Evaluation of range of motion using vision artificial intelligence (AI) in musculoskeletal medicine
Highlight box
Key findings
• The range of motion (ROM) of various joints can be accurately measured by vision artificial intelligence (AI) on the ViFive app.
What is known and what is new?
• New technological advances have provided potential alternative tools to measure joint ROM such as motion analysis systems, digital goniometers, and mobile device applications. Smartphone application-based ROM measurement tools have shown consistently promising results in terms of their reliability and validity.
• This study aims to evaluate the accuracy and reliability of ViFive in measuring participants’ ROM in comparison to the universal goniometer. In addition, this study examines multiple ROMs, including shoulder flexion, abduction, extension, internal rotation, external rotation, as well as hip, knee, and ankle ROMs. To our knowledge, this is the first study to date that examines the ROM of both upper and lower extremities in a single study, largely made possible by the convenience of vision AI technology.
What is the implication, and what should change now?
• This study lends credit to the exciting possibility of using a mobile device to assess a patient’s musculoskeletal conditions in lieu of a traditional physical exam. This is beneficial in challenging situations like virtual visits and when staff or resources are limited. Other potential clinical applications include gait analysis, measurement of the quadriceps angle (Q-angle), and strength assessment. The application is already being applied to other parts of the human body such as neck, back, and elbow. Furthermore, ViFive can be incorporated into web-based remote physical therapy sessions to ensure proper form and to track progression.
Introduction
The analysis and detection of human movement is significant in research related to artificial intelligence (AI) due to its many applications in rehabilitation and musculoskeletal medicine. While AI has been successfully used in recognizing patterns in various clinical contexts and specialties, including neurology (1-3), cardiovascular (4-6), and pulmonary (7-9), there have also been recent strides in musculoskeletal medicine. For instance, machine learning algorithms based on ultrasound images programmed to detect degenerative findings and intrasubstance tears in lateral elbow tendinopathy (LET) presented with high diagnosis accuracy of LET (10). Machine learning coupled with motion capture for biomechanical range of motion (ROM) assessment can also successfully estimate the physical state of the lumbar spine and help in diagnosis of patients with low back pain affecting range mobility (11).
The ROM evaluation is a significant component of the musculoskeletal examination. Assessments of mobility in musculoskeletal medicine are typically achieved by measuring the ROM of various joints, either using a manual instrument such as the goniometer, or by visual approximation by an examiner. These assessments provide important data in arriving at a clinical diagnosis, evaluation for functional impairments, monitoring treatment response, and assessing for clinical progression in rehabilitation. However, measurements obtained by subjective estimation or manual instruments often have significant room for variation and may lead to discrepancies in data due to measurement errors and different patient characteristics (12). The universal goniometer (UG) has long been considered the gold standard for measuring joint ROM due to its low cost, ease of use, and portability (13). The reliability of UG was validated in the 1980s by demonstrating a strong correlation between radiographic and goniometric measures of the knee (14). However, limitations with using the UG remain. For example, the goniometer requires an in-person evaluation and both hands to use, is time-consuming, needs clear visualization of the joint and surrounding body structures, and requires the subject to maintain still for a period of time. All of these conditions can be difficult to achieve in certain patients and contribute to measurement errors. Alternative methods, such as 3-dimentional gyroscopes (15,16), inertial sensors and magnetic sensors (17-19), and motion capture systems (20) are also limited by high costs, requirement of skilled operators, and restricted accessibility.
New technological advances have provided potential alternative tools to measure joint ROM such as motion analysis systems, digital goniometers, and mobile device applications. Recent progressions in computer vision and marker-less methods have propelled the advancement of posture-estimation algorithms, enabling precise tracking of human motion with heightened accuracy and minimal technical prerequisites (21-23). It is crucial to establish the reliability and validity inherent in these tools that allow for ROM assessment. Reliability can be defined as consistency in results using similar methods while validity is agreement in measurements or results using two different methods (24). A review of the literature revealed studies with mixed results concerning the validity and reliability of motion analysis systems. Video-based tracking systems have been reported to be a reliable and valid method to measure wrist and forearm ROM, while two other studies evaluating shoulder and lower extremity ROM showed substantial variation between the measurements obtained by a goniometer and the motion analysis (25-27). Latreche et al. further supported that a MediaPipe-based measurement system is reliable and valid for telerehabilitation for shoulder ROM (28,29). In contrast, the reliability and validity of smartphone application-based ROM measurement tools have shown consistently promising results (30-32). Jones et al. and Dos Santos et al. demonstrated that the knee joint angle measurements as measured by the Simple Goniometer® App and the ROM© applications, respectively, had no significant differences compared to those measured by the UG (30,32). Additionally, Mitchell et al. evaluated active shoulder external rotation (ER) ROM measured by novice and experienced raters using two smartphone-based applications and found that intra-rater reliability and inter-rater reliability were excellent (31). Given these promising results, we hope to validate and utilize another smartphone-based application called ViFive for ROM measurements in a clinical setting. The coronavirus disease 2019 (COVID-19) pandemic and the resulting growth in telemedicine further served as an impetus for us to utilize ViFive as a convenient tool that can be used in virtual clinic visits.
ViFive is an electronic device-based goniometric mobile application that uses a proprietary model of vision AI to produce real-time measurements of various joint ROM. In addition to minimizing human errors, the ViFive app has the additional advantages of being low-cost, widely applicable via any smartphone or tablet device and obtains rapid measurements. Of note, the validity and reliability of ViFive have not been evaluated previously. Therefore, this study aims to evaluate the accuracy and reliability of ViFive in measuring participants’ ROM in comparison to the current gold standard, the UG. In addition, this study aims to examine multiple ROMs, including shoulder flexion, abduction, extension, internal rotation (IR), ER, as well as hip, knee, and ankle ROMs. To our knowledge, this is the first study to date that examines the ROM of both upper and lower extremities in a single study, largely made possible by the convenience of vision AI technology. The results of this study will inform future studies using mobile device-based applications in musculoskeletal medicine and pave the way for more efficient and accurate assessment of joint conditions.
Methods
Subjects
Thirty-one adult participants were recruited from the community and the sports medicine outpatient clinic, as shown in Table 1. Inclusion criteria included adults above the age of 18 years. Exclusion criteria included individuals 18 years and younger, and pre-existing conditions or weight-bearing limitations that may prevent the subject from standing or squatting. Participants completed a demographics questionnaire including information on gender, age, ethnicity, race, height, and weight. The study was conducted in accordance with the Declaration of Helsinki (as revised in 2013). The study was approved by the Stanford University Institutional Review Board (IRB 6) prior to participant recruitment, and informed consent was obtained from all individual participants.
Table 1
Variable | Overall (N=31) |
---|---|
Age (years) | 30.7±12.0 |
Height (cm) | 171.1±10.7 |
Weight (kg) | 70.0±12.9 |
BMI (kg/m2) | 23.8±3.5 |
Sex | |
Female | 12 [39] |
Male | 19 [61] |
Race | |
Asian | 16 [52] |
Unknown/not reported | 1 [3] |
White | 13 [42] |
More than one race | 1 [3] |
Side evaluated (shoulder) | |
Right | 18 [58] |
Left | 8 [26] |
Both | 5 [16] |
Side evaluated (hip/knee/ankle) | |
Right | 17 [55] |
Left | 9 [29] |
Both | 2 [6] |
None | 3 [10] |
Data are reported with means ± standard deviation or frequency [%]. BMI, body mass index.
Procedures and tools
ViFive is an electronic device-based mobile application that uses a proprietary model of vision AI to measure various joint ROM in real-time using the AI stacks owned by ViFive. All parts of this study were conducted on iPad Air (4th generation) using its front camera. ViFive application completes calibration internally based on ViFive’s proprietary algorithm (patent pending).
Active ROM of the glenohumeral (shoulder), femoroacetabular (hip), tibiofemoral (knee), and talocrural (ankle) joints were measured simultaneously with the ViFive app (Figure 1A-1F) and by a trained examiner using a UG simultaneously in the same position. Shoulder ROM was measured across abduction, flexion, extension, IR, and ER with the subjects in a standing position. Active femoroacetabular, tibiofemoral, and talocrural joint ROMs were measured in hip flexion, knee flexion, and ankle dorsiflexion in a squat position. The subjects were asked to choose a side (e.g., right, left, or both) from which all ROM measurements were conducted. Participants were positioned by following the instructions on the ViFive app until their body was fully visible on the device screen and their arms or legs were in the desired position for each ROM measurement. Then, they were instructed to maintain the target posture until the measurements were completed. The ViFive app displayed the real-time ROM results on the device screen, which mirrors the movements to the subject for feedback purposes. The subject’s movements on the device’s screen were saved via screen-recording and entered into the database a later time. While the subject was in the desired position, the examiner measured the ROM manually using a goniometer. Goniometer measurements taken by the examiner were recorded into a secure database system immediately following the measurements. ViFive ROM measurement results were either verbally reported to the examiner by the subject viewing the device screen after manual measurements were obtained or later transcribed from screen recordings. Therefore, the examiners were masked to the ViFive results while measuring and recording the measurements produced by a goniometer. Each measurement was repeated three times for the first 11 subjects and twice for the remaining 20 subjects, as it was determined from preliminary data from the first 11 subjects that only two measurements were sufficient for data analysis purposes.
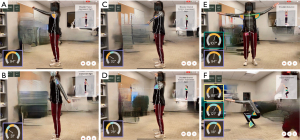
Statistical analysis
Based on a power analysis, a sample size of 34 would yield at least 90% power to detect a minimum slop of 0.5 when using a linear model to analyze the relationship between goniometer and ViFive measurements. After data collection from the ViFive app and the manual goniometer measurements, mixed-effects linear regression models were used to assess the relationship between ViFive application and goniometer measures, including participant ID as a random factor. This accounted for multiple measurements taken of each individual and addresses within-participant variability. Intraclass correlation coefficients (ICC) were also calculated to determine the level of agreement between the two modalities using ICC (2,1) values. The ICC are classified as poor when it is 0.00–0.40, fair when it is 0.40–0.59, good when it is 0.60–0.74, and excellent when it is 0.75–1.00 (33). Intra-rater reliability of goniometer and ViFive measurements were assessed using ICC (2,1) values and their 95% confident intervals. Analyses were run in RStudio version 2022.12.0+353 (Boston, MA, USA) using a two-sided level of significance of 0.05.
Results
Subjects range from age 21 to 70 years, 39% of whom were female. The ROM measurements were performed on either left, right, or both sides (Table 1).
Data obtained from 31 subjects with up to 100 separate measurements of each ROM assessment demonstrate strong association between measurements shown on the ViFive app and those measured by researchers using a goniometer. Specifically, mixed-effects linear regression model shows strong association with all shoulder, hip, knee, and ankle goniometric values (P<0.001 for all) (Figure 2, Table 2). It also shows that ViFive measurements tend to slightly overestimate goniometer values, indicated by estimates less than 1. For example, the shoulder flexion estimate number of 0.94 indicates that for every increase in ViFive values by 1 degree, its corresponding goniometer values increase by 0.94 degree.
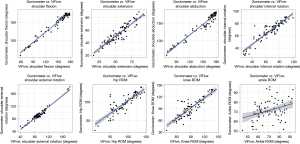
Table 2
ViFive measure | Estimate | Lower 95% CI | Upper 95% CI | P value |
---|---|---|---|---|
Shoulder flexion | 0.94 | 0.90 | 0.97 | <0.001* |
Shoulder extension | 0.78 | 0.70 | 0.86 | <0.001* |
Shoulder abduction | 0.96 | 0.92 | 1.01 | <0.001* |
Shoulder internal rotation | 0.81 | 0.71 | 0.90 | <0.001* |
Shoulder external rotation | 0.90 | 0.86 | 0.94 | <0.001* |
Hip | 0.62 | 0.51 | 0.73 | <0.001* |
Knee | 0.78 | 0.64 | 0.92 | <0.001* |
Ankle | 0.36 | 0.18 | 0.53 | <0.001* |
*, significance, alpha =0.05.
Of note, even though there is strong association between ViFive and goniometer of the ankle ROM, its estimate is the farthest away from 1. There was a software update to improve measurement accuracy for ankle ROM half-way through the study. The update changed the ankle ROM measurement from the angle formed between knee-ankle-ground originally to a measurement of the angle formed between knee-ankle-toe. However, even though there remains a strong association between measurements done with ViFive and goniometer, the software update did not significantly improve the range of motion estimates, shown by the confidence intervals overlapping (Table 3).
Table 3
ViFive measure | Estimate | Lower 95% CI | Upper 95% CI | P value |
---|---|---|---|---|
Ankle (before update) | 0.57 | 0.17 | 0.97 | 0.009 |
Ankle (after update) | 0.37 | 0.15 | 0.59 | 0.002 |
CIs for overlap, suggesting no significant improvement in the accuracy. ROM, range of motion; CI, confidence interval.
Lastly, intra-rater reliability assessed by the ICC values demonstrate that the intra-rater reliability was good to excellent for most joints, with ankle ROM having the worst reliability (Table 4).
Table 4
Variable | ViFive ICC (95% CI) | Goniometer ICC (95% CI) |
---|---|---|
Rater 1 | ||
Shoulder flexion | 0.97 (0.92–0.99) | 0.97 (0.92–0.99) |
Shoulder extension | 0.89 (0.77–0.97) | 0.88 (0.74–0.96) |
Shoulder abduction | 0.93 (0.85–0.98) | 0.96 (0.90–0.99) |
Shoulder internal rotation | 0.93 (0.85–0.98) | 0.92 (0.82–0.97) |
Shoulder external rotation | 0.77 (0.56–0.92) | 0.80 (0.61–0.93) |
Hip | 0.79 (0.59–0.93) | 0.75 (0.52–0.92) |
Knee | 0.74 (0.52–0.91) | 0.76 (0.56–0.92) |
Ankle | 0.83 (0.67–0.95) | 0.70 (0.47–0.90) |
Rater 2 | ||
Shoulder flexion | 0.68 (0.26–0.93) | 0.79 (0.53–0.96) |
Shoulder extension | 0.80 (0.16–0.96) | 0.81 (0.34–0.96) |
Shoulder abduction | 0.99 (0.96–0.99) | 0.98 (0.94–0.99) |
Shoulder internal rotation | 0.92 (0.76–0.98) | 0.95 (0.83–0.99) |
Shoulder external rotation | 0.99 (0.96–0.99) | 0.99 (0.96–0.99) |
Hip | 0.81 (0.49–0.96) | 0.81 (0.49–0.96) |
Knee | 0.81 (0.50–0.96) | 0.76 (0.40–0.95) |
Ankle | 0.60 (0.17–0.90) | 0.50 (0.04–0.87) |
Rater 3 | ||
Shoulder flexion | 0.82 (0.67–0.94) | 0.71 (0.47–0.89) |
Shoulder extension | 0.71 (0.50–0.88) | 0.54 (0.31–0.79) |
Shoulder abduction | 0.89 (0.78–0.96) | 0.93 (0.85–0.98) |
Shoulder internal rotation | 0.85 (0.71–0.95) | 0.70 (0.49–0.88) |
Shoulder external rotation | 0.86 (0.72–0.95) | 0.87 (0.74–0.95) |
Hip | 0.68 (0.46–0.87) | 0.74 (0.55–0.90) |
Knee | 0.63 (0.40–0.85) | 0.68 (0.46–0.87) |
Ankle | 0.19 (0.01–0.51) | 0.74 (0.54–0.90) |
Poor (0.00–0.39), fair (0.40–0.59), good (0.60–0.74), and excellent (0.75–1.00). ICC, intraclass correlation coefficient; CI, confidence interval.
Discussion
There are several limitations to this present study. The most significant limitation in the methods of this study is that by obtaining the goniometer measurements right after the ViFive measurements, there is a chance for the patients to change their shoulder or squat position. Goniometer measurements could also take up to 5–10 seconds longer than the instantaneous results produced on the ViFive interface. It is likely that the subjects will drop their shoulder or rise in their squat during this time due to fatigue. This possibility could have contributed to ViFive overestimating the ROM when comparing to goniometric measurements. The second limitation is that ankle ROM remains challenging for the current vision AI technology utilized by ViFive despite a software update at the time of this study. This is because the software assumes no shoe wear in the subject and does not account for ankle ER (Figure 1F). Future studies can eliminate this challenge by ensuring the subjects’ feet and the camera are perpendicular to each other and shoes are removed for future subjects. The third limitation is that the population studied is relatively small and specific (i.e., 31 participants who work at or seek care at a tertiary outpatient sports medicine clinic), which may limit the generalizability of the study results to other populations or settings. Lastly, inter-rater reliability was not evaluated in this study, as it can be logistically challenging to measure the same joint by multiple examiners using the goniometer simultaneously.
Despite its limitations, this study lends credit to the exciting possibility of using a mobile device to assess a patient’s musculoskeletal conditions in lieu of a traditional physical exam. This may especially be beneficial in challenging situations like virtual visits and when staff or resources are limited. There could be other potential clinical applications using ViFive, such as gait analysis, measurement of the quadriceps angle (Q-angle), and strength assessment. The application is already being applied to other parts of the human body such as neck, back, and elbow ROM. Furthermore, ViFive can be incorporated into web-based remote physical therapy or personal training sessions to ensure proper form and to track progression.
Conclusions
The results from this study demonstrate that the ViFive mobile application is a valid and reliable tool for measuring active shoulder, hip, knee, and ankle ROMs as compared to the current gold standard of measurement, the UG. In addition, this study demonstrated good to excellent intra-rater reliability between the different examiners.
The results of this study may inform future studies using mobile device-based applications in musculoskeletal medicine. Future research may be beneficial to reproduce the findings of this study and to aid in the development of clinical confidence of smartphone application-based ROM measurement tools.
Acknowledgments
We would like to thank all of our volunteers for making this study possible. We also would like to thank Joshua Kang for helping us with data collection.
Funding: None.
Footnote
Data Sharing Statement: Available at https://jmai.amegroups.com/article/view/10.21037/jmai-24-58/dss
Peer Review File: Available at https://jmai.amegroups.com/article/view/10.21037/jmai-24-58/prf
Conflicts of Interest: All authors have completed the ICMJE uniform disclosure form (available at https://jmai.amegroups.com/article/view/10.21037/jmai-24-58/coif). The authors have no conflicts of interest to declare.
Ethical Statement: The authors are accountable for all aspects of the work in ensuring that questions related to the accuracy or integrity of any part of the work are appropriately investigated and resolved. The study was conducted in accordance with the Declaration of Helsinki (as revised in 2013). The study was approved by the Stanford University Institutional Review Board (IRB 6) prior to participant recruitment, and informed consent was obtained from all individual participants.
Open Access Statement: This is an Open Access article distributed in accordance with the Creative Commons Attribution-NonCommercial-NoDerivs 4.0 International License (CC BY-NC-ND 4.0), which permits the non-commercial replication and distribution of the article with the strict proviso that no changes or edits are made and the original work is properly cited (including links to both the formal publication through the relevant DOI and the license). See: https://creativecommons.org/licenses/by-nc-nd/4.0/.
References
- Cascianelli S, Scialpi M, Amici S, et al. Role of Artificial Intelligence Techniques (Automatic Classifiers) in Molecular Imaging Modalities in Neurodegenerative Diseases. Curr Alzheimer Res 2017;14:198-207. [Crossref] [PubMed]
- Zhe S, Xu Z, Qi Y, et al. Sparse Bayesian Multiview Learning for Simultaneous Association Discovery and Diagnosis of Alzheimer’s Disease. Proc AAAI Conf Artif Intell 2015;29. doi:
10.1609/aaai.v29i1.9473 . - Liu X, Chen K, Wu T, et al. Use of multimodality imaging and artificial intelligence for diagnosis and prognosis of early stages of Alzheimer's disease. Transl Res 2018;194:56-67. [Crossref] [PubMed]
- Itu L, Rapaka S, Passerini T, et al. A machine-learning approach for computation of fractional flow reserve from coronary computed tomography. J Appl Physiol (1985) 2016;121:42-52. [Crossref] [PubMed]
- Kolossváry M, De Cecco CN, Feuchtner G, et al. Advanced atherosclerosis imaging by CT: Radiomics, machine learning and deep learning. J Cardiovasc Comput Tomogr 2019;13:274-80. [Crossref] [PubMed]
- Lee H, Huang C, Yune S, et al. Machine Friendly Machine Learning: Interpretation of Computed Tomography Without Image Reconstruction. Sci Rep 2019;9:15540. [Crossref] [PubMed]
- Hwang EJ, Park S, Jin KN, et al. Development and Validation of a Deep Learning-Based Automated Detection Algorithm for Major Thoracic Diseases on Chest Radiographs. JAMA Netw Open 2019;2:e191095. [Crossref] [PubMed]
- Rajpurkar P, Irvin J, Ball RL, et al. Deep learning for chest radiograph diagnosis: A retrospective comparison of the CheXNeXt algorithm to practicing radiologists. PLoS Med 2018;15:e1002686. [Crossref] [PubMed]
- Nam JG, Park S, Hwang EJ, et al. Development and Validation of Deep Learning-based Automatic Detection Algorithm for Malignant Pulmonary Nodules on Chest Radiographs. Radiology 2019;290:218-28. [Crossref] [PubMed]
- Droppelmann G, Tello M, García N, et al. Lateral elbow tendinopathy and artificial intelligence: Binary and multilabel findings detection using machine learning algorithms. Front Med (Lausanne) 2022;9:945698. [Crossref] [PubMed]
- Villalba-Meneses F, Guevara C, Lojan AB, et al. Classification of the Pathological Range of Motion in Low Back Pain Using Wearable Sensors and Machine Learning. Sensors (Basel) 2024;24:831. [Crossref] [PubMed]
- Batista L, Camargo PR, Aiello GV, et al. Knee joint range-of-motion evaluation: correlation between measurements achieved using a universal goniometer and an isokinetic dynamometer. Rev Bras Fisioter 2005;10:193-8.
- Wochatz M, Tilgner N, Mueller S, et al. Reliability and validity of the Kinect V2 for the assessment of lower extremity rehabilitation exercises. Gait Posture 2019;70:330-5. [Crossref] [PubMed]
- Gogia PP, Braatz JH, Rose SJ, et al. Reliability and validity of goniometric measurements at the knee. Phys Ther 1987;67:192-5. [Crossref] [PubMed]
- El-Zayat BF, Efe T, Heidrich A, et al. Objective assessment of shoulder mobility with a new 3D gyroscope--a validation study. BMC Musculoskelet Disord 2011;12:168. [Crossref] [PubMed]
- El-Zayat BF, Efe T, Heidrich A, et al. Objective assessment, repeatability, and agreement of shoulder ROM with a 3D gyroscope. BMC Musculoskelet Disord 2013;14:72. [Crossref] [PubMed]
- Picerno P. 25 years of lower limb joint kinematics by using inertial and magnetic sensors: A review of methodological approaches. Gait Posture 2017;51:239-46. [Crossref] [PubMed]
- Yamaura K, Mifune Y, Inui A, et al. Accuracy and reliability of tridimensional electromagnetic sensor system for elbow ROM measurement. J Orthop Surg Res 2022;17:60. [Crossref] [PubMed]
- Mukohara S, Mifune Y, Inui A, et al. A new quantitative evaluation system for distal radioulnar joint instability using a three-dimensional electromagnetic sensor. J Orthop Surg Res 2021;16:452. [Crossref] [PubMed]
- Yahya M, Shah JA, Kadir KA, et al. Motion capture sensing techniques used in human upper limb motion: a review. Sens Rev 2019;39:504-11.
- Stenum J, Cherry-Allen KM, Pyles CO, et al. Applications of Pose Estimation in Human Health and Performance across the Lifespan. Sensors (Basel) 2021;21:7315. [Crossref] [PubMed]
- Faisal AI, Majumder S, Mondal T, et al. Monitoring Methods of Human Body Joints: State-of-the-Art and Research Challenges. Sensors (Basel) 2019;19:2629. [Crossref] [PubMed]
- Toshev A, Szegedy C. DeepPose: Human Pose Estimation via Deep Neural Networks. In: 2014 IEEE Conference on Computer Vision and Pattern Recognition, 2014:1653-60. [cited 2024 May 16]. Available online: https://ieeexplore.ieee.org/document/6909610
- Hammersley M. Some Notes on the Terms ‘Validity’ and ‘Reliability’. Br Educ Res J 1987;13:73-81.
- Kassay AD, Daher B, Lalone EA. An analysis of wrist and forearm range of motion using the Dartfish motion analysis system. J Hand Ther 2021;34:604-11. [Crossref] [PubMed]
- Russo SA, Chafetz RS, Rodriguez LM, et al. Comparison of Shoulder Motion Measurements by Visual Estimate, Goniometer and Motion Capture. J Pediatr Orthop 2022;42:443-50. [Crossref] [PubMed]
- Ore V, Nasic S, Riad J. Lower extremity range of motion and alignment: A reliability and concurrent validity study of goniometric and three-dimensional motion analysis measurement. Heliyon 2020;6:e04713. [Crossref] [PubMed]
- Latreche A, Kelaiaia R, Chemori A, et al. Reliability and validity analysis of MediaPipe-based measurement system for some human rehabilitation motions. Measurement 2023;214:112826.
- Latreche A, Kelaiaia R, Chemori A. AI-based Human Tracking for Remote Rehabilitation Progress Monitoring. AIJR Abstr 2024 Feb 5 [cited 2024 May 16]. Available online: https://books.aijr.org/index.php/press/catalog/book/163/chapter/2860
- Jones A, Sealey R, Crowe M, et al. Concurrent validity and reliability of the Simple Goniometer iPhone app compared with the Universal Goniometer. Physiother Theory Pract 2014;30:512-6. [Crossref] [PubMed]
- Mitchell K, Gutierrez SB, Sutton S, et al. Reliability and validity of goniometric iPhone applications for the assessment of active shoulder external rotation. Physiother Theory Pract 2014;30:521-5. [Crossref] [PubMed]
- Dos Santos RA, Derhon V, Brandalize M, et al. Evaluation of knee range of motion: Correlation between measurements using a universal goniometer and a smartphone goniometric application. J Bodyw Mov Ther 2017;21:699-703. [Crossref] [PubMed]
- Cicchetti DV. Guidelines, criteria, and rules of thumb for evaluating normed and standardized assessment instruments in psychology. Psychol Assess 1994;6:284-90.
Cite this article as: Meng Y, Lee M, Clark HJY, Nguyen C, Kwon S, Schultz S, Pham NS, Schirmer D, Roh EY. Evaluation of range of motion using vision artificial intelligence (AI) in musculoskeletal medicine. J Med Artif Intell 2024;7:22.