high-quality content. They may not reflect the probabilistic and subjective mechanism by which a prompt becomes a response.
Conclusions
The error rate of ChatGPT 4.0 is currently too high to rely on its understanding of medical literature fully. Future studies should consider validating the accuracy of newer versions of ChatGPT and other LLMs that now have access to online resources. Additional studies may wish to validate LLMs’ ability to formulate a clinical plan for specific patient scenarios. This could be compared in a blinded fashion with plans produced by physicians relevant to the field of inquiry and judged by senior physicians in those fields. Such studies should also consider gender, racial, and socioeconomic factors inserted into the clinical prompt and measure biases in their response to avoid promulgating entrenched biases into AI-supported care.
Acknowledgments
Michael Menke provided data analysis, which was incomplete for our purposes and ultimately not used in the paper.
Funding: Financial support for this study was provided in part by
Footnote
Data Sharing Statement: Available at https://jmai.amegroups.com/article/view/10.21037/jmai-24-48/dss
Peer Review File: Available at https://jmai.amegroups.com/article/view/10.21037/jmai-24-48/prf
Conflicts of Interest: All authors have completed the ICMJE uniform disclosure form (available at https://jmai.amegroups.com/article/view/10.21037/jmai-24-48/coif). All authors are employed by HonorHealth. The authors have no other conflicts of interest to declare.
Ethical Statement: The authors are accountable for all aspects of the work in ensuring that questions related to the accuracy or integrity of any part of the work are appropriately investigated and resolved.
Open Access Statement: This is an Open Access article distributed in accordance with the Creative Commons Attribution-NonCommercial-NoDerivs 4.0 International License (CC BY-NC-ND 4.0), which permits the non-commercial replication and distribution of the article with the strict proviso that no changes or edits are made and the original work is properly cited (including links to both the formal publication through the relevant DOI and the license). See: https://creativecommons.org/licenses/by-nc-nd/4.0/.
References
- Samuel AL. Some studies in machine learning using the game of Checkers. IBM Journal of Research and Development 1959;3:210-29. [Crossref]
- Hopfield JJ. Neural networks and physical systems with emergent collective computational abilities. Proc Natl Acad Sci U S A 1982;79:2554-8. [Crossref] [PubMed]
- Arnold L, Rebecchi S, Chevallier S, et al. An Introduction to Deep Learning. The European Symposium on Artificial Neural Networks (ESANN), Bruges, Belgium; Apr 2011.
- Ghojogh B, Ghodsi A. Attention Mechanism, Transformers, Bert, and GPT: Tutorial and Survey. (2020). Available online: https://api.semanticscholar.org/CorpusID:242275301
- Kung TH, Cheatham M, Medenilla A, et al. Performance of ChatGPT on USMLE: Potential for AI-assisted medical education using large language models. PLOS Digit Health 2023;2:e0000198. [Crossref] [PubMed]
- Ayers JW, Poliak A, Dredze M, et al. Comparing Physician and Artificial Intelligence Chatbot Responses to Patient Questions Posted to a Public Social Media Forum. JAMA Intern Med 2023;183:589-96. [Crossref] [PubMed]
- Alkaissi H, McFarlane SI. Artificial Hallucinations in ChatGPT: Implications in Scientific Writing. Cureus 2023;15:e35179. [Crossref] [PubMed]
- Walters WH, Wilder EI. Fabrication and errors in the bibliographic citations generated by ChatGPT. Sci Rep 2023;13:14045. [Crossref] [PubMed]
- Flanagin A, Bibbins-Domingo K, Berkwits M, et al. Nonhuman "Authors" and Implications for the Integrity of Scientific Publication and Medical Knowledge. JAMA 2023;329:637-9. [Crossref] [PubMed]
- Ji Z, Lee N, Frieske R, et al. Survey of hallucination in natural language generation. ACM Computing Surveys 2023;55:1-38. [Crossref]
- Jin Q, Leaman R, Lu Z. Retrieve, Summarize, and Verify: How Will ChatGPT Affect Information Seeking from the Medical Literature? J Am Soc Nephrol 2023;34:1302-4. [Crossref] [PubMed]
- Lozano A, Fleming SL, Chiang CC, et al. Clinfo.ai: An Open-Source Retrieval-Augmented Large Language Model System for Answering Medical Questions using Scientific Literature. Pac Symp Biocomput 2024;29:8-23. [PubMed]
- Fleming SL, Lozano A, Haberkorn WJ, et al. MedAlign: A Clinician-Generated Dataset for Instruction Following with Electronic Medical Records. Proceedings of the AAAI Conference on Artificial Intelligence 2024;38:22021-30. [Crossref]
- Tang L, Sun Z, Idnay B, et al. Evaluating large language models on medical evidence summarization. NPJ Digit Med 2023;6:158. [Crossref] [PubMed]
- SAFE Study Investigators. Saline or albumin for fluid resuscitation in patients with traumatic brain injury. N Engl J Med 2007;357:874-84. [Crossref] [PubMed]
- Finfer S, Bellomo R, Boyce N, et al. A comparison of albumin and saline for fluid resuscitation in the intensive care unit. N Engl J Med 2004;350:2247-56. [Crossref] [PubMed]
- Haydel MJ, Preston CA, Mills TJ, et al. Indications for computed tomography in patients with minor head injury. N Engl J Med 2000;343:100-5. [Crossref] [PubMed]
- Kelly CJ, Karthikesalingam A, Suleyman M, et al. Key challenges for delivering clinical impact with artificial intelligence. BMC Med 2019;17:195. [Crossref] [PubMed]
- Yu P, Xu H, Hu X, et al. Leveraging Generative AI and Large Language Models: A Comprehensive Roadmap for Healthcare Integration. Healthcare (Basel) 2023;11:2776. [Crossref] [PubMed]
Johnson D Goodman R Patrinely J Assessing the Accuracy and Reliability of AI-Generated Medical Responses: An Evaluation of the Chat-GPT Model. (2023 ). Available online:10.21203/rs.3.rs-2566942/v1 - Carlini N, Ippolito D, Jagielski M, et al. Quantifying Memorization Across Neural Language Models. Published as a conference paper at ICLR (2023). arXiv 2022. doi:
10.48550 /arXiv.2202.07646. - Chang K, Cramer M, Soni S, et al. Memory: An Archaeology of Books Known to ChatGPT/GPT-4. Proceedings of the 2023 Conference on Empirical Methods in Natural Language Processing (2023). Available online: https://aclanthology.org/2023.emnlp-main.453
Cite this article as: Weinberg J, Goldhardt J, Patterson S, Kepros J. Assessment of accuracy of an early artificial intelligence large language model at summarizing medical literature: ChatGPT 3.5 vs. ChatGPT 4.0. J Med Artif Intell 2024;7:33.