Comprehensive guide and checklist for clinicians to evaluate artificial intelligence and machine learning methodological research
Highlight box
Key findings
• A new 30-item checklist has been developed and validated to evaluate artificial intelligence (AI) and machine learning (ML) research in healthcare, offering a structured approach to assess quality and reliability.
• This checklist encompasses a broad range of criteria, including study design, data acquisition, data processing, analysis methods, and reporting, making it applicable across various types of AI/ML studies.
What is known and what is new?
• Previous guidelines for AI and ML in healthcare focus on specific study types, such as clinical trials or diagnostic accuracy. This new checklist, however, takes a broader view, incorporating criteria that are relevant to a wider array of studies.
• The comprehensive nature of this checklist distinguishes it from other guidelines, providing clinicians and researchers with a more robust tool to critically appraise AI and ML research in clinical medicine.
What is the implication, and what should change now?
• The availability of this checklist is expected to enhance clinicians' ability to critically assess AI and ML research, promoting improved evaluation practices and contributing to greater transparency in healthcare studies.
• This checklist may lead to more informed decision-making regarding the adoption and implementation of AI technologies in healthcare settings, fostering a culture of rigorous evaluation and best practices in clinical research.
Introduction
Background
Artificial intelligence (AI) and machine learning (ML) are burgeoning fields with substantial implications for enhancing patient care, augmenting clinical decision-making, and optimizing healthcare costs. While the term “Artificial Intelligence” was coined as far back as the 1950s, its integration into healthcare systems began in earnest during the early 1970s (1). AI and ML applications span virtual and physical healthcare domains, primarily characterized by ML, a data-driven algorithmic approach that emulates human cognition (2). Deep learning (DL), a subset of ML, employs neural networks to mimic human cognitive processes with minimal human intervention (3). These technologies have showcased proficiency in medical imaging, aiding in data extraction and complex case resolution. The relationships among AI, ML, and DL are further elucidated in Figure 1. On the physical front, AI contributions manifest in robotic technologies and AI-assisted surgical procedures fostering cost-effective, precise treatment options, streamlined diagnostics, and expedited drug discovery (4-6). To ensure a seamless transition of healthcare professionals into this new paradigm, it is essential to disseminate foundational knowledge. Table 1 elucidates key terminologies and acronyms common in AI applications, while Table 2 enumerates essential resources for clinicians aspiring to gain in-depth understanding of AI and related disciplines (7,8).
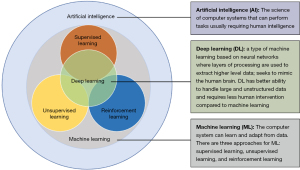
Table 1
Term | Definition |
---|---|
AI | The science of computer systems that can perform tasks usually requiring human intelligence |
AI intervention | A health intervention by artificial intelligence or machine learning to serve its purpose |
ANI | Most AI systems today are ANI, which means they can only perform certain tasks, and knowledge gain from the tasks cannot be applied to other tasks |
Algorithm | Series of steps to perform an action |
Class activation map | A visualization of pixels that had the greatest influence on the predicted class; displays gradient of predicted outcome from model with respect to input |
DL | Type of machine learning based on neural networks where layers of processing are used to extract higher level data; seeks to mimic the human brain |
NLP | A subfield of AI that uses algorithm to understand human language as it is spoken and written |
Human-AI interaction | Process in how users interact with AI intervention, so it functions as intended |
ML | A field of computer science that uses algorithms that can solve tasks by learning patterns from data |
NN | Teaches a system to process data like a human brain does; necessary for deep learning |
Pattern recognition | Where system recognizes patterns in data, optimizing outcomes |
Performance error | When AI intervention does not perform as expected |
AI, artificial intelligence; ANI, artificial narrow intelligence; DL, deep learning; NLP, natural language processing; ML, machine learning; NN, neural networks.
Table 2
Course | Learning objectives |
---|---|
AI for healthcare | • Application of AI to 2D medical imaging data |
• Application of AI to 3D medical imaging data | |
• Application of AI to EHR data | |
• Application of AI to wearable device data | |
AI for medicine specialization | • Estimation of treatment effects using data from randomized control trials |
• Exploration of methods to interpret diagnostic and prognostic models | |
• Application of natural language processing to extract information from unstructured medical data | |
AI in healthcare specialization | • Identification of problems healthcare providers face that machine learning can solve |
• Analyzation of how AI affects patient care safety, quality, and research | |
• Relation of AI to science, practice, and business of medicine | |
• Application of building blocks of AI to help innovate and understand emerging technologies | |
Statistical analysis with R for public health specialization | • Recognition of key components of statistical thinking to defend the critical role of statistics in modern public health research and practice |
• Description of a given data set from scratch using descriptive statistics and graphical methods as a first step for more advanced analysis using R software | |
• Application of appropriate methods to formulate and examine statistical associations between variables within a data set in R | |
• Interpretation of output from analysis and appraisal of the role of chance and bias as explanations for results | |
Fundamentals of machine learning for healthcare | • Ethical use of machine learning technology in healthcare |
• Best practices for development and deployment of machine learning systems in healthcare | |
• Common challenges and pitfalls in developing machine learning applications for healthcare | |
AI for medical diagnosis | • Disease detection with computer vision |
• Evaluation of models | |
• Image segmentation on MRI images | |
Biostatistics in public health specialization | • Calculation summary statistics from public health and biomedical data |
• Interpretation of written and visual presentations of statistical data | |
• Evaluation and interpretation of results of various regression methods | |
• How to choose the most appropriate statistical methods to answer a research question |
AI, artificial intelligence; ML, machine learning; 2D, two-dimensional; 3D, three-dimensional; EHR, electronic health record; MRI, magnetic resonance imaging.
While AI and ML offer utility beyond traditional healthcare, their applications in medicine revolutionize retail and pharmaceutical operations, facilitating efficiency, accuracy, and innovation. For instance, the algorithm ‘aTarantula’ was developed to scan social media platforms for adverse events related to Warfarin, achieving high sensitivity and specificity in its findings (9). Other notable examples include AI algorithms in medication management, ML techniques expediting drug discovery, and clinical decision support systems enhancing patient safety (10-13). The integration of AI and ML promotes individualized, evidence-based patient care in healthcare, heralds a transformative era with lasting implications (14-17). Despite the increasing prevalence of AI and ML studies in the field, clinicians exhibit reluctance in adopting AI (18). This hesitancy contributes to a notable deficiency among clinicians in interpreting and evaluating research studies. This deficiency not only compromises the quality of patient care but could also hinder innovation within the healthcare field (19). The development and implementation of a structured, easy-to-follow methodology for evaluating AI and ML articles is more than an academic exercise; it is a critical step towards ensuring that clinicians can effectively contribute to the intelligent application of these technologies in real-world patient care. Establishing a robust evaluation mechanism aligns with the scientific rigor and evidence-based approach that underpins the healthcare profession, fostering an environment where technology and clinical expertise merge to enhance patient outcomes.
Rationale and knowledge gap
The American Society of Health-System Clinicians (ASHP) has issued a statement underscoring the distinctive role of clinicians in the realm of AI-assisted medical interventions (20). Similarly, the American Medical Association (AMA) released new principles to guide the development, deployment, and use of augmented intelligence in healthcare (21). These principles focus on promoting a consistent governance structure that prioritizes ethics, equity, responsibility, and transparency in AI applications. AMA President emphasized the need for a principled approach to AI oversight and governance due to its potential to enhance diagnostic accuracy and patient care, but also due to associated ethical considerations and risks. Clinicians not only have the potential to enhance the quality of healthcare through the application of AI and ML in their daily practice but can also be instrumental in the design, implementation, and analysis of AI-centric applications in the medication usage cycle (22-24). Hence, it is imperative for clinicians to acquire a foundational understanding of AI and ML technologies, and critically evaluate their accuracy and interpretability as presented in primary research literature.
To address this, there is a necessity in the realm of healthcare to adopt comprehensive reporting guidelines. Tailored reporting guidelines are crucial due to the unique nature of AI/ML studies in healthcare, the need for standardization, and the critical importance of reducing bias in medical research. Enhanced reporting frameworks would facilitate clinicians in appraising the utility of AI and ML in patient care with rigorous scientific scrutiny (25).
Objective
The overarching objective of this research is to establish and rigorously develop and apply an evidence-based guideline checklist designed for clinicians, bridging the gap between theoretical knowledge of AI and ML and practical strategies for daily clinical operations. The expected result of implementing this framework is to empower clinicians, enabling them to understand and apply AI and ML effectively, contributing to the progress of clinical innovation and improving patient outcomes.
Methods
Overview of methodological framework
This study adopts a multi-faceted approach aimed at bridging the gap between AI/ML technologies and clinical practices. The multi-faceted approach includes a systematic review of existing guidelines, the development of a checklist, and the application of the checklist to a diverse set of AI/ML studies. The method encompasses study design, data collection, criteria for guideline inclusion and exclusion, evaluation of these guidelines, checklist development, validation, and statistical analysis to offer a panoramic view of the research process.
Study design
A systematic review and evaluation of existing guidelines for AI and ML applications in healthcare were conducted with the aim of developing a comprehensive evaluative checklist for clinicians.
Data collection
Key guidelines like MI-CLAIM, MINIMAR, DECIDE-AI, SPIRIT-AI, CONSORT-AI, STARD-AI, and TRIPOD-AI were reviewed. We conducted an extensive search across several reputable databases, including PubMed, IEEE Xplore, ACM Digital Library, and Google Scholar. These platforms were chosen for their comprehensive coverage of scientific and academic publications, particularly those related to AI/ML research in healthcare. We only included journals and article in English. We searched the databases from January 1st, 2010 through April 15th, 2024.
To ensure a thorough review, we applied specific search criteria to identify guidelines relevant to AI/ML applications in healthcare. This process involved using keywords and terms that focused on AI, ML, clinical medicine, and related topics. After compiling a list of potential guidelines, we conducted an in-depth evaluation to determine which ones met the criteria for inclusion in our study.
Criteria for inclusion and exclusion
To ensure a robust and thorough assessment, we formulated explicit criteria for the inclusion and exclusion of guidelines. Inclusion criteria required guidelines to demonstrate a targeted focus on healthcare relevant to clinical practice. We emphasized the need for comprehensive guidance, ensuring a well-rounded approach to guideline content that addresses various aspects crucial for robust research. The inclusion of peer-reviewed guidelines adds an additional layer of quality assurance, as it involves assessment by experts in the field to validate the relevance and credibility of the guidelines. Also, the criteria that guidelines should be published in reputable journals is important due to their recognition for high editorial standards, ethical practices, and commitment to disseminating credible and impactful research were also an important criteria.
The inclusion criteria were as follows: Guidelines were considered for inclusion if they met the following specific criteria: explicitly designed for AI and ML studies in healthcare relevant to clinical practice; provided comprehensive guidance on study design, data acquisition, data processing, data analysis, and reporting methods; peer-reviewed and published in reputable journals. We only included primary journals that are peer reviewed and from reputable high impact journals. The journals were defined using several key criteria: adherence to high editorial standards, rigorous peer review processes, ethical practices, impact factor, and recognition by professional bodies. These factors ensure that journals maintain quality, integrity, and credibility. The editorial standards include thorough peer review by experts to validate research accuracy, while ethical practices ensure transparency and compliance with established research guidelines. The impact factor indicates the journal’s influence, with higher numbers reflecting broader acceptance in the academic community. Recognition by respected professional organizations further confirms the journal’s standing.
The exclusion criteria were as follows: Guidelines were excluded if they met any of the following conditions: not specifically designed for AI and ML studies in healthcare relevant to clinical practice; lacked a focus on study design, data acquisition, processing, analysis, or reporting; not peer-reviewed or were published in non-reputable sources.
Evaluation of guidelines
In addressing the validation of the checklist, a comprehensive approach was undertaken to ensure the dimensions of quality, validity, and robustness were rigorously evaluated. To ensure quality, the checklist is comprised of carefully chosen parameters and questions based on existing guidelines that are considered standards in the field. Furthermore, it was reviewed by a panel of experts specializing in both healthcare and AI to affirm its content validity. A scoring system was also established to quantitatively measure study quality, where a higher score is indicative of better quality. To add another layer of rigor, inter-rater reliability was tested using the Cronbach’s alpha, ensuring that the checklist produces consistent and reliable results across different users. As for validity, a two-step validation process was employed. Initially, the checklist was applied to a pilot set of five studies, and the results were reviewed by domain experts to establish the tool’s face validity. Subsequently, the checklist was used in a larger, more diverse sample of 50 studies. The scores derived from the checklist were then correlated with external validity metrics, such as citation count and journal impact factor, thereby establishing criterion validity. Finally, robustness was addressed through sensitivity analyses that assessed how variations in checklist scoring criteria could impact the overall study score. The sensitivity analysis in this study aimed to evaluate the robustness of our findings. We focused on how changes in checklist scoring criteria affected the total scores, and we tested variations in data subsets to understand their influence on results. Stability tests of the findings across multiple datasets were also conducted to ensure that the checklist was not overly sensitive to outliers or specific variables.
Methodology in the development of checklist
A checklist of 30 questions was synthesized from the guideline evaluation, designed for clinicians to systematically assess the quality and integrity of AI/ML studies. The motivation behind developing the 30-item checklist for evaluating AI and ML research in healthcare arises from the need for a standardized and comprehensive method to assess the quality, reliability, and robustness of such studies. The checklist was created to bridge the gap between AI/ML technologies and clinical practices, helping clinicians critically evaluate research outcomes and identify potential biases or inconsistencies.
The 30-item checklist aims to standardize and streamline the evaluation of AI and ML research within healthcare. By providing a comprehensive framework, it helps clinicians assess the quality and reliability of studies through a consistent scoring system. The checklist addresses critical aspects of AI and ML research, including study design, data acquisition, data processing, analysis methods, and reporting. This structured approach fosters reproducibility and objectivity, enabling clinicians to identify potential biases and ensure research integrity.
Validation and scoring system
To ensure a comprehensive and representative analysis, we carefully selected 50 relevant studies exploring AI and ML applications within clinical medicine. This diverse sample aimed to capture the breadth of research in this rapidly evolving field. A scoring system was established to quantify the quality of the studies assessed. The score was out of a possible 30 points where a higher score is indicative of better quality and the average and standard of deviation were assessed.
Statistical analysis
Descriptive statistics summarized the validation scores. A P value of less than 0.05 determined statistical significance. This study employed hypothesis testing to assess the statistical significance of outcomes based on the checklist scoring system, with a P value indicating the probability that observed results could happen randomly if the null hypothesis were true. A P value threshold of less than 0.05 was used to determine statistical significance; when the P value falls below this threshold, it suggests that the results are unlikely due to chance, leading to the rejection of the null hypothesis. This approach provided confidence in the study’s findings and supported the validity of the checklist in evaluating AI and ML research, as it indicated that the observed differences were statistically significant. This threshold was chosen to determine whether observed differences in validation scores were likely due to actual differences in adherence to the checklist criteria rather than random chance. The P value served as a valuable quantitative indicator to assess the reliability and generalizability of our findings. Sensitivity analyses were also conducted to validate our findings.
Results
Identification and selection of guidelines
An extensive search led to the identification of 57 potential AI and ML reporting guidelines. After eliminating 12 duplicates, 45 guidelines underwent an in-depth review based on their abstracts and titles. This primary screening removed 28 guidelines that did not match the inclusion criteria. A subsequent full-text review of the remaining 17 guidelines was conducted, which further excluded 10 as they did not offer specific guidance for AI or ML applications within healthcare. Therefore, seven guidelines (MI-CLAIM, MINIMAR, DECIDE-AI, SPIRIT-AI, CONSORT-AI, STARD-AI, and TRIPOD-AI) were shortlisted for a comprehensive evaluation.
Evaluation of guidelines
Overall, our analysis revealed that only a handful of guidelines provided satisfactory advice on the specific aspects of clinical practice. It is essential to recognize that each reporting guideline has its unique features and biases. Clinical trials have some of the most robust evidence for safety and effectiveness due to randomization, especially among AI and ML interventions. AI intervention studies can provide strong evidence for regulators, payers, and policymakers to establish a safety profile for the AI and ML intervention technologies (26). SPIRIT-AI and CONSORT-AI are guidelines developed to address the validity of robustness for AI and ML studies. Some examples of clinical trial elements these guidelines look at include the algorithm version, the procedure for acquiring and processing data, and inclusion criteria on the level of participants (7). The diagnostic accuracy studies aim to achieve diagnostic preciseness similar to expert clinicians while reducing unnecessary healthcare resources. The STARD-AI guideline should be used to interpret these AI-enabled diagnosis studies (27). Another type of AI intervention study is the prediction model study. The prediction model study estimates the likelihood of an individual having or developing a disease using predictor variables (26). The TRIPOD-AI guideline has been developed for these trials and should be used for similar studies (28). A complete summary of these guidelines can be found in Table 3 (7,27,28-32).
Table 3
Type of study | AI reporting guideline | Guideline information | When to use this guideline |
---|---|---|---|
Clinical trial | SPIRIT-AI | First global standards for reporting AI studies | • When a clinical trial requires detailed and specific reporting: |
CONSORT-AI | o Algorithm version | ||
o Procedure for input data | |||
o Criteria for inclusion | |||
Diagnostic accuracy study | STARD-AI | This AI extension is used alongside STARD 2015 guidelines | • To aid in the comprehensive reporting of research that uses AI techniques to assess diagnostic test accuracy and performance |
• Can be used for either single or combined data | |||
• May also be used within studies which report on image segmentation and other relevant data classification techniques | |||
Prediction model study | TRIPOD-AI | This AI extension is used alongside TRIPOD 2015 guidelines | • If the study is either developing, validation, or updating a multivariable prediction model which produces an individualized probability of developing a condition |
Other | MI-CLAIM | Published to improve reporting information regarding clinical AI algorithms | • Tool for users to be informed about AI algorithms that ensures that information about how it was developed and validated is clearly and comprehensively reported |
MINIMAR | Designed for studies reporting the use of AI systems in healthcare | • For descriptions of minimum information necessary to understand intended predictions, target population, and hidden biases, and the ability to generalize these emerging technologies | |
DECIDE-AI | Purpose is to improve the evaluation and reporting of human factors in clinical AI studies | • Use in early stage, small-scale clinical studies of AI interventions and AI-based decision support systems, independent from the study design chosen |
AI, artificial intelligence.
Outcome on the development and validation of the checklist
The review and evaluation of the existing guidelines resulted in the creation of a 30-item checklist. This checklist was specifically designed to assess the reporting quality of AI and ML studies. It covers aspects of study design, data acquisition, data processing, methods of analysis, and reporting of results and interpretation. To validate the checklist, it was applied to a representative sample of 50 recent AI and ML studies. We included peer-revived journal articles from January 1st, 2010 through April 15th, 2024.
This sample encompassed a wide range of AI and ML applications, including both observational and experimental studies. During the validation process, each item on the checklist was meticulously applied to assess the reporting quality of the selected studies. This involved a detailed examination of how well each study adhered to the predefined criteria, providing insights into the checklist’s effectiveness in evaluating a wide range of AI and ML applications in healthcare.
Scoring results
During the validation phase, total scores varied, ranging from 16 to 29 out of a possible 30 points. The total score is a composite measure derived from the 30-item checklist used to evaluate AI and ML studies in healthcare. Each item on the checklist is scored with a ‘yes’ or ‘no’ based on whether the study meets the specific criteria outlined in the checklist. A ‘yes’ response adds one point to the total score, while a ‘no’ response adds none. The average score was 22.8, with a standard deviation of 3.2. Although the majority of studies scored high in areas of data acquisition, data processing, and result reporting, many lacked detailed descriptions of their study design and methods of analysis. To add granularity to the results, the checklist was further categorized into subsections: Study Design, Data Acquisition, Data Processing, Methods of Analysis, and Reporting of Results. When comparing the scoring results with existing guidelines like MI-CLAIM, MINIMAR, DECIDE-AI, SPIRIT-AI, CONSORT-AI, STARD-AI, and TRIPOD-AI, the checklist appeared to demand more comprehensive details, especially in the areas of study design and methods of analysis (7,27,28-32). This could explain why the average score was relatively moderate at 22.8. While many studies performed well in areas like data acquisition and data processing, the overall results indicated lower scores in study design and methods of analysis. This outcome suggests that certain aspects of AI/ML studies in healthcare require more attention and rigorous reporting standards. The sensitivity analysis indicated that studies with inadequate descriptions of study design and analysis methods scored on average 3.8 points lower (P<0.01), underlining the importance of these elements in the evaluation of AI and ML studies. Such a comparison with existing guidelines underscores the unique emphasis placed on study design and analysis methods in our developed checklist. The results stress the urgent need for more rigorous and transparent reporting in AI and ML studies.
The checklist’s scoring system revealed specific areas where studies often failed to meet the required criteria. Among the most frequently failed checklist items were detailed descriptions of study design, indicating that many studies lacked a comprehensive outline of their methodologies. Additionally, inadequate explanations of data processing methods were common, pointing to a need for clearer reporting on how data was handled and analyzed. Similarly, unclear descriptions of analysis techniques affected the reproducibility and validity of the studies, emphasizing the importance of detailed reporting.
Discussion
This study stands out in its pioneering approach to bridging the gap between the complexities of AI and ML technologies and the practical needs of clinicians. Unlike previous works, it acknowledges the critical role that clinicians play in healthcare and recognizes their need for specialized tools to engage with the rapidly evolving field of AI and ML. The 30-item checklist presented in this research is a novel contribution, meticulously crafted to serve as a practical guide for clinicians. The checklist offers a structured approach to evaluating AI and ML studies, with clear criteria to guide clinicians. The study establishes the checklist as a comprehensive and versatile tool applicable across various healthcare contexts.
The checklist allows clinicians to assess AI and ML studies, providing insights that can guide their evaluation process. The assessment of standard guidelines can be reproduced within all areas of healthcare. The cooperative game theoretic metrics, such as Shapley values, contribute to making the model more interpretable, enhancing its practicality for clinicians (29). The combination of thorough theoretical foundation, practical application, and a focus on an often-overlooked aspect of healthcare technology integration sets this study apart, making a distinctive and valuable contribution to both the field of medicine and the broader healthcare community.
There is a great need for further implementation of AI and ML in all aspects of healthcare. Clinicians need to effectively manage patients’ medical therapy and improve health outcomes in this era of massive data (33). AI and ML can utilize the data to reduce biases and improve decision-making by providing real-time life-saving data to clinicians and patients. The advent of AI and ML has revolutionized treatment planning, drug discovery, and therapeutic management. The study acknowledges the current proficiency gap among clinicians in critically appraising these technologies and positions the checklist-based guidance tool as a crucial resource to bridge this gap. This 30-item checklist not only ensures a standardized and rigorous evaluation of AI and ML studies but also fortifies the clinicians’ capacity to discern potential flaws, biases, and inconsistencies that could undermine study outcomes. The emphasis on statistical robustness, clear delineation of methodologies, and comprehensive scrutiny of data types and outcome variables will undoubtedly enrich the clinicians’ understanding and confidence in employing these technologies. The checklist helps clinicians evaluate AI and ML studies, but a deeper understanding of AI requires additional resources and education. Future research should be directed towards further refining this checklist and advocating for its widespread implementation. The checklist shown in Table 4, contains a total of 30 questions that clinicians can use to assess the integrity of a study methodically. Although some questions include multiple answers, if any of those answers are checked “yes”, then the question is counted in the score. Using the checklist, the clinicians can evaluate if potential biases diminish the significance of study results. By placing particular importance on study design and analysis methods, the checklist offers a valuable resource for advancing the robustness and transparency of AI and ML studies within the healthcare landscape.
Table 4
Requirement | Yes | No |
---|---|---|
1. Is the study population described in terms of inclusion and exclusion criteria? | □ | □ |
2. Is the study design described (e.g., retrospective, prospective, etc.)? | □ | □ |
3. Is the study setting described (e.g., primary care, ambulatory care, etc.)? | □ | □ |
4. Is the source of the data described (e.g., laboratory information system, EHR, etc.)? | □ | □ |
5. Is the medical task reported (e.g., diagnostics)? | □ | □ |
6. Is the data collection process described in terms of setting-specific data collection strategies? | □ | □ |
7. Are subject demographics described in terms of: | ||
• Average age | □ | □ |
• Age variability | □ | □ |
• Gender breakdown | □ | □ |
• Main comorbidities | □ | □ |
• Ethnic group | □ | □ |
• Socioeconomic status | □ | □ |
8. If the task is supervised, is the gold standard described? Authors should describe the following processes: | ||
• Number of annotators producing labels | □ | □ |
• Their profession and expertise | □ | □ |
• Instructions for quality control | □ | □ |
• Inter-rater agreement score | □ | □ |
• Labeling technique | □ | □ |
9. In case of tabular data, are the features described? | ||
• If continuous: unit of measure, range, mean, and standard deviation | □ | □ |
• If nominal: all codes, values, and descriptions | □ | □ |
10. Is outlier detection and analysis performed and reported? | □ | □ |
11. Is missing-value management described? | ||
• Missing rate for each feature reported | □ | □ |
• Technique of imputation, if any, is described and reasons for its choice given | □ | □ |
12. Is feature pre-processing performed and described? | □ | □ |
13. Is data imbalance analysis and adjustment performed and reported? | □ | □ |
14. Is the model task reported? | ||
• Binary classification | □ | □ |
• Multi-class classification | □ | □ |
• Ordinal regression | □ | □ |
• Continuous regression | □ | □ |
• Clustering | □ | □ |
• Dimensionality reduction | □ | □ |
• Segmentation | □ | □ |
15. Is the model output specified (examples below)? | ||
• Disease positivity probability score | □ | □ |
• Probability of infection | □ | □ |
• Postoperative pain scores | □ | □ |
16. Is the model architecture or type described? | ||
• SVM | □ | □ |
• Random forest | □ | □ |
• Boosting | □ | □ |
• Logistic regression | □ | □ |
• Nearest neighbors | □ | □ |
• Convolutional neural network | □ | □ |
17. Is the data splitting described? | ||
• In case of data splitting, authors stated that splitting was performed before any pre-processing steps or model construction steps to avoid data leakage and overfitting | □ | □ |
18. Is the model training and selection described? | ||
• Range of hyperparameters | □ | □ |
• Method used to select the best hyperparameter configuration | □ | □ |
• Full specification of the hyperparameters used to generate results | □ | □ |
• Procedure (if any) to limit over-fitting | □ | □ |
19. Is the model calibration described? | ||
• If yes, Brier score must be reported, and a calibration plot presented | □ | □ |
20. Is the internal/internal-external model validation procedure described (examples below)? | ||
• Internal 10-fold cross-validation | □ | □ |
• Time-based cross-validation | □ | □ |
21. Has the model been externally validated? | ||
• If yes, the characteristics of the external validation set must be described | □ | □ |
22. Are the main error-based metrics used? | ||
• Classification performance: accuracy, balanced accuracy, specificity, sensitivity (recall), area under the curve | □ | □ |
• Regression performance: of: R2, mean absolute error, root mean square error, mean absolute percentage error or the ratio between mean absolute error, and SD | □ | □ |
• Clustering performance: external validation metrics and internal validation metrics | □ | □ |
• Image segmentation performance: accuracy-based metrics, distance-based metrics, or area-based metrics | □ | □ |
• Reinforcement learning performance: fixed-policy regret, dispersion across time, dispersion across runs, risk across time, risk across runs, dispersion across fixed-policy rollouts, risk across fixed-policy rollouts | □ | □ |
23. Are some relevant errors described? | □ | □ |
24. Is the target user indicated (e.g., clinician)? | □ | □ |
25. Is the utility of the AI model discussed? | □ | □ |
26. Is information regarding model interpretability and explanation available? | ||
• Feature importance | □ | □ |
• Interpretable surrogate models | □ | □ |
• Shapely value calculations were mentioned | □ | □ |
27. Is there any discussion regarding model fairness, ethical concerns or risks of bias? | □ | □ |
28. Is any point made about the environmental sustainability of the model, or about the carbon footprint of either the training phase or inference phase of the model? | □ | □ |
29. Is code and data shared with the community? | ||
• If not, are there reasons given? | □ | □ |
30. Is the system already adopted in daily practice? | □ | □ |
AI, artificial intelligence; ML, machine learning; EHR, electronic health record; SVM, support vector machine; SD, standard deviation.
Mastery of AI and ML is no longer optional but essential for clinicians to navigate a rapidly changing landscape. Incorporating AI and ML into medical training provides multiple benefits. It enables clinicians to critically evaluate AI-driven studies and apply AI technology to improve patient care. It also fosters innovation, allowing clinicians to optimize drug therapy, identify medication errors, and tailor treatment plans. Additionally, it ensures clinicians stay relevant and adaptable in a field where technology is a key driver of change. As clinicians play a central role in patient care and medication management, integrating AI and ML into their education enhances their professional agility, scientific rigor, and clinical acumen. This alignment between education and clinical practice positions clinicians to lead and excel in this evolving technological landscape.
Bias is inherent in any study, and guidelines are designed to identify and mitigate these biases (26). One focus is on administrative information, where the study’s title should clearly indicate AI or ML use. The study’s purpose and intended users should be defined, along with any pre-existing evidence supporting the AI/ML intervention. Other biases can be found in the study’s methods, like vague inclusion and exclusion criteria or unclear integration of AI into the trial setting. To minimize these biases, studies should specify the AI/ML algorithm version, data acquisition process, and how the AI intervention was used.
By understanding these biases, readers can better assess the robustness and reliability of AI/ML studies. This perspective allows clinicians and researchers to improve the quality of AI and ML research in healthcare, leading to more reliable and effective outcomes.
Conclusions
The integration of AI and ML into healthcare has presented a pressing need for rigorous and reliable evaluative frameworks within clinical practice. The guidelines and checklist delineated in this study respond to this complex challenge, furnishing future clinicians with an intricate tool that enables them to assess the robustness, validity, and reproducibility of AI and ML intervention studies. In a field that has been fraught with common pitfalls and challenges associated with the development of trustworthy AI systems, our comprehensive proposal for AI research guidelines offers a tangible pathway forward. These guidelines equip clinicians to unlock the full potential of AI systems in enhancing medication management and innovating patient care strategies. As AI and ML continue their transformative journey within healthcare, the tools we have created stand as a strategic convergence of technology, evidence-based practice, and clinical wisdom. They empower clinicians to interpret, critique, and apply the growing body of AI and ML literature with confidence, precision, and acumen. With health applications increasingly tailored to clinicians and patients, the future of healthcare is undeniably linked to the intelligent application of AI and ML. As AI and ML technologies continue to revolutionize healthcare, there is an urgent, unmet need to integrate these subjects into the educational curriculum for clinicians. A profound understanding of AI and ML is not just a value-added skill; it’s a prerequisite for clinicians to become leaders in the rapidly evolving landscape of healthcare. Educating clinicians in these cutting-edge technologies is not merely a matter of staying current; it’s about equipping them to be the architects of an innovative, efficient, and patient-centric future in all aspects of healthcare.
Acknowledgments
We are grateful to the Western University of Health Sciences pharmacy professional students for contributing to the research.
Funding: None.
Footnote
Data Sharing Statement: Available at https://jmai.amegroups.com/article/view/10.21037/jmai-24-65/dss
Peer Review File: Available at https://jmai.amegroups.com/article/view/10.21037/jmai-24-65/prf
Conflicts of Interest: The author has completed the ICMJE uniform disclosure form (available at https://jmai.amegroups.com/article/view/10.21037/jmai-24-65/coif). The author has no conflicts of interest to declare.
Ethical Statement: The author is accountable for all aspects of the work in ensuring that questions related to the accuracy or integrity of any part of the work are appropriately investigated and resolved. Ethical approval was not required for this study as it did not involve human participants, human data, or human tissue. This decision is in compliance with the national and institutional requirements for research involving non-human subjects. The research methods were carried out in accordance with relevant guidelines and regulations, ensuring the integrity and ethical conduct of the study.
Open Access Statement: This is an Open Access article distributed in accordance with the Creative Commons Attribution-NonCommercial-NoDerivs 4.0 International License (CC BY-NC-ND 4.0), which permits the non-commercial replication and distribution of the article with the strict proviso that no changes or edits are made and the original work is properly cited (including links to both the formal publication through the relevant DOI and the license). See: https://creativecommons.org/licenses/by-nc-nd/4.0/.
References
- Patel VL, Shortliffe EH, Stefanelli M, et al. The coming of age of artificial intelligence in medicine. Artif Intell Med 2009;46:5-17. [Crossref] [PubMed]
- Krusling J. Research Guides: Artificial Intelligence/Chat-GPT: IBM Watson 2023 [cited 2023 Aug 28]. Available online: https://ggu.libguides.com/c.php?g=1163426&p=8493172
- Karthikeyan A, Priyakumar UD. Artificial intelligence: machine learning for chemical sciences. J Chem Sci (Bangalore) 2022;134:2. [Crossref] [PubMed]
- Hamet P, Tremblay J. Artificial intelligence in medicine. Metabolism 2017;69:S36-40. [Crossref] [PubMed]
- Four ways AI can slash healthcare costs around the world | World Economic Forum 2023 [cited 2023 Aug 28]. Available online: https://www.weforum.org/agenda/2018/05/four-ways-ai-is-bringing-down-the-cost-of-healthcare/
- Roosan D, Chok J, Baskys A, et al. PGxKnow: a pharmacogenomics educational HoloLens application of augmented reality and artificial intelligence. Pharmacogenomics 2022;23:235-45. [Crossref] [PubMed]
- Cruz Rivera S, Liu X, Chan AW, et al. Guidelines for clinical trial protocols for interventions involving artificial intelligence: the SPIRIT-AI extension. Nat Med 2020;26:1351-63. [Crossref] [PubMed]
- Zafar A. 7 Best AI (Artificial Intellligence) Courses for Healthcare in 2023 MLTut. 2020 [cited 2023 Aug 28]. Available online: https://www.mltut.com/best-artificial-intelligence-courses-for-healthcare/
- Roosan D, Law AV, Roosan MR, et al. Artificial Intelligent Context-Aware Machine-Learning Tool to Detect Adverse Drug Events from Social Media Platforms. J Med Toxicol 2022;18:311-20. [Crossref] [PubMed]
- Javed R, Saba T, Humdullah S, et al. An efficient pattern recognition based method for drug-drug interaction diagnosis. In: 2021 1st International Conference on Artificial Intelligence and Data Analytics (CAIDA). IEEE; 2021:221-6.
- Hung TNK, Le NQK, Le NH, et al. An AI-based Prediction Model for Drug-drug Interactions in Osteoporosis and Paget's Diseases from SMILES. Mol Inform 2022;41:e2100264. [Crossref] [PubMed]
- Zhang Y, Deng Z, Xu X, et al. Application of Artificial Intelligence in Drug-Drug Interactions Prediction: A Review. J Chem Inf Model 2024;64:2158-73. [Crossref] [PubMed]
- Roosan D, Chok J, Karim M, et al. Artificial Intelligence-Powered Smartphone App to Facilitate Medication Adherence: Protocol for a Human Factors Design Study. JMIR Res Protoc 2020;9:e21659. [Crossref] [PubMed]
- Roosan D, Clutter J, Kendall B, et al. Power of heuristics to improve health information technology system design. ACI Open 2022;6:e114-e122. [Crossref]
- Islam R, Weir C, Del Fiol G. Clinical Complexity in Medicine: A Measurement Model of Task and Patient Complexity. Methods Inf Med 2016;55:14-22. [Crossref] [PubMed]
- Islam R, Weir CR, Jones M, et al. Understanding complex clinical reasoning in infectious diseases for improving clinical decision support design. BMC Med Inform Decis Mak 2015;15:101. [Crossref] [PubMed]
- Roosan D, Hwang A, Law AV, et al. The inclusion of health data standards in the implementation of pharmacogenomics systems: a scoping review. Pharmacogenomics 2020;21:1191-202. [Crossref] [PubMed]
- Jones C, Thornton J, Wyatt JC. Artificial intelligence and clinical decision support: clinicians' perspectives on trust, trustworthiness, and liability. Med Law Rev 2023;31:501-20. [Crossref] [PubMed]
- Khan O, Parvez M, Kumari P, et al. The future of pharmacy: How AI is revolutionizing the industry. Intell Pharm 2023;1:32-40. [Crossref]
- Schutz N, Olsen CA, McLaughlin AJ, et al. ASHP Statement on the Use of Artificial Intelligence in Pharmacy. Am J Health Syst Pharm 2020;77:2015-8. [Crossref] [PubMed]
- AMA issues new principles for AI development, deployment & use American Medical Association. 2023 [cited 2024 Apr 24]. Available online: https://www.ama-assn.org/press-center/press-releases/ama-issues-new-principles-ai-development-deployment-use
- Wu Y, Li Y, Baskys A, et al. Health disparity in digital health technology design. Health Technol 2024;14:239-49. [Crossref]
- Roosan D, Padua P, Khan R, et al. Effectiveness of ChatGPT in clinical pharmacy and the role of artificial intelligence in medication therapy management. J Am Pharm Assoc (2003) 2024;64:422-428.e8. [Crossref] [PubMed]
- Li Y, Phan H, Law AV, et al. Gamification to Improve Medication Adherence: A Mixed-method Usability Study for MedScrab. J Med Syst 2023;47:108. [Crossref] [PubMed]
- Kaul V, Enslin S, Gross SA. History of artificial intelligence in medicine. Gastrointest Endosc 2020;92:807-12. [Crossref] [PubMed]
- Ibrahim H, Liu X, Denniston AK. Reporting guidelines for artificial intelligence in healthcare research. Clin Exp Ophthalmol 2021;49:470-6. [Crossref] [PubMed]
- Sounderajah V, Ashrafian H, Golub RM, et al. Developing a reporting guideline for artificial intelligence-centred diagnostic test accuracy studies: the STARD-AI protocol. BMJ Open 2021;11:e047709. [Crossref] [PubMed]
- Collins GS, Dhiman P, Andaur Navarro CL, et al. Protocol for development of a reporting guideline (TRIPOD-AI) and risk of bias tool (PROBAST-AI) for diagnostic and prognostic prediction model studies based on artificial intelligence. BMJ Open 2021;11:e048008. [Crossref] [PubMed]
- Liu X, Cruz Rivera S, Moher D, et al. Reporting guidelines for clinical trial reports for interventions involving artificial intelligence: the CONSORT-AI extension. Nat Med 2020;26:1364-74. [Crossref] [PubMed]
- Norgeot B, Quer G, Beaulieu-Jones BK, et al. Minimum information about clinical artificial intelligence modeling: the MI-CLAIM checklist. Nat Med 2020;26:1320-4. [Crossref] [PubMed]
- Hernandez-Boussard T, Bozkurt S, Ioannidis JPA, et al. MINIMAR (MINimum Information for Medical AI Reporting): Developing reporting standards for artificial intelligence in health care. J Am Med Inform Assoc 2020;27:2011-5. [Crossref] [PubMed]
- Vasey B, Nagendran M, Campbell B, et al. Reporting guideline for the early stage clinical evaluation of decision support systems driven by artificial intelligence: DECIDE-AI. BMJ 2022;377:e070904. [Crossref] [PubMed]
- Zhang Z, Sun C, Liu ZP. Discovering biomarkers of hepatocellular carcinoma from single-cell RNA sequencing data by cooperative games on gene regulatory network. J Comput Sci 2022;65:101881. [Crossref]
Cite this article as: Roosan D. Comprehensive guide and checklist for clinicians to evaluate artificial intelligence and machine learning methodological research. J Med Artif Intell 2024;7:26.