Decoding the heart: how artificial intelligence is transforming cardiology
A primer to artificial intelligence (AI) in cardiology: why do we need it?
While cardiovascular disease remains a leading cause of death, claiming millions of lives annually (1), cutting-edge technologies such as AI promise transformative solutions. Up to now, diagnostics in cardiology relied on human interpretations of various data, including imaging records, electrocardiograms (ECG) and health record reports. However, the advent of AI reveals certain limitations in this approach, prompting exploration of novel solutions aimed at enhancing data integration, improving diagnostic accuracy and leveraging big data to uncover new associations with clinical phenotypes.
AI excels at analyzing complex data and identifying intricate patterns that may elude human detection. What sets AI apart from previous computerized solutions is its ability to learn from examples rather than relying on diagnostic rules. AI is not rule-based and does not require any predefined set of instructions to identify, for example, conduction abnormalities in ECG strips. On the contrary, it “learns by example” (2). It suffices to “train” an AI-based system by providing it with lots of annotated input examples, for instance, ECG signals with and without a bundle branch block, and let it find complex, non-linear patterns in the signals that distinguish pathological records from normal, finally leading to the diagnosis. Then, when the model is tested on ECG data with hidden diagnostic labels, it will be able to recognize the patterns it learned and achieve a highly accurate diagnosis (3).
This example conveys an intuitive idea of how the “vanilla” version of an AI model functions. However, the actual mechanics are much more complicated, yielding numerous challenges in the development of such systems, but also offering an impressive armamentarium of capable solutions to a remarkable variety of tasks, spanning from automating imaging-based diagnoses to creating sophisticated risk stratification systems. While diagnostics is the main application field, AI extends to therapeutics, encompassing drug development and interventional procedures, with impressive performance and precision (4-7). This editorial explores how AI can revolutionize cardiology by overcoming limitations in traditional approaches, focusing on its significant strides in areas previously deemed challenging by human experts. We provide a comprehensive overview of the current and future landscape of AI tools in cardiology, along with challenges that emerge (Figure 1).
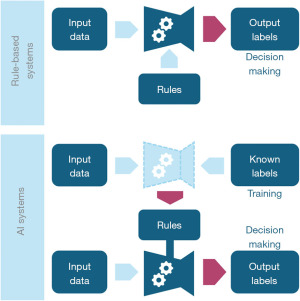
AI “under the hood”
AI is a broad field encompassing various techniques that enable machines to simulate intelligent behavior. Machine learning (ML), a more traditional and older subfield of AI, allows computers to learn from data without explicit programming. Typical ML algorithms range from classical linear regression to support vector machines (SVMs) and k-nearest neighbors (kNN), which can combine features from input data in complex ways to make predictions or classifications (8,9). However, these techniques may struggle with intricate patterns in high-dimensional data.
Deep learning (DL), another subfield of AI, takes inspiration from the structure of the human brain. It utilizes artificial neural networks (NNs), which are interconnected layers of processing units called neurons. Each neuron performs a simple mathematical operation, and by combining many such neurons, NNs can learn complex relationships within data and link input signals to their corresponding output labels with high precision (2). DL excels at tasks involving raw data like signals, images and text, where it can capture subtle differences that might elude traditional methods. Popular DL architectures and subfields include:
- Convolutional neural networks (CNNs) for image recognition, particularly adept at analyzing medical scans to detect abnormalities (10).
- Recurrent neural networks (RNNs) for sequential data, useful for tasks like analyzing heart rhythm patterns in ECG signals (11).
- Natural language processing (NLP) enables computers to understand and process human language. In healthcare, NLP can be employed to analyze medical records or patient-doctor conversations (12). Large language models (LLMs) like ChatGPT by OpenAI and Gemini by Google represent advances in NLP, trained on massive text data to generate human-like responses. While not yet mainstream in cardiology, these models hold promise for tasks such as summarizing research or generating healthcare reports.
The recent advancements in AI are primarily fueled by one vital element: data. DL algorithms, in particular, require vast amounts of labeled data for learning. The surge in generating multi-source data, including medical records and wearable sensor data, is the key driver behind the explosive growth of AI. This exponential increase in multi-modal data, coupled with the development of more capable computers, propels the development of such methods, leading to their application also in cardiology.
AI overcoming human limitations in cardiology
Relying on expert knowledge for interpreting diagnostic tests like ECGs and echocardiograms has been valuable over the years and until recently, it has been the common approach to medical data. However, today, it is evident that analyzing the enormous amounts of continuously produced data, including imaging scans, biosignals, electronic health records (EHR), wearable sensor data and genetic information, can be overwhelming for human interpretation. This can introduce bias into our judgment, as the sheer volume of data surpasses human cognitive capabilities to assimilate in a reasonable time frame. Additionally, the complexity within data is challenging to capture by conventional, mostly linear models typically used in research efforts. Finally, subtle patterns indicative of early disease stages can be easily missed by novice clinicians, while some novel diagnostic tasks are also intractable even by experts.
State-of-the-art algorithms can process massive datasets, extracting hidden insights and identifying complex relationships between various data points. This leads to accurate and automated diagnostics, allowing for earlier intervention and potentially improved patient outcomes. For example, analyzing ECGs relies on established criteria indicative of arrhythmias, which young or untrained caregivers might miss if the changes are subtle or not typical. AI algorithms can analyze vast amounts of ECG data with superior sensitivity, identifying subtle or atypical patterns of arrhythmias with higher accuracy, in the blink of an eye. Moving to more advanced tasks, efforts are made to predict the onset of future arrhythmias before their onset (13), or uncover early signs of silent lesions, such as paroxysmal atrial fibrillation (PAF) while still in sinus rhythm (14), allowing for earlier intervention and potentially life-saving treatment.
Another limitation of traditional diagnostic methods is the focus on individual tests. AI excels at integrating and combining data from various sources, including biosignals, imaging modalities, and genetic data, allowing for a more holistic picture of a patient’s cardiovascular profile. By analyzing the heterogeneous data points in conjunction, AI can identify complex relationships that might escape human observation. This integrated approach holds great promise for personalized medicine (15), allowing for tailored treatment plans based on each patient’s unique risk factors and profile.
Decoding the heart: AI in action
Curious about the specific domains where AI has started to thrive? The following subfields offer a comprehensive overview (Figure 2).
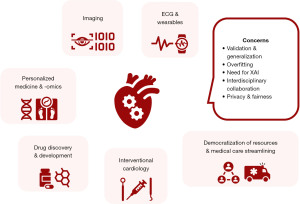
ECG and wearables
AI algorithms can analyze vast amounts of ECG data, identifying subtle patterns indicative of arrhythmias. This allows for early detection and intervention, potentially improving patient outcomes. For instance, AI can detect atrial fibrillation, a common arrhythmia that significantly increases the risk of stroke, with higher accuracy than traditional methods. Furthermore, evidence suggests that AI can predict the onset of imminent paroxysms, indicating its potential to achieve tasks that exceed the capabilities of human expertise (13). Moreover, AI can analyze ECG signals to uncover even “hidden” patterns suggestive of silent arrhythmia risk (e.g., risk for malignant tachycardias or PAF) (14). Interestingly, AI-enabled systems have recently been used not only to detect “hard-to-spot” arrhythmias but also to diagnose lesions not traditionally visible on ECG, such as moderate to severe aortic valve stenosis, misjudging fewer than one in four cases (16). Finally, by applying AI algorithms to wearable sensor data, wearables can continuously monitor and assess physiological parameters, providing a more comprehensive picture of a patient’s health compared to single snapshots of traditional diagnostic tests.
Imaging
AI can analyze non-traditional imaging modalities like cardiac magnetic resonance (CMR) and computed tomography (CT) scans to detect subtle changes indicative of early-stage heart disease or complications, while these changes may not be readily apparent through traditional visual assessment by human experts. For example, efforts focus on developing DL models that can diagnose cardiac amyloidosis with high precision from CMR or even echocardiographic images (10,17). In its most typical form, AI has been employed to simulate expert cognition in accurately identifying existing structural abnormalities, or automate and improve conventional tasks, such as estimating the ejection fraction (EF) and global longitudinal strain (GLS) from echocardiography images (18). One of the first blinded randomized controlled trials (RCTs) in this field recently demonstrated the utility of AI in cardiac echocardiography. The assessment of the left ventricle EF by the AI-enabled echocardiography system was found non-inferior to that of human sonographers, while the whole AI-powered workflow saved time (19).
Personalized medicine and -omics
Such methods can also integrate -omics data (genomics, proteomics, metabolomics), along with EHRs and other information, to create personalized risk profiles for cardiovascular diseases and “decode” the possibility of responding to certain therapies. This allows for early and personalized intervention and prevention strategies, while lowering the risk for adverse effects, thus paving the way for “precision” medicine in cardiology (15). Applying such strategies large-scale can contribute to identifying populations at high cardiovascular risk and uncover genetic, demographic and social determinants of health, allowing for targeted prevention and resource allocation. However, despite the revolutionary promises of such systems to “ingest” multi-modal data and provide highly precise, patient-tailored recommendations for a variety of conditions, their integration into clinical routine remains a goal to be achieved.
Drug discovery and development
AI accelerates the drug discovery and development pipeline by utilizing AI in several key steps, ranging from target identification to clinical testing design (4). AI algorithms analyze vast datasets of biological information to identify novel pathway targets for specific diseases and also predict the potential efficacy and safety of drug candidates. This significantly reduces the time and cost associated with traditional methods. Sophisticated AI-powered software can also analyze patient data to identify individuals most likely to benefit from new cardiovascular drugs, leading to more targeted clinical trials and faster development timelines (5). With the use of such tools in drug development, aside from process acceleration, the future holds immense promise for the creation of safer, more effective and personalized cardiovascular medications.
Interventional cardiology
AI applications extend also to interventional procedures, offering assistance across multiple levels, from guiding operators to providing robotic support. Tools have been developed to analyze a variety of data, from coronary angiographic images to intravascular modalities like optical coherence tomography (OCT) and intravascular ultrasound (IVUS), in order to guide stent sizing and deployment, and aid decision-making during percutaneous coronary interventions (PCI) (6,20). AI-assisted multi-modality imaging is also applied to electrophysiology, leveraging multiple modalities at once, from cardiac CT to specialized electroanatomical mapping, to derive a comprehensive, both anatomical and functional view of the heart, guiding further ablation strategies (21). Other applications, such as prediction of cardiac resynchronization therapy (CRT) response and implantable devices enhanced with AI algorithms, are also a reality (22), while robotic-assisted interventions that can at least semi-automate procedural steps, are emerging (7).
LLM-enabled workflow transformation
The recent evolution of DL models into LLMs, such as ChatGPT and Gemini, has expanded their potential applications in various fields. These advanced systems can generally process and generate human-like text, making them invaluable in numerous contexts. In cardiology, LLMs can support many “back-end” clinical activities, transforming how healthcare is delivered. For instance, powerful LLM models can assist in generating and summarizing clinical notes (23). This automation can save time and improve the efficiency and accuracy of documentation. Additionally, LLMs can facilitate patient-clinician communication by providing more understandable explanations of medical conditions and treatments (24). Routine tasks such as managing patient records can also be automated, enhancing the overall efficiency and quality of healthcare delivery in cardiology.
Expanding the landscape of AI in cardiology: opportunities and challenges
While AI can analyze complex data and provide insightful findings, it is crucial to recognize the valuable clinical judgment of cardiologists and the irreplaceable empathy they bring to the patient-physician relationship. AI is a powerful tool, yet still unable to replace human expertise, bearing limitations and presenting future challenges. However, by actively integrating AI tools into clinical workflow, the cardiology community can achieve superior outcomes. AI can provide real-time data analysis and risk stratification, aiding informed decision-making with treatments tailored to each patient’s unique needs. Moreover, seamless integration of AI tools, with user-friendly interfaces and robust support, can streamline patient care. Additionally, the capability of AI systems to process and utilize multi-modal data unlocks new possibilities in pre-symptomatic and personalized diagnosis of cardiac disease. For instance, integrating AI into sports cardiology enhances risk assessment by integrating and analyzing complex data from imaging, genetic testing and wearable devices (25). Finally, AI-enhanced approaches can contribute to the democratization of medical resources. For instance, integrating automated diagnostic systems in underserved areas, such as ECG modules capable of recognizing myocardial infarction, can aid unspecialized medical professionals during emergent conditions, facilitating increased alertness and timely patient management. Ensuring AI tools are accessible to clinical environments with limited resources can horizontally expand and improve medical care through low-cost viable solutions.
However, challenges persist. The medicolegal landscape for AI is still uncertain, posing potential risks for practitioners and patients alike, in certain circumstances (26). AI algorithms can be susceptible to overfitting, performing well on the data they are trained on but struggling to generalize well to other populations (27). Addressing this requires diverse and inclusive datasets during development, alongside external validation to ensure generalizability across patient demographics. Additionally, prospective studies are needed to ensure the safety and effectiveness of AI tools, particularly toward high-risk patients for whom accurate predicting and decision-making can be of paramount. Creative study designs, such as those centered around implementation science, are crucial to studying AI within clinical workflows and enhance their actual applicability (28). Furthermore, AI model decisions are often opaque since most AI architectures, and particularly DL ones, do not inherently provide explanations for their outputs. Understandably, there is an oppressing need for interpretable and explainable AI (XAI) models to build trust among cardiologists (29). By understanding how AI arrives at its conclusions, physicians can leverage its insights while maintaining control over clinical decision-making. XAI can further offer new insights into previously unknown features within the data (imaging scans, biosignals) that are associated with patient outcomes, shedding light on new research directions (30). Furthermore, recognizing the interdisciplinary nature of this field is crucial for future advancements. Fostering collaboration between cardiologists and engineers through dedicated teams or joint research programs can leverage the unique strengths and knowledge base of each field, leading to innovative solutions that bridge the gap between medical needs and cutting-edge technological advancements.
Finally, ethical considerations are paramount as AI integrates further into cardiology. Strict regulations ensure patient data privacy and security, with strong requirements for de-identification and anonymization of sensitive patient data (31). Moreover, data biases can lead to unfair outcomes for certain patient populations. Careful selection and curation of diverse training data sets is essential to mitigate bias and ensure the fair and equitable application of AI in cardiology. Nevertheless, physicians need to understand how AI algorithms arrive at their conclusions to build trust and ensure responsible integration into clinical practice. From this perspective, efforts to develop more transparent and XAI models are vital. Robust regulatory frameworks and close collaboration between healthcare professionals, technologists and policymakers are essential to address these ethical considerations.
Concluding remarks
This review provides a comprehensive and up-to-date overview of AI applications in cardiology, offering a holistic perspective that encompasses both diagnostic and therapeutic aspects. Complementing previous efforts, this work emphasizes the integration of AI across various modalities, including ECG signals, imaging, wearables, and personalized medicine. It also highlights the latest advancements in emerging fields such as personalized medicine and LLMs, while offering a critical analysis of the challenges and opportunities ahead.
Evidence suggests that AI is revolutionizing cardiology, offering transformative solutions to overcome limitations in traditional approaches. We are witnessing a paradigm shift, from reactive care to proactive, preventative and personalized strategies. AI empowers cardiologists to even achieve tasks unimaginable in the past, improving patient outcomes by identifying “hidden” patterns of future or concomitant yet silent diseases. As research progresses and ethical considerations are addressed, AI holds immense promise for the future of cardiology, paving the way for more accurate diagnoses and personalized treatment, and also guiding further research through the discovery of novel disease features. Collaborative efforts between cardiologists, AI experts, and policymakers will unlock the full potential of this transformative technology.
Acknowledgments
Funding: None.
Footnote
Provenance and Peer Review: This article was a standard submission to the journal. The article has undergone external peer review.
Peer Review File: Available at https://jmai.amegroups.com/article/view/10.21037/jmai-24-139/prf
Conflicts of Interest: All authors have completed the ICMJE uniform disclosure form (available at https://jmai.amegroups.com/article/view/10.21037/jmai-24-139/coif). The authors have no conflicts of interest to declare.
Ethical Statement: The authors are accountable for all aspects of the work in ensuring that questions related to the accuracy or integrity of any part of the work are appropriately investigated and resolved.
Open Access Statement: This is an Open Access article distributed in accordance with the Creative Commons Attribution-NonCommercial-NoDerivs 4.0 International License (CC BY-NC-ND 4.0), which permits the non-commercial replication and distribution of the article with the strict proviso that no changes or edits are made and the original work is properly cited (including links to both the formal publication through the relevant DOI and the license). See: https://creativecommons.org/licenses/by-nc-nd/4.0/.
References
- Timmis A, Vardas P, Townsend N, et al. European Society of Cardiology: cardiovascular disease statistics 2021. Eur Heart J 2022;43:716-99. [Crossref] [PubMed]
- Deitel P, Deitel H. Chapter 15: Deep Learning. In: Python for programmers: with introductory AI case studies. Pearson; 2019.
- Yang T, Gregg RE, Babaeizadeh S. Detection of strict left bundle branch block by neural network and a method to test detection consistency. Physiol Meas 2020;41:025005. [Crossref] [PubMed]
- Pantelidis P, Spartalis M, Zakynthinos G, et al. Artificial Intelligence: The New "Fuel" to Accelerate Pharmaceutical Development. Curr Pharm Des 2022;28:2127-8. [Crossref] [PubMed]
- Chopra H. Revolutionizing clinical trials: the role of AI in accelerating medical breakthroughs. Int J Surg 2023;109:4211-20. [Crossref] [PubMed]
- Ploscaru V, Popa-Fotea NM, Calmac L, et al. Artificial intelligence and cloud based platform for fully automated PCI guidance from coronary angiography-study protocol. PLoS One 2022;17:e0274296. [Crossref] [PubMed]
- Thirumurugan E, Gomathi K, Swathy P, et al. Robotic percutaneous coronary intervention (R-PCI): Time to focus on the pros and cons. Indian Heart J 2023;75:161-8. [Crossref] [PubMed]
- Deitel PJ, Deitel HM. Chapter 14: Machine Learning: Classification, Regression and Clustering. In: Python for programmers: with introductory AI case studies. Pearson; 2019.
- Murthy HSN, Meenakshi M. ANN, SVM and KNN Classifiers for Prognosis of Cardiac Ischemia- A Comparison. Bonfring Int J Res Commun Eng 2015;5:7-11. [Crossref]
- Agibetov A, Kammerlander A, Duca F, et al. Convolutional Neural Networks for Fully Automated Diagnosis of Cardiac Amyloidosis by Cardiac Magnetic Resonance Imaging. J Pers Med 2021;11:1268. [Crossref] [PubMed]
- Singh S, Pandey SK, Pawar U, et al. Classification of ECG Arrhythmia using Recurrent Neural Networks. Procedia Comput Sci 2018;132:1290-7. [Crossref]
- Wang Y, Luo J, Hao S, et al. NLP based congestive heart failure case finding: A prospective analysis on statewide electronic medical records. Int J Med Inform 2015;84:1039-47. [Crossref] [PubMed]
- Surucu M, Isler Y, Perc M, et al. Convolutional neural networks predict the onset of paroxysmal atrial fibrillation: Theory and applications. Chaos 2021;31:113119. [Crossref] [PubMed]
- Noseworthy PA, Attia ZI, Behnken EM, et al. Artificial intelligence-guided screening for atrial fibrillation using electrocardiogram during sinus rhythm: a prospective non-randomised interventional trial. Lancet 2022;400:1206-12. [Crossref] [PubMed]
- Krittanawong C, Zhang H, Wang Z, et al. Artificial Intelligence in Precision Cardiovascular Medicine. J Am Coll Cardiol 2017;69:2657-64. [Crossref] [PubMed]
- Cohen-Shelly M, Attia ZI, Friedman PA, et al. Electrocardiogram screening for aortic valve stenosis using artificial intelligence. Eur Heart J 2021;42:2885-96. [Crossref] [PubMed]
- Goto S, Mahara K, Beussink-Nelson L, et al. Artificial intelligence-enabled fully automated detection of cardiac amyloidosis using electrocardiograms and echocardiograms. Nat Commun 2021;12:2726. [Crossref] [PubMed]
- O'Driscoll JM, Tuttolomondo D, Gaibazzi N. Artificial intelligence calculated global longitudinal strain and left ventricular ejection fraction predicts cardiac events and all-cause mortality in patients with chest pain. Echocardiography 2023;40:1356-64. [Crossref] [PubMed]
- He B, Kwan AC, Cho JH, et al. Blinded, randomized trial of sonographer versus AI cardiac function assessment. Nature 2023;616:520-4. [Crossref] [PubMed]
- Chu M, Jia H, Gutiérrez-Chico JL, et al. Artificial intelligence and optical coherence tomography for the automatic characterisation of human atherosclerotic plaques. EuroIntervention 2021;17:41-50. [Crossref] [PubMed]
- Schwartz AL, Chorin E, Mann T, et al. Reconstruction of the left atrium for atrial fibrillation ablation using the machine learning CARTO 3 m-FAM software. J Interv Card Electrophysiol 2022;64:39-47. [Crossref] [PubMed]
- Brown G, Conway S, Ahmad M, et al. Role of artificial intelligence in defibrillators: a narrative review. Open Heart 2022;9:e001976. [Crossref] [PubMed]
- Zaretsky J, Kim JM, Baskharoun S, et al. Generative Artificial Intelligence to Transform Inpatient Discharge Summaries to Patient-Friendly Language and Format. JAMA Netw Open 2024;7:e240357. [Crossref] [PubMed]
- Sarraju A, Bruemmer D, Van Iterson E, et al. Appropriateness of Cardiovascular Disease Prevention Recommendations Obtained From a Popular Online Chat-Based Artificial Intelligence Model. JAMA 2023;329:842-4. [Crossref] [PubMed]
- Palermi S, Vecchiato M, Saglietto A, et al. Unlocking the potential of artificial intelligence in sports cardiology: does it have a role in evaluating athlete's heart? Eur J Prev Cardiol 2024;31:470-82. [Crossref] [PubMed]
- Mello MM, Guha N. ChatGPT and Physicians' Malpractice Risk. JAMA Health Forum 2023;4:e231938. [Crossref] [PubMed]
- Mutasa S, Sun S, Ha R. Understanding artificial intelligence based radiology studies: What is overfitting? Clin Imaging 2020;65:96-9. [Crossref] [PubMed]
- Nilsen P, Svedberg P, Neher M, et al. A Framework to Guide Implementation of AI in Health Care: Protocol for a Cocreation Research Project. JMIR Res Protoc 2023;12:e50216. [Crossref] [PubMed]
- Bienefeld N, Boss JM, Lüthy R, et al. Solving the explainable AI conundrum by bridging clinicians' needs and developers' goals. NPJ Digit Med 2023;6:94. [Crossref] [PubMed]
- Salih A, Boscolo Galazzo I, Gkontra P, et al. Explainable Artificial Intelligence and Cardiac Imaging: Toward More Interpretable Models. Circ Cardiovasc Imaging 2023;16:e014519. [Crossref] [PubMed]
- Regulation (EU) 2016/679 of the European Parliament and of the Council of 27 April 2016 on the protection of natural persons with regard to the processing of personal data and on the free movement of such data, and repealing Directive 95/46/EC (General Data Protection Regulation) (Text with EEA relevance). 2016.
Cite this article as: Pantelidis P, Oikonomou E, Gialamas I, Goliopoulou A, Sarantos S, Zakynthinos GE, Anastasiou A, Gounaridi MI, Kalogeras K, Siasos G. Decoding the heart: how artificial intelligence is transforming cardiology. J Med Artif Intell 2025;8:9.