Artificial intelligence and logistic regression vs. the modified Duke score in the detection of infective endocarditis: a comparison within the PRO-ENDOCARDITIS study
Highlight box
Key findings
• Neural nets and logistic regression can be used in the diagnosis of infective endocarditis (IE) and to evaluate pretest probability prior to transesophageal echocardiography (TEE).
What is known and what is new?
• The Duke criteria are the current standard in the diagnosis using a predefined classification of each variable, while heavily relying on TEE.
• We generate the hypothesis that dedicated calculatory approaches in the diagnosis of IE and especially prior to TEE can improve the current diagnostic standards.
What is the implication, and what should change now?
• A large dataset or metanalysis of an IE cohort could use logistic regression or neural networks to improve diagnostic accuracy or diminish the dependence on TEE.
Introduction
The modified Duke criteria are recommended by current European Society of Cardiology (ESC) guidelines as diagnostic algorithm in suspected infective endocarditis (IE) (1). Using the categorization in major and minor criteria, it enables an easy application in clinical routine, but may not optimally utilize the underlying information from each individual patient. Over time, the initial criteria as published in 1994 (2) were modified based on clinical examples and observed frequencies within population cohorts. Suggested changes were then tested against the current benchmark (3). In the latest update the major criterion of positive imaging was extended by cardiac computed tomography (CT) and positron emission tomography-CT (PET-CT) (1). In contrast, calculatory approaches created an optimized prediction model (e.g., PREDICT, VRISTA) (4), still heavily relying on high quality transesophageal echocardiography (TEE) (5). On the other hand, detailed statistical evaluation of multiple characteristics, especially by artificial intelligence are rising in the diagnostic of IE and other cardiovascular diseases (6).
We tested the hypothesis that (I) neuronal networks and conventional logistic regression analysis would provide improved prediction of IE as compared to the modified Duke score and (II) may potentially provide improved pre-test-probability prior to TEE. We present this article in accordance with the STARD reporting checklist (available at https://jmai.amegroups.com/article/view/10.21037/jmai-23-169/rc).
Methods
The details of this prospective single-center study have been described previously (7). Briefly, the cohort consists of 261 patients enrolled between December 2017 and May 2019 who were referred to the West German Heart and Vascular Center’s echocardiography laboratory for suspected IE. Patients with pre-existing confirmed IE or inability or unwillingness to provide informed consent were excluded. All TEE exams were performed using an Epig 7 C ultrasound system with an X7-2 or X8-2 probe (Philips Healthcare, Amsterdam, The Netherlands). Covariates and other criteria were obtained by structured anamnesis, physical examination, the patient’s medical history and laboratory as well as microbiological proofs. As the clinical endpoint, a board of independent cardiologists diagnosed IE, having access to all clinical information [e.g., laboratory and blood culture results, discharge letters and available imaging (8)]. To calculate the predictive accuracy of different approaches all Duke criteria (Table 1) were used. For the calculation of a pretest probability the results of the TEE exam were excluded, while the model consisted of all remaining variables. The study was conducted in accordance with the Declaration of Helsinki (as revised in 2013). The study was approved by the regional ethics committee of the West-German Heart and Vascular Center (No. 17-7747-BO) and informed consent was obtained from all individual participants.
Table 1
Variable | Total (n=261) | No IE (n=214) | IE (n=47) |
---|---|---|---|
Age (years) | 60.1±16.1 | 59.3±16.2 | 63.6±15.6 |
Male gender | 164 (62.8) | 133 (62.2) | 31 (66.0) |
BMI (kg/m2) | 26.7±5.8 | 26.4±5.2 | 28.3±8.2 |
Device | 27 (10.3) | 20 (9.4) | 7 (15.9) |
Prosthetic valve | 34 (13.0) | 27 (12.6) | 7 (15.9) |
Fever | 144 (55.2) | 118 (55.1) | 26 (55.3) |
Positive blood culture | 112 (42.1) | 79 (36.9) | 33 (70.2) |
Vascular phenomena | 13 (5.0) | 8 (3.7) | 5 (10.6) |
Heart defect | 9 (3.5) | 6 (2.8) | 3 (6.4) |
Drug abuse | 6 (2.3) | 4 (1.9) | 2 (4.3) |
Embolic disease | 40 (15.3) | 23 (10.8) | 17 (36.2) |
Modified Duke score | |||
Rejected | 152 (58.2) | 150 (70.0) | 2 (4.3) |
Possible | 94 (36.0) | 62 (29.0) | 32 (68.0) |
Definite | 15 (5.8) | 2 (1.0) | 13 (27.7) |
Data are presented as mean ± standard deviation or n (%). IE, infective endocarditis; BMI, body mass index.
Statistical analysis and neural network
The receiver operating characteristics (ROCs) were used to evaluate the ability of the modified Duke score, logistic regression analysis and a neural net in the diagnosis of IE.
Due to the small amount of features, no variable/feature selection was necessary. In this prospective trial, there was no missing data. Therefore, a specific treatment of missing data was not necessary. For the neural nets, we used neural nets with input layers consisting of 16 and 22 nodes for the two models respectively. Each neural net had two hidden layers, each consisting of two nodes. As activation function, we used the logistic function at hidden layers, but the identity at the output layer. To train the neural net we used resilient backpropagation with weight backtracking, where the sum of squared errors served as error function. The training process of the neural net was calibrated as follows: Random initial weights were used. The multiplication factor for the upper and lower learning rate was set to 1.2 and 0.5 respectively. The training process was stopped when the partial derivatives of the error function (i.e., the sum of squared errors) crossed the threshold of 0.01, but at most after 100,000 steps. For the logistic regression model, all variables of the modified Duke score were added into the multivariable model.
The Duke score was categorized in a combined variable of reject and possible vs. definite IE. The overall database was randomly stratified into a learning (80%) and a validation cohort (20%) (Table 2). The neural net was run 100 times, its mean receiver operating curve was depicted. Results are shown as area under the curve (AUC) and 95% confidence interval. To address the vulnerability of the imbalanced dataset we applied undersampling and the SMOTE algorithm (9). Precision, Recall and G-mean are provided as supplementary data (Tables S1-S5). All analyses were performed using RStudio (Version 1.4.1103, Boston, USA).
Table 2
Variable | Training (n=183) | Validation (n=78) |
---|---|---|
IE positive | 32 (17.5) | 15 (19.2) |
Age (years) | 61.18±16.20 | 62.0±16.07 |
Sex female | 68 (37.5) | 28 (35.9) |
TEE positive | 47 (25.7) | 26 (33.3) |
Data are presented as mean ± standard deviation or n (%). IE, infective endocarditis; TEE, transesophageal echocardiography.
Results
Overall, data from 261 patients with suspected IE were included in the prospective single center trial. Patients had a mean age of 60.1±16.1 years and 62.8% were male.
Figure 1 depicts the ROC curve for the modified Duke score, the binary logistic regression and the neural network. In the prediction of endocarditis the Duke Score achieves an AUC of 0.623 (0.56, 0.686). With a mean AUC of 0.878 (0.863, 0.893) the unbalanced neural net and the logistic regression [AUC: 0.984 (0.973, 0.996)] exceed the Duke score in the prediction of IE.
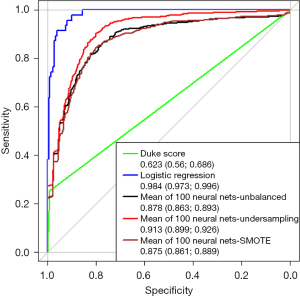
In the evaluation of pretest-probability prior to TEE the logistic regression achieved an AUC of 0.919 (0.879, 0.959) and the unbalanced neural net an AUC of 0.778 (0.757, 0.8) (Figure 2).
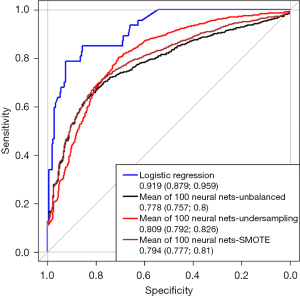
Discussion
In this observational study of 261 patients with suspected IE, we compared the established modified Duke score, logistic regression and a neural net in their predictive value quantified by the AUC. As shown in Figure 1 the logistic regression analysis and the neural network prove a higher predictive value as compared to the Duke score. As published before (10) the diagnostic accuracy of IE highly relies on the results of the TEE exam. In the evaluation of pre-test probability prior to TEE using the remaining clinical and microbiological Duke criteria, the results of neural nets and logistic regression were comparable. The small cohort of a single centered observational study is a limitation of our analysis. Although the neural net was run 100 times and cohorts were assembled randomly, the neural net needs to be tested regarding robustness in other cohorts.
Conclusions
As a hypothesis generating study, we can indicate that sophisticated statistical analyses (e.g., binary logistic regression or neural nets) can be an alternative to the graduated Duke score and that these methods can be used to increase pretest probability before performing TEE. Further studies are needed to confirm our findings and possibly generate a new algorithm for the evaluation of the risk of IE.
Acknowledgments
Funding: None.
Footnote
Reporting Checklist: The authors have completed the STARD reporting checklist. Available at https://jmai.amegroups.com/article/view/10.21037/jmai-23-169/rc
Data Sharing Statement: Available at https://jmai.amegroups.com/article/view/10.21037/jmai-23-169/dss
Peer Review File: Available at https://jmai.amegroups.com/article/view/10.21037/jmai-23-169/prf
Conflicts of Interest: All authors have completed the ICMJE uniform disclosure form (available at https://jmai.amegroups.com/article/view/10.21037/jmai-23-169/coif). T.R. received grant from German Research Foundation, speaker fees from AstraZeneca, Bayer, BMS, Pfizer, Daiichy Sankyo, CRVx, support for attending meeting from AstraZeneca, Bayer, payment from Bayer for discussion on anticoagulation strategies, and is the co-founder of Bimyo, Yes2NO, and Mycor. T.R. has the following patents: bnip3 peptides for the treatment of reperfusion injury (WO EP US CN JP KR AU CA CO CU EA IL MX SG CO2021015130A2 Tienush Rassaf Bimyo GmbH), Amelioration and treatment of infarction damage (WO WO2023079141A2 Tienush Rassaf), System for transporting and delivering of nitric oxide (WO CN KR CA CA3223532A1 Tienush Rassaf Yes2No Gmbh), Amelioration and treatment of infarction damage (US US20240058417A1 Tienush RASSAF Bimyo GmbH), Antihypertensive Composition (WO EP US US20220339228A9 Tienush RASSAF Marcus Kruse). The other authors have no conflicts of interest to declare.
Ethical Statement: The authors are accountable for all aspects of the work in ensuring that questions related to the accuracy or integrity of any part of the work are appropriately investigated and resolved. The study was conducted in accordance with the Declaration of Helsinki (as revised in 2013). The study was approved by the regional ethics committee of the West-German Heart and Vascular Center (No. 17-7747-BO) and informed consent was obtained from all individual participants.
Open Access Statement: This is an Open Access article distributed in accordance with the Creative Commons Attribution-NonCommercial-NoDerivs 4.0 International License (CC BY-NC-ND 4.0), which permits the non-commercial replication and distribution of the article with the strict proviso that no changes or edits are made and the original work is properly cited (including links to both the formal publication through the relevant DOI and the license). See: https://creativecommons.org/licenses/by-nc-nd/4.0/.
References
- Delgado V, Ajmone Marsan N, de Waha S, et al. 2023 ESC Guidelines for the management of endocarditis. Eur Heart J 2023;44:3948-4042. [Crossref] [PubMed]
- Durack DT, Lukes AS, Bright DK. New criteria for diagnosis of infective endocarditis: utilization of specific echocardiographic findings. Duke Endocarditis Service. Am J Med 1994;96:200-9. [Crossref] [PubMed]
- Li JS, Sexton DJ, Mick N, et al. Proposed modifications to the Duke criteria for the diagnosis of infective endocarditis. Clin Infect Dis 2000;30:633-8. [Crossref] [PubMed]
- Habib G, Derumeaux G, Avierinos JF, et al. Value and limitations of the Duke criteria for the diagnosis of infective endocarditis. J Am Coll Cardiol 1999;33:2023-9. [Crossref] [PubMed]
- Palraj BR, Baddour LM, Hess EP, et al. Predicting Risk of Endocarditis Using a Clinical Tool (PREDICT): Scoring System to Guide Use of Echocardiography in the Management of Staphylococcus aureus Bacteremia. Clin Infect Dis 2015;61:18-28. [Crossref] [PubMed]
- Tubiana S, Duval X, Alla F, et al. The VIRSTA score, a prediction score to estimate risk of infective endocarditis and determine priority for echocardiography in patients with Staphylococcus aureus bacteremia. J Infect 2016;72:544-53. [Crossref] [PubMed]
- Mahabadi AA, Mahmoud I, Dykun I, et al. Diagnostic value of the modified Duke criteria in suspected infective endocarditis -The PRO-ENDOCARDITIS study. Int J Infect Dis 2021;104:556-61. [Crossref] [PubMed]
- Ris T, Teixeira-Carvalho A, Coelho RMP, et al. Inflammatory biomarkers in infective endocarditis: machine learning to predict mortality. Clin Exp Immunol 2019;196:374-82. [Crossref] [PubMed]
- Chawla NV, Bowyer KW, Hall LO, et al. SMOTE: Synthetic Minority Over-sampling Technique. Journal Of Artificial Intelligence Research 2002;16:321-57. [Crossref]
- Krittanawong C, Virk HUH, Bangalore S, et al. Machine learning prediction in cardiovascular diseases: a meta-analysis. Sci Rep 2020;10:16057. [Crossref] [PubMed]
Cite this article as: Vogel LH, Kampf J, Dykun I, Mahmoud I, Rath PM, Buer J, Rassaf T, Mahabadi AA. Artificial intelligence and logistic regression vs. the modified Duke score in the detection of infective endocarditis: a comparison within the PRO-ENDOCARDITIS study. J Med Artif Intell 2024;7:24.