Evaluating the predictive power of machine learning in cirrhosis mortality: a systematic review
Highlight box
Key findings
• Machine learning (ML) models consistently outperform traditional prognostic scores like model for end-stage liver disease (MELD) and Child-Pugh in predicting mortality among end-stage liver disease (ESLD) patients.
• Reviewed studies show ML models achieving area under the receiver operating characteristic curve values ranging from 0.71 to 0.96, indicating substantial improvement in predictive accuracy.
What is known and what is new?
• Traditional models, including MELD and Child-Pugh, are widely used but have inherent limitations in predictive accuracy.
• This systematic review demonstrates that ML models, by leveraging a broader array of clinical and demographic parameters, offer significantly improved mortality prediction.
What is the implication, and what should change now?
• Adoption of ML models in clinical practice could enhance the accuracy of mortality predictions for ESLD patients.
• Future research should focus on validating these models, ensuring transparency in their development, and enhancing interpretability to facilitate their integration into clinical settings.
Introduction
Cirrhosis continues to be an important cause of morbidity and mortality. Major etiologies of liver cirrhosis include metabolic dysfunction-associated steatotic liver disease (MASLD), alcohol-associated liver disease, viral hepatitis, or cholestatic liver diseases (1). The natural history of cirrhosis is well known, with a compensated stage followed by decompensation, complications of portal hypertension, and a high short-term mortality. However, this course can vary according to patient and disease-related factors (2). This variability reinforces the need to be able to predict the survival of patients with cirrhosis to optimize the use of limited therapeutic resources, such as liver transplantation (3).
Numerous prognostic models have been developed for chronic liver disease problems (4-6). In current end-stage liver disease (ESLD) research, only some of the prognostic scoring systems are accessible for early survival evaluation in noncancer-related patients. The most commonly used models are the Child-Pugh score (4), the MELD, and the model for end-stage liver disease-sodium (MELD-Na) (5,6). Even though these above models are widely used, however, each option has drawbacks due to poor detection and difficult evaluation (7).
The use of artificial intelligence (AI), especially machine learning (ML) methods, creates a chance to uncover patterns and correlations through data mining, turning them into data-driven prediction models (8). Previous studies have revealed that ML models are being successfully utilized for the prognostication of liver transplantation (9,10) and to predict adverse events including mortality in ESLD patients (11,12). For instance, in a recent study by Yu et al., the ML model based on random forest (RF) and adaptive boosting (AdaBoost) algorithm performed better than the existing scoring systems for predicting 30-day mortality in patients with non-cholestatic cirrhosis [area under the receiver operating characteristic curve (AUROC) of the RF and AdaBoost was 0.838 and 0.792 respectively] (12). However, there has been no systematic review critically evaluating and comparing all these ML models to predict the mortality of ESLD patients.
This systematic review aims to identify and evaluate the performance of ML models specifically designed to predict mortality in ESLD patients, offering a focused analysis of non-traditional predictive methodologies. We present this article in accordance with the PRISMA and CHARMS (13) reporting checklists (available at https://jmai.amegroups.com/article/view/10.21037/jmai-24-205/rc).
Methods
Our review was registered to PROSPERO (CRD42024558517) (14,15).
Literature search
In April 2024, a comprehensive search was carried out in several databases (PubMed, CINAHL, Ovid, and Cochrane) from the inception to the present day. The search strategy was designed and conducted by an experienced medical librarian with input from the study investigators. A controlled vocabulary, enhanced with relevant keywords, was employed to search for studies related to ML, mortality, and cirrhosis/ESLD. The complete search strategy is provided in Appendix 1. Additionally, the reference lists of the included studies were reviewed to identify duplicates, assess the study populations, and determine the types of ML models utilized.
Eligibility criteria
Original articles published in peer-reviewed journals that employed ML approaches and developed or validated tools, scores, or algorithms for predicting mortality in patients with ESLD/cirrhosis were included. The studies were excluded that used image-based input variables to predict ESLD mortality or the studies predicting mortality in acute liver failure. Studies that were not full-text publications or did not utilize ML techniques were excluded.
Selection process
S.M. and L.J.F. screened the titles and abstracts of the publications identified through the search strategy independently. Full texts of potentially relevant articles were retrieved. The same authors subsequently assessed the full-text publications and applied the eligibility criteria. Any disagreements were resolved through discussion, and if necessary, K.Q. was consulted to arbitrate and resolve any remaining discrepancies.
Data collection process
Following the checklist for Critical Appraisal and Data Extraction for Systematic Reviews of Prediction Modelling Studies (the CHARMS checklist), S.M. and L.J.F. independently performed data extraction. We collected information on study characteristics such as study type (prospective or retrospective), authors, journal, year of publication, population setting (inpatient, outpatient, or both), country, data source (e.g., electronic health records), sample size, and follow-up timeframe. Additionally, we abstracted and summarized details about the ML models used, including the type of model, number of models per outcome, training sample size, type and number of features, data preprocessing methods, class imbalance management strategies, training optimization criteria, reported performance metrics, and choice of test set. To assess model performance, we summarized the point estimates of the AUROC and sensitivity, specificity, and accuracy for each study. If available, we reported performance metrics from a held-out test set, and otherwise from cross-validation or bootstrapping.
Risk of bias assessment
Two reviewers independently assessed the risk of bias for each study using the guidelines for developing and reporting ML predictive models in biomedical research, as well as the recommendations for reporting ML analysis in clinical research (16,17). The evaluation criteria encompassed nine potential sources of bias, including (I) study design, population, and setting. (II) Sample size and proportion of cases. (III) Assessment of outcomes (approach for identifying outcomes and defining them). (IV) Availability of features at the time of prediction (reported timing of feature collection or assessment). (V) Data preprocessing (encoding features and managing missing data). (VI) Management of class imbalance (addressing the significant unequal distribution of classes in the training set, such as through data resampling, bagging, or using asymmetric misclassification cost). (VII) Model development (including feature selection and training optimization). (VIII) Prevention of data leakage. (IX) Code availability.
Statistical analyses
The results were summarized using descriptive statistics. We provided the frequency and proportion of each item pertaining to study characteristics, ML choices, and the assessment of reporting quality. Given the inherent heterogeneities in study designs, populations, ML approaches, and prediction targets, a meta-analysis was not performed in this systematic review.
Results
Study selection
Figure 1 depicts the PRISMA flowchart illustrating the study identification and selection process. Our search strategy yielded 360 unique citations and 57 after full-text screening. Ultimately, 10 studies (12,18-26) were included in the review. The agreement between the S.M. and L.J.F. for study inclusion was nearly perfect (Cohen’s k: 0.96, 95% confidence interval: 0.80–1.00).
Characteristics of studies
We included 10 retrospective studies. Of these, 2 were conducted in the United States and 4 in Taiwan. The remaining four studies were conducted in China, Italy, India, and Germany. Half of the studies (5/10) were single-centered. Our review included studies spanning various mortality predictions in liver disease, with two studies on 30-day mortality, four on 3-month mortality, and four also on 1-year mortality, targeting liver cirrhosis patients and those awaiting liver transplantation. The characteristics of the included studies are summarized in Table 1.
Table 1
Study | Year | Study type | Location | Centers | Etiology of liver disease |
---|---|---|---|---|---|
Cucchetti et al. (19) | 2007 | Retrospective cohort | Italy, UK | Multi | Cirrhosis of viral origin, alcohol-related chronic liver disease, cholestatic liver disease |
Gibb et al. (20) | 2023 | Retrospective cohort | Leipzig | Single | Alcohol, hepatitis B/C, autoimmune hepatitis, primary sclerosing cirrhosis, primary biliary cirrhosis, unknown causes (NASH, cryptogenic hepatitis) |
Guo et al. (21) | 2021 | Retrospective cohort | Taiwan | Single | Cardiac/congestive cirrhosis, unspecified cirrhosis, viral hepatitis C, alcoholic cirrhosis, other cirrhosis types, esophageal/gastric varices, hepatorenal syndrome, spontaneous bacterial peritonitis, primary biliary cirrhosis, pigmentary cirrhosis, Wilson’s disease |
Hu et al. (22) | 2021 | Prospective | USA | Multi | Alcohol-related, nonalcoholic fatty liver, hepatitis C, others |
Kanwal et al. (23) | 2020 | Retrospective cohort | USA | Multi | Not available |
Lin et al. (24) | 2020 | Retrospective cohort | Taiwan | Multi | Chronic liver diseases with/without complications |
Yu et al. (12) | 2021 | Retrospective cohort | Taiwan | Single | Noncancer-related chronic liver disease |
Simsek et al. (25) | 2021 | Retrospective cohort | Taiwan | Single | Cryptogenic, alcoholic liver disease, chronic hepatitis B/C, vascular disease, NASH |
Yu et al. (26) | 2022 | Prospective | China | Single | Non-cholestatic cirrhosis |
Banerjee et al. (18) | 2003 | Retrospective cohort | India | Multi | Cirrhosis |
NASH, nonalcoholic steatohepatitis.
The sample sizes used for training the models exhibited considerable variation, ranging from 124 to 107,939, with a median of 793. Among the studies cited six (12,18-20,25,26) utilized datasets with fewer than 1,000 samples, with a mean of 329. Two studies (22,24) employed datasets with less than 5,000 samples, averaging at 2,036. One study (21) utilized a sizable dataset of 34,575 samples, while only one study (23) used a dataset exceeding 100,000 samples, specifically 107,939. Only three studies (18,19,24) utilized external datasets to validate their models, highlighting a relatively limited application of external data for model validation within the referenced studies.
ML approaches
Ten out of 10 (100%) of included studies used supervised ML. Logistic regression (LR), RF, and gradient descent boosting were used in 5 studies. Four studies used artificial neural networks (ANN). Support vector machines (SVM) and classification and regression tree (CART) were used in two studies each. Naive Bayes, stacking, and linear discriminant analysis were used only in one study. Tables 2,3 provide details of ML models and their performance metrics utilized across various studies.
Table 2
Study | Type of ML model (classification) |
---|---|
Cucchetti et al. (19) | Artificial neural network |
Gibb et al. (20) | Penalized regression algorithms. In favor of a simpler model, we chose the LASSO regression |
Guo et al. (21) | Deep learning and ML algorithms, i.e., DNN, RF and LR |
Hu et al. (22) | LR, kernel SVM, and RFC |
Kanwal et al. (23) | Gradient descent boosting, LR with LASSO regularization, and LR with LASSO constrained |
Lin et al. (24) | LDA, SVM, CART, AdaBoost, Naïve Bayes, RF |
Yu et al. (12) | Decision tree, CART, random decision forest, AdaBoost |
Simsek et al. (25) | Light gradient boosting machine |
Yu et al. (26) | XGBoost, LR, gradient boost machine, RF, deep learning, stacking |
Banerjee et al. (18) | Artificial neural network: neural network software (Statistica Neural Networks, Statsoft, Tulsa, USA) |
ML, machine learning; LASSO, least absolute shrinkage and selection operator; DNN, deep neural networks; RF, random forest; LR, logistic regression; SVM, support vector machine; RFC, random forest classifiers; LDA, linear discriminant analysis; CART, classification and regression tree; AdaBoost, adaptive boosting; XGBoost, extreme gradient boosting.
Table 3
Study | AUROC | Sensitivity | Specificity | PPV | NPV | Accuracy |
---|---|---|---|---|---|---|
Cucchetti et al. (19) | ANN: internal: 0.95; external: 0.96 | 67.9% | 94.8% | 67.9% | 94.8% | 0.91 |
Gibb et al. (20) | Penalized regression: 0.961 | – | – | – | – | – |
Guo et al. (21) | ANN model: 90 days, 0.88; 180 days, 0.86; 365 days, 0.85 | – | 90 days: 0.92; 180 days: 0.88; 365 days: 0.85 | – | – | 90 days: 0.90; 180 days: 0.86; 365 days: 0.83 |
Hu et al. (22) | LR: 0.67, RFC: 0.62, RBF kernel SVM: 0.58 | – | – | – | – | 90-day mortality: LR, 0.85; RFC, 0.85; RBF kernel SVM, 0.85 |
Kanwal et al. (23) | GDB: 0.81, LR with LASSO regularization: 0.78, partial path logistic model: 0.78 | – | – | – | – | – |
Lin et al. (24) | RF: 0.852, AdaBoost: 0.833 | LDA: 0.7, SVM: 0.31, Naive Bayes: 0.29, CART: 0.37, RF: 0.37, AdaBoost: 0.45 | LDA: 0.83, SVM: 0.96, Naive Bayes: 0.92, CART: 0.37, RF: 0.37, AdaBoost: 0.45 | – | – | LDA: 0.82, SVM: 0.81, Naive Bayes: 0.78, CART: 0.79, RF: 0.82, AdaBoost: 0.81 |
Yu et al. (12) | RF: 0.838, AdaBoost: 0.792 | – | – | – | – | – |
Simsek et al. (25) | Light GBM: 30 days, 0.87; 90 days, 0.85; 365 days, 0.76 | – | – | – | – | – |
Yu et al. (26) | XGBoost: 0.888, LR: 0.673, GBM: 0.886, RF: 0.866, DNN: 0.83, stacking: 0.85 | – | – | – | – | – |
Banerjee et al. (18) | ANN: internal, 0.94; external, 0.85 | Internal: 0.90; external: 0.88 | Internal: 0.92; external: 0.91 | Internal: 0.90; external: 0.58 | Internal: 0.92; external: 0.98 | Internal: 0.91; external: 0.90 |
AUROC, area under the receiver operating characteristic curve; PPV, positive predictive value; NPV, negative predictive value; ANN, artificial neural network; LR, logistic regression; RFC, random forest classifiers; RBF, radial basis function network; SVM, state vector machines; GDB, gradient descent boosting; LASSO, least absolute shrinkage and selection operator; AdaBoost, adaptive boosting; RF, random forest; LDA, linear discriminant analysis; CART, classification and regression tree; XGBoost, extreme gradient boosting; GBM, gradient boosting machine; DNN, deep neural networks.
In the comparative analysis, 90% (9/10) of studies demonstrated superior performance of ML models over classical MELD, MELD-Na, or Child-Pugh’s models while 10% (1/10) studies showed similar performance of ML models and classical models (Figure 2). Banerjee’s ANN achieved an accuracy of 0.90 (0.82–0.98), surpassing Child-Pugh’s score of 0.55 (0.45–0.65) (18). Cucchetti’s ANN outperformed MELD with 0.96 (0.91–0.98) area under the curve (AUC) compared to 0.86 (0.79–0.91) (19). Guo’s ANN achieved an AUC of 0.88 (0.87–0.89), surpassing MELD’s 0.81 (0.80–0.82) (21). Lin’s RF model scored 0.85, exceeding MELD’s 0.76 (24). Yu’s RF model yielded 0.83, while MELD-Na scored 0.65 (12). Gibb’s LR achieved an AUC of 0.96 (0.94–0.98), outperforming MELD’s 0.934 (0.91–0.96) (20). Kanwal’s gradient boost obtained an AUC of 0.81 (0.80–0.82), significantly higher than MELD’s 0.67 (0.66–0.68) (23). Simsek’s gradient boost achieved 0.85 (0.77–0.93) AUC, while MELD-Na scored 0.73 (0.63–0.83) (25). Yu’s gradient boost model yielded 0.886, surpassing MELD-Na’s 0.782 (26). Notably, only one study by Hu showed a similar performance of LR with an average accuracy of 0.71 (0.67–0.75) compared to MELD-Na 0.72 (0.68–0.75) (22) (Figure 1).
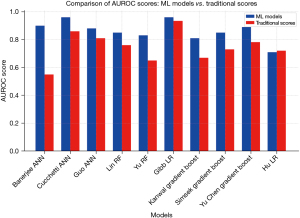
Parameters for predicting mortality
The details of various parameters utilized across various ML models are summarized in Table 4. Compared to traditional models like MELD relying on only four parameters, and MELD-Na and Child-Pugh relying on five parameters for predictions, Gibb employed 44 parameters, Guo used 41, Hu utilized 32, Simsek incorporated 23, Banerjee employed 22, Kanwal utilized 18, Yu CS included 17, Lin incorporated 15, and both Yu C and Cucchetti employed 10 parameters in training mortality prediction models.
Table 4
Study | Clinical variables used to build the predictive models |
---|---|
Cucchetti et al. (19) | Age, sex, indication for liver transplant, hepatitis B or C cirrhosis, alcoholic liver disease, primary cholestatic disease, AST, total bilirubin, GGT, ALP, creatinine, albumin, INR, platelet count, WBC, haemoglobin |
Gibb et al. (20) | Follow-up time, age, liver transplant, total bilirubin, cystatin C, creatinine, INR, sodium, WBC, IL-6, albumin, total protein, cholesterine, ALAT, ASAT, MELD score, MELD-Na score, MELD category, MELD 3.0 score, cirrhosis, ALF, CLI, ethyltoxic, HBV, HCV, AIH, PBC, PSC, NASH, cryptogenic, dialysis, GIB, HCC, SBP, mortality within 7, 30, 90 days |
Guo et al. (21) | Patient demographics, laboratory data, and information on last hospitalization including age, race, ethnicity, serum aspartate aminotransferase, alanine aminotransferase, and total bilirubin |
Hu et al. (22) | Admission variables (demographics, cirrhosis severity, history including MELD, MELD-Na, Child-Pugh score, systemic inflammatory response syndrome, medications, reasons for admission), hospital course variables (second infections, organ failures, ACLF), discharge variables (lab values, cirrhosis severity, medications) |
Kanwal et al. (23) | Age, race/ethnicity, sex, marital status, etiology of cirrhosis, MELD-Na score, cirrhosis complications (hepatic encephalopathy, ascites, varices, hepatocellular cancer), laboratory tests (sodium, creatinine, bilirubin, albumin, platelet count, hemoglobin), physical health conditions (diabetes, COPD, heart failure, cancer, chronic kidney disease), CirCom score, use of health care resources |
Lin et al. (24) | Ammonia, albumin, BUN, complete blood count, CRP, creatinine, glutamic-pyruvic transaminase, PT and INR, glutamic-oxaloacetic transaminase, serum sodium, serum potassium, total bilirubin |
Yu et al. (12) | Complete blood count, PT and INR, glutamic-oxaloacetic transaminase, serum albumin, BUN, creatinine, total bilirubin, serum sodium, serum potassium, ammonia, CRP. Patient anthropometrics and physiological characteristics including age, sex, etiology of CLD |
Simsek et al. (25) | Gender, esophageal varices, MELD-Na groups, CTP class, cirrhosis stage |
Yu et al. (26) | Demographic information: gender, age, BMI, history of hypertension, diabetes, etiology and complications of cirrhosis. Laboratory parameters: WBC, platelet count, total bilirubin, creatinine, ALT, AST, albumin, sodium, total cholesterol |
Banerjee et al. (18) | Age, sex, history of various diseases, presence of various symptoms, initial laboratory values including hemoglobin, white blood count, platelet count, blood urea nitrogen, creatinine, prothrombin time, serum bilirubin, AST, ALT, alkaline phosphatase, serum total protein, serum albumin |
AST, aspartate aminotransferase; GGT, gama glutamyl transpeptidase; ALP, alkaline phosphatase; INR, international normalized ratio; WBC, white blood count; IL-6, interleukin 6; ALT, alanine transferase; AST, aspartate transferase; MELD, model for end-stage liver disease; MELD-Na, model for end-stage liver disease-sodium; ALF, acute liver failure; CLI, chronic liver injury; HBV, hepatitis B virus; HCV, hepatitis C virus; AIH, autoimmune hepatitis; PBC, primary biliary cholangitis; PSC, primary sclerosing cholangitis; NASH, nonalcoholic steatohepatitis; GIB, gastrointestinal bleed; HCC, hepatocellular carcinoma; SBP, spontaneous bacterial peritonitis; ACLF, acute on chronic liver failure; COPD, chronic obstructive pulmonary disease; BUN, blood urea nitrogen; CRP, C-reactive protein; PT, prothrombin time; CLD, chronic liver disease; CTP, Child-Turcotte-Pugh; BMI, body mass index.
The most common parameters identified across these studies include laboratory test results and patient demographics. Laboratory tests such as complete blood count (CBC), prothrombin time (PT) and international normalized ratio (INR), serum albumin levels, creatinine, total bilirubin, and serum sodium are frequently mentioned. Patient demographics and clinical characteristics, including age, sex, etiology of cirrhosis, and complications like hepatic encephalopathy, ascites, and varices were also commonly utilized. Other parameters such as blood urea nitrogen (BUN), C-reactive protein (CRP), and liver enzymes, including glutamic-pyruvic transaminase (GPT), also known as alanine aminotransferase (ALT) and glutamic-oxaloacetic transaminase (GOT), known as aspartate aminotransferase (AST), alongside serum potassium were also included.
It is observed that similar models utilizing more parameters performed better compared to those using fewer input variables. Guo et al. (21) compared three different ML approaches with 41 vs. four parameters that are used in MELD. The deep neural networks (DNN) performance drops by 0.07 when using 41 vs. four parameters (mean AUC of 0.88 vs. 0.81). Similarly, LR and RF show a decrease in performance by 0.07 and 0.13 (mean AUC of 0.80 vs. 0.73 and 0.90 vs. 0.77) respectively.
ML risk of bias
Table 5 provides an overview of the quality assessment of the ML aspects across the included studies. Notably, code availability emerged as the least frequently reported element, with none of the studies (n=0) providing accessible code to reproduce their results. Additionally, a mere 20% (n=2) of the studies explicitly addressed the management of class imbalance and data leakage prevention. Chawla et al. employed the Synthetic Minority Over-sampling Technique (SMOTE) (27) to address imbalanced class distribution. In contrast, Hu et al. divided the data into men-only and women-only groups, alongside the general population, to examine differences in ESLD predictions across these classes. Only 40% (n=4) mentioned preprocessing the data before its utilization.
Table 5
Assessment item | No. of reporting [%] |
---|---|
Study design, population, and setting | 10 [100] |
Sample size and case proportion | 10 [100] |
Outcome assessment | 10 [100] |
Feature availability at time of prediction | 10 [100] |
Data preprocessing | 4 [40] |
Class imbalance management | 2 [20] |
Model development and feature selection | 10 [100] |
Data leakage prevention | 2 [20] |
Code availability | 0 [0] |
Discussion
Our systematic review underscores the potential of ML models in enhancing mortality prediction for patients with ESLD. With an AUROC performance range spanning from 0.71 to 0.96, our findings emphasize the necessity for standardized validation procedures and tailored management of datasets to ensure the reliability and clinical applicability of ML in healthcare settings. The observed performance differences, e.g., between models trained on small versus large datasets, highlight crucial data quality considerations, susceptibility to overfitting, and the inherent complexity of ESLD outcome prediction. The review draws attention to the need for transparent and explainable AI systems that can provide confidence and improve interpretability for clinical practitioners (28).
Predicting mortality poses a significant challenge due to the intricate interactions among biological parameters in a nonlinear fashion (29). In the past, prediction models such as the MELD, MELD-Na, and Child-Pugh scores have served as cornerstones in evaluating ESLD outcomes. However, the simplicity of these models often obscures the complicated relationship of variable interactions critical for accurate mortality prediction (30). In contrast, ML models offer a distinct advantage by leveraging multiple parameters, enabling them to uncover complex nonlinear relationships and enhance mortality prediction accuracy (31). This review aligns with prior literature, affirming that ML models, with their ability to accommodate and comprehend intricate nonlinear relationships of input variables, potentially enable greater predictive accuracy (32).
However, the use of ML in clinical practice is not without its problems. The reliance on small data sets because of the limited availability of data hinders the generalization of the models in our study population. In addition, the lack of transparency and interpretability in ML approaches is a significant obstacle to clinical application (33). ML techniques such as ANN require ample data and are susceptible to overfitting on the training dataset. Studies that utilize small sample sizes, particularly fewer than 100 samples, without adequate external validation run the risk of overfitting (34). Only a minority of studies in the survey (4/10) used ANN, which remains underutilized in ESLD mortality prediction despite their potential for superior performance on big data. Furthermore, more than half the studies (7/10) did not use any external validation. A standardized external dataset with a diverse and balanced population could prove beneficial for the generalizability and improve our understanding of patient mortality risk of such ML models (35).
The overarching “black box” nature of ML models calls for a shift towards more interpretable and explainable ML models for wider acceptance among clinicians (36). One study [Yu et al. (26)] tried using visualizations to better explain the significant variables the underlying ML models used. Similarly, Kanwal et al. (23) chose a simpler ML model with less accuracy but higher transparency over other more complicated but opaque ML models. This sentiment is echoed in the broader literature (37), where there is a growing call for simpler, more interpretable models for better explainability (37). Additionally, the inclusion of socioeconomic and psychosocial variables in ML models could yield a richer, more holistic view of patient outcomes. This approach not only aligns with the emerging emphasis on integrating broader determinants of health into predictive models but also addresses the limitations inherent in models that focus predominantly on clinical and laboratory data (38,39).
Our systematic review highlights the potential of ML models to enhance ESLD mortality prediction. However, to fully harness this potential in clinical practice, it is crucial to address challenges related to data integrity and model transparency. The role of missing data in these studies remains unclear, which is a critical issue. Missing data can lead to systematic bias, particularly if certain subgroups of patients are underrepresented in the healthcare system. In our review, while all studies (10/10) accounted for base characteristics including gender, ethnicity, and age, only a minority (3/10) (17,18,20) addressed and specified missing data as part of their research. This oversight can contribute to biases in ML algorithms, which may arise from factors such as sample size, misclassification, and measurement error (39). To transition effectively from research to practical clinical application, research should focus on developing robust, interpretable models validated across diverse datasets and incorporating a comprehensive set of predictive variables. Transparent models that provide clear, actionable insights are essential for gaining the trust of clinicians and ensuring reliable deployment across varied clinical environments. The adoption of ML tools by leading institutions underscores the growing recognition of their potential in enhancing clinical decision-making (40). We urge future researchers to share their code and data transparently, facilitating peer reviews to identify any instances of overfitting. Additionally, pragmatic trials that test interventions in routine clinical settings are essential for gathering insights into the real-world efficacy of these models. By overcoming these challenges, ML models can significantly improve the prognostic tools available in ESLD, providing clinicians with sophisticated resources to enhance patient care and outcomes, marking a significant advancement from traditional, simplistic models to dynamic, data-driven methodologies.
The role of missing data in these studies remains unclear, which is a critical issue. Missing data can lead to systematic bias, particularly if certain subgroups of patients are underrepresented in the healthcare system.
Conclusions
In conclusion, our systematic review highlights the potential of ML models to improve ESLD mortality prediction. However, to enable an effective transition from research to bedside, future research should focus on developing robust, interpretable models validated against multiple data sets and including a comprehensive set of predictive variables. In addition, sharing of codes and data transparency will facilitate peer reviews to identify any instances of overfitting. By addressing these challenges, ML models can greatly enhance the tools used to predict outcomes in ESLD, marking a major shift from traditional, simple models to more dynamic, data-driven approaches.
Acknowledgments
Funding: This work was supported in part by
Footnote
Reporting Checklist: The authors have completed the PRISMA and CHARMS reporting checklists. Available at https://jmai.amegroups.com/article/view/10.21037/jmai-24-205/rc
Peer Review File: Available at https://jmai.amegroups.com/article/view/10.21037/jmai-24-205/prf
Conflicts of Interest: All authors have completed the ICMJE uniform disclosure form (available at https://jmai.amegroups.com/article/view/10.21037/jmai-24-205/coif). L.J.F. received Veterans Affairs Health Systems Research Merit Grant (No. 1I01HX003379). The other authors have no conflicts of interest to declare.
Ethical Statement: The authors are accountable for all aspects of the work in ensuring that questions related to the accuracy or integrity of any part of the work are appropriately investigated and resolved.
Open Access Statement: This is an Open Access article distributed in accordance with the Creative Commons Attribution-NonCommercial-NoDerivs 4.0 International License (CC BY-NC-ND 4.0), which permits the non-commercial replication and distribution of the article with the strict proviso that no changes or edits are made and the original work is properly cited (including links to both the formal publication through the relevant DOI and the license). See: https://creativecommons.org/licenses/by-nc-nd/4.0/.
References
- Lindor KD, Gershwin ME, Poupon R, et al. Primary biliary cirrhosis. Hepatology 2009;50:291-308. [Crossref] [PubMed]
- Cheemerla S, Balakrishnan M. Global Epidemiology of Chronic Liver Disease. Clin Liver Dis (Hoboken) 2021;17:365-70. [Crossref] [PubMed]
- da Silveira F, Soares PHR, Marchesan LQ, et al. Assessing the prognosis of cirrhotic patients in the intensive care unit: What we know and what we need to know better. World J Hepatol 2021;13:1341-50. [Crossref] [PubMed]
- Pugh RN, Murray-Lyon IM, Dawson JL, et al. Transection of the oesophagus for bleeding oesophageal varices. Br J Surg 1973;60:646-9. [Crossref] [PubMed]
- Wiesner R, Edwards E, Freeman R, et al. Model for end-stage liver disease (MELD) and allocation of donor livers. Gastroenterology 2003;124:91-6. [Crossref] [PubMed]
- Kamath PS, Kim WRAdvanced Liver Disease Study Group. The model for end-stage liver disease (MELD). Hepatology 2007;45:797-805. [Crossref] [PubMed]
- Said A, Williams J, Holden J, et al. Model for end stage liver disease score predicts mortality across a broad spectrum of liver disease. J Hepatol 2004;40:897-903. [Crossref] [PubMed]
- Rojas E, Munoz-Gama J, Sepúlveda M, et al. Process mining in healthcare: A literature review. J Biomed Inform 2016;61:224-36. [Crossref] [PubMed]
- Chongo G, Soldera J. Use of machine learning models for the prognostication of liver transplantation: A systematic review. World J Transplant 2024;14:88891. [Crossref] [PubMed]
- Soldera J, Corso LL, Rech MM, et al. Predicting major adverse cardiovascular events after orthotopic liver transplantation using a supervised machine learning model: A cohort study. World J Hepatol 2024;16:193-210. [Crossref] [PubMed]
- Soldera J, Tomé F, Corso LL, et al. Use of a Machine Learning Algorithm to Predict Rebleeding and Mortality for Oesophageal Variceal Bleeding in Cirrhotic Patients. EMJ Gastroenterol 2020;9:46-8.
- Yu CS, Chen YD, Chang SS, et al. Exploring and predicting mortality among patients with end-stage liver disease without cancer: a machine learning approach. Eur J Gastroenterol Hepatol 2021;33:1117-23. [Crossref] [PubMed]
- Moons KG, de Groot JA, Bouwmeester W, et al. Critical appraisal and data extraction for systematic reviews of prediction modelling studies: the CHARMS checklist. PLoS Med 2014;11:e1001744. [Crossref] [PubMed]
- Page MJ, McKenzie JE, Bossuyt PM, et al. The PRISMA 2020 statement: an updated guideline for reporting systematic reviews. BMJ 2021;372:n71. [Crossref] [PubMed]
- Moher D, Liberati A, Tetzlaff J, et al. Preferred reporting items for systematic reviews and meta-analyses: the PRISMA statement. Int J Surg 2010;8:336-41. Erratum in: Int J Surg 2010;8:658. [Crossref] [PubMed]
- Stevens LM, Mortazavi BJ, Deo RC, et al. Recommendations for Reporting Machine Learning Analyses in Clinical Research. Circ Cardiovasc Qual Outcomes 2020;13:e006556. [Crossref] [PubMed]
- Luo W, Phung D, Tran T, et al. Guidelines for Developing and Reporting Machine Learning Predictive Models in Biomedical Research: A Multidisciplinary View. J Med Internet Res 2016;18:e323. [Crossref] [PubMed]
- Banerjee R, Das A, Ghoshal UC, et al. Predicting mortality in patients with cirrhosis of liver with application of neural network technology. J Gastroenterol Hepatol 2003;18:1054-60. [Crossref] [PubMed]
- Cucchetti A, Vivarelli M, Heaton ND, et al. Artificial neural network is superior to MELD in predicting mortality of patients with end-stage liver disease. Gut 2007;56:253-8. [Crossref] [PubMed]
- Gibb S, Berg T, Herber A, et al. A new machine-learning-based prediction of survival in patients with end-stage liver disease. Journal of Laboratory Medicine 2023;47:13-21. [Crossref]
- Guo A, Mazumder NR, Ladner DP, et al. Predicting mortality among patients with liver cirrhosis in electronic health records with machine learning. PLoS One 2021;16:e0256428. [Crossref] [PubMed]
- Hu C, Anjur V, Saboo K, et al. Low Predictability of Readmissions and Death Using Machine Learning in Cirrhosis. Am J Gastroenterol 2021;116:336-46. [Crossref] [PubMed]
- Kanwal F, Taylor TJ, Kramer JR, et al. Development, Validation, and Evaluation of a Simple Machine Learning Model to Predict Cirrhosis Mortality. JAMA Netw Open 2020;3:e2023780. [Crossref] [PubMed]
- Lin YJ, Chen RJ, Tang JH, et al. Machine-Learning Monitoring System for Predicting Mortality Among Patients With Noncancer End-Stage Liver Disease: Retrospective Study. JMIR Med Inform 2020;8:e24305. [Crossref] [PubMed]
- Simsek C, Sahin H, Emir Tekin I, et al. Artificial intelligence to predict overall survivals of patients with cirrhosis and outcomes of variceal bleeding. Hepatol Forum 2021;2:55-9. [Crossref] [PubMed]
- Yu C, Li Y, Yin M, et al. Automated Machine Learning in Predicting 30-Day Mortality in Patients with Non-Cholestatic Cirrhosis. J Pers Med 2022;12:1930. [Crossref] [PubMed]
- Chawla NV, Bowyer KW, Hall LO, et al. SMOTE: Synthetic Minority Over-sampling Technique. Journal of Artificial Intelligence Research 2002;16:321-57. [Crossref]
- Markus AF, Kors JA, Rijnbeek PR. The role of explainability in creating trustworthy artificial intelligence for health care: A comprehensive survey of the terminology, design choices, and evaluation strategies. J Biomed Inform 2021;113:103655. [Crossref] [PubMed]
- Villaverde AF, Banga JR. Reverse engineering and identification in systems biology: strategies, perspectives and challenges. J R Soc Interface 2014;11:20130505. [Crossref] [PubMed]
- Peng Y, Qi X, Guo X. Child-Pugh Versus MELD Score for the Assessment of Prognosis in Liver Cirrhosis: A Systematic Review and Meta-Analysis of Observational Studies. Medicine (Baltimore) 2016;95:e2877. [Crossref] [PubMed]
- Kline A, Wang H, Li Y, et al. Multimodal machine learning in precision health: A scoping review. NPJ Digit Med 2022;5:171. [Crossref] [PubMed]
- Rajula HSR, Verlato G, Manchia M, et al. Comparison of Conventional Statistical Methods with Machine Learning in Medicine: Diagnosis, Drug Development, and Treatment. Medicina (Kaunas) 2020;56:455. [Crossref] [PubMed]
- Plass M, Kargl M, Kiehl TR, et al. Explainability and causability in digital pathology. J Pathol Clin Res 2023;9:251-60. [Crossref] [PubMed]
- Charilaou P, Battat R. Machine learning models and over-fitting considerations. World J Gastroenterol 2022;28:605-7. [Crossref] [PubMed]
- Dexter GP, Grannis SJ, Dixon BE, et al. Generalization of Machine Learning Approaches to Identify Notifiable Conditions from a Statewide Health Information Exchange. AMIA Jt Summits Transl Sci Proc 2020;2020:152-61. [PubMed]
- Rudin C. Stop Explaining Black Box Machine Learning Models for High Stakes Decisions and Use Interpretable Models Instead. Nat Mach Intell 2019;1:206-15. [Crossref] [PubMed]
- Linardatos P, Papastefanopoulos V, Kotsiantis S, Explainable AI. A Review of Machine Learning Interpretability Methods. Entropy (Basel) 2020;23:18. [Crossref] [PubMed]
- Adler-Milstein J, Aggarwal N, Ahmed M, et al. Meeting the Moment: Addressing Barriers and Facilitating Clinical Adoption of Artificial Intelligence in Medical Diagnosis. NAM Perspect 2022;2022: [Crossref] [PubMed]
- Gianfrancesco MA, Tamang S, Yazdany J, et al. Potential Biases in Machine Learning Algorithms Using Electronic Health Record Data. JAMA Intern Med 2018;178:1544-7. [Crossref] [PubMed]
- Khosravi M, Zare Z, Mojtabaeian SM, et al. Artificial Intelligence and Decision-Making in Healthcare: A Thematic Analysis of a Systematic Review of Reviews. Health Serv Res Manag Epidemiol 2024;11:23333928241234863. [Crossref] [PubMed]
Cite this article as: Malik S, Frey LJ, Qureshi K. Evaluating the predictive power of machine learning in cirrhosis mortality: a systematic review. J Med Artif Intell 2025;8:15.